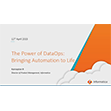
Cataloging Alation’s Growth Potential
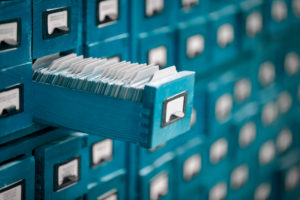
(Andrey_Kuzmin/Shutterstock)
Super-fast processors and snappy visualizations may elicit “oohs” and “ahhs” from the big data masses. But here’s the thing: the best analytics setup isn’t worth a dime if you can’t find the right data to analyze in the first place. That was the discovery that Alation made when it created the data catalog product category two years ago, and it continues to be the company’s focus going forward.
“When we launched the company back in 2015, nobody knew what a data catalog was,” says Alation co-founder and CEO Satyen Sangani. “Many people didn’t care or thought it was a feature. We’ve proven very clearly that’s not the case.”
Armed with its industry-first data catalog software – which not only tracks the data sets available within an organization but also tracks how people use that data — the company has racked up nearly 100 customer wins over the past two years, including blue-chip names like GE, Pfizer, LinkedIn, EBay, and Tesco. It’s also drawn praise from analyst groups like Gartner, Forrester, and Dresner Advisory Services.
Instead of being a feature within a broader data integration toolset, Alation has shown that data catalogs can be standalone products that play a role in many different projects, including data governance initiatives, Hadoop and S3 metadata management and optimization projects, and GDPR remediation initiatives. (Datanami also added a data catalog category for its 2017 Reader’s Choice Awards; nominations are now being taken.)
Today the Redwood City, California company announced that it has raised $23 million in a Series B round led by Icon Ventures, bringing its total to-date funding to about $31 million. The plan calls for investing in sales and marketing to strengthen its go-to-market strategy, and doubling the size of its engineering staff to accommodate continued development of its product.
No Single Source of Truth
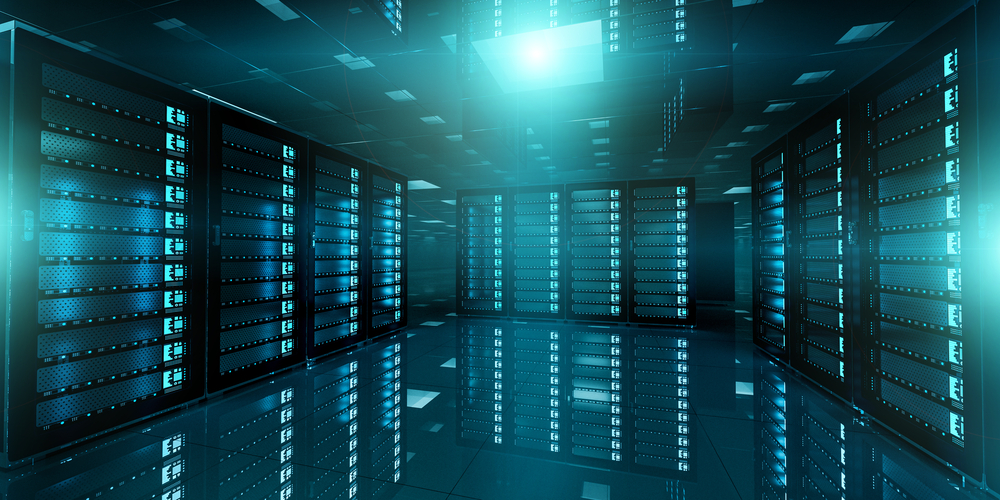
The truth is not in the data warehouse (sdecoret/Shutterstock)
Sangani is obviously bullish on his company’s prospects. But that confidence does not stem from blind faith in his ability to execute a plan. Rather, it seems to come from some hard-won lessons about the state of big data management, and how best to equip teams to succeed going forward.
In Sangani’s view, organizations will struggle if they cling to old views about data, including how to store it, how to track it, and ultimately what it can tell you about the truth.
“The old data warehouse was all about a single source of truth, and at some level creating a data lake is about creating a single source of truth, which is ‘Let’s go physically land all the data in a single place,'” he tells Datanami. “That doesn’t meet with any form of reality that I’ve ever been associated with.”
The reality, he says, is data is spread across a thousand different systems and accessed using hundreds of different tools. “And there’s going to be lots of code knitting all this stuff together,” he says. “That is the state of enterprise IT and that will be the state of enterprise IT because there’s just a lot more software and data being built than there are people to put it all in one place.
“I think the single source of truth is a myth,” he continues.
Shades of Gray
Instead of spending millions trying to hammer data into a single-source-of-truth format, Sangani prefers the more organic approach that positions a data catalog as the single point of reference – one that will hopefully yield insights that take his customers closer to the truth. It’s a quest that starts with a strong philosophical viewpoint, is hardened by reality, and yields to the pragmatic lessons of what works in big data initiatives, and what doesn’t.
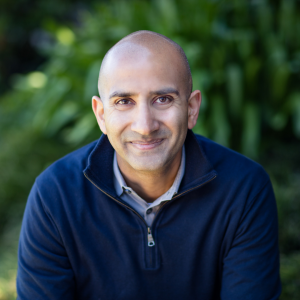
Alation CEO Satyen Sangani
“You have this fictional notion that truth is singular and it’s not going to ever change. The entire notion of analytics is you’re constantly learning,” Sangani says. “I think it’s an absolute recognition – a pragmatic and realistic recognition — that the single source of truth is a myth. There’s sales’ truth and finance’s truth, which is different than marketing’s truth. And those things will converge over time, but it’s not a clean process. It’s a messy process. And it’s not a black-and-white process. It’s a shade-of-grey process.”
Alation intends to go on enabling teams of data scientists, analysts, engineers, executives, and managers to continue their messy quest to find data that can help them answer their questions, and get closer to that state of truth. Sometimes that means finding new sources of data that have not been acted upon. Other times it means picking up an old data project that somebody started but abandoned along the way. It’s as much about enabling the analytics journey as actually getting the right answer.
Managing the Process
As powerful as today’s analytics technology have become, they still can’t eliminate some of the most basic data management challenges that organizations face when they attempt to harness big data. It’s why data scientists still spend most of their time collecting data and getting it ready to analyze. The analysis portion is actually a small part of the overall big data analytics equation.
Alation’s goal is to help organizations solve one part of this problem, which is pointing them to the right data and enabling employees to collaborate more effectively around their data. “The catalog notion…gets to the point of ‘I don’t need to know everything. I just need to know where to go when I have a question,'” Sangani says.
“Managing data is really about managing not just the data itself, but the technology around the data and the knowledge about how to use this stuff appropriately,” the CEO continues. “And that problem is a problem that every single person that has a knowledge worker job in the enterprise is going to need to deal with. Whether you’re a data steward or data scientist or a product manager or executive, you’re going to need a place to go and find and use the right data. And that’s not going to be some random arbitrary data warehouse in the company. It’s going to be a data catalog and that’s what we’re building.”
Related Items:
Data Catalogs Emerge as Strategic Requirement for Data Lakes
Battling Big Data’s Tribal Knowledge Problem