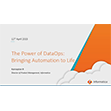
Cloudera Unveils Altus to Simplify Hadoop in the Cloud
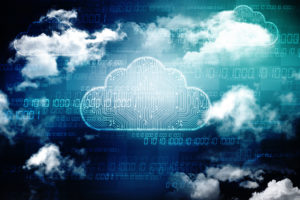
(Blackboard/Shutterstock)
Running Hadoop, whether on-premise or in the cloud, is neither simple nor easy. Administrators with specialized skills are needed to configure, manage, and maintain the clusters for their clients, who are data scientists, engineers, and analysts. Now Cloudera is looking to eliminate that burden with a new cloud-based offering dubbed Altus.
Altus, which the Hadoop distributor unveiled today at its Strata Data Conference in London, is a new platform designed to make it easier for users to access and run its Hadoop suite of tools and applications on public cloud infrastructure. The first Altus offering runs on AWS, consumes data from the S3 object store, and targets data engineering workloads, but other Cloudera products and public cloud platforms (and object stores) will be supported over time.
It’s all about making Hadoop easy, says David Tishgart, who heads up product marketing for Cloudera’s Data Engineering, the first Cloudera product to be exposed to the public atop the Altus platform.
“We want to make sure the data engineers, who are more frequently working on transient clusters in cloud, are able to quickly spin up jobs, run their jobs, and terminate them, but do so in a way that doesn’t require them to also deal with cluster operations and management,” Tishgart tells Datanami.
Cloudera is no cloud newbie – 18% of the workloads under Cloudera’s Distribution of Hadoop (CDH) already run on cloud infrastructure, Tishgart says. The difference is that organizations would have to provide their own personnel to handle cluster and cloud administrative tasks with standard cloud CDH. That requirement is eliminated with the Altus platform as a service (PaaS) offering.
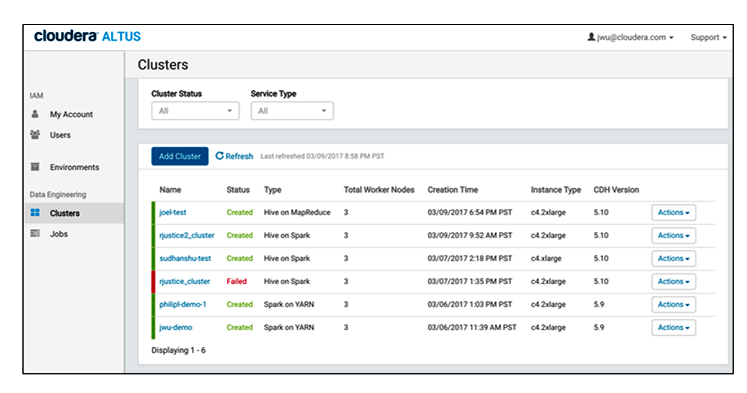
Altus provides a GUI dashboard for data engineers to work with Spark, Hive, and MapReduce2 jobs that execute in the cloud
“Before Altus, when you wanted to run your data processing jobs on cloud environments, you also had to deal with the infrastructure overhead, the management and operations of your cluster,” Tishgart says. “What Altus does is it remove that burden completely.”
Cloudera sells its Hadoop-based distribution through various SKUs (or stock keeping units). The Data Engineering SKU is focused on data ingest and transformation tasks, and includes Spark, Hive, Hive on Spark, and MapReduce2 engines.
Up to this point, Cloudera customers could license the Data Engineering SKU, deploy it on AWS or Azure public clouds , and then manage those clusters with Cloudera Director. Now that Data Engineering is a PaaS offering on AWS, clients using no longer have to worry about using Cloudera Director (although Cloudera points out that they will still need Director for other cloud uses).
Data engineers, who are the targeted customers with this product, interact with Altus primarily through a portal. Cloudera provides a GUI and a command line environment where they can submit their Spark, Hive, and MR2 jobs. The Altus PaaS handles all the nitty gritty details of obtaining EC2 computing resources from AWS, configuring the cluster, spinning it up, and then closing it out.
“Altus represents a large step toward making this much more usable to the end user,” says Jennifer Wu, a product manager for Data Engineering. “The ease-of-use element here is a big step forward because we are now managing the cluster, whereas before there are full-time people actually doing administration of these clusters.”
There is a one-time setup that does require an administrator to assist with, which mostly involves mapping the workload to the actual compute resources that will be needed to process the jobs. After that, it’s a simple matter for data engineers to access the portal, submit a job, run some data pipelines, and get the results.
Cloudera says it takes about 30 seconds for a data engineer to configure an Altus cluster on AWS, while the spin-up of the cluster takes minutes. “The configuration of the cluster is very easy, the spin-up of the cluster is very easy, and you just get a portal to actually do this for workloads,” Wu says. “What’s left is basically submitting jobs, troubleshooting jobs, and rerunning your jobs.”
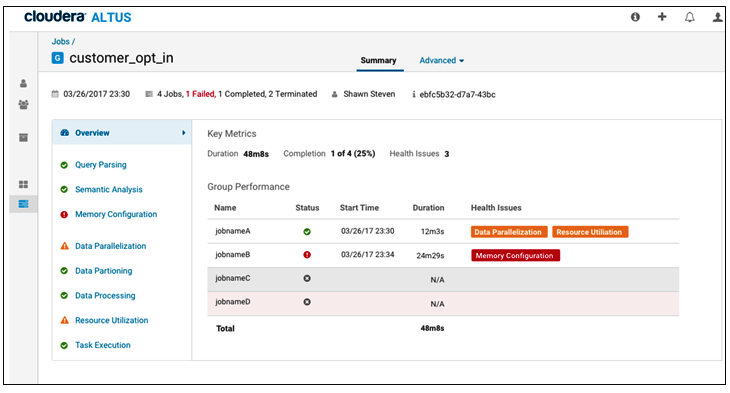
More advanced troubleshooting capabilities in Altus are slated for release in June
Cloudera has also build some troubleshooting capabilities into Altus Data Engineering. The dashboard includes a job history component where data engineers can see which jobs completed and which ones failed. It also allows them clone jobs and terminate them as well.
In June, Cloudera plans to add a workload analytics capability to the offering. “It will give you a whole bunch of root cause analysis about why jobs aren’t running as performant as before,” Wu says. “If a user ran 50 pipelines one day and four of them failed, then they would go and troubleshoot those jobs.”
Cloudera is not supporting dynamic workload scaling with the Data Engineering offering on Altus. Data Engineering workloads are typically batch oriented and have well-defined boundaries in terms of the resources they require and their service level agreement (SLA), Wu says, so there is not as great of a need to dynamically scale the size of Altus clusters up and down.
“You typically know the size of the cluster and the instance type you need,” Wu says. “You know that this 1TB is coming in and you know which instance type and cluster type you need in order to meet your SLA of cleaning this within four hours, for example.”
Where dynamic scaling becomes more useful is with interactive applications where the number of users can’t be predicted, she says. That could potentially include Impala and HBase, which are also on the roadmap for inclusion into the Altus PaaS via the Analytic DB product and the Operational DB product, respectively.
Cloudera’s roadmap calls for Altus becoming available on Microsoft Azure cloud nex. “It’s our goal ultimately to make this platform more accessible to cloud users,” Tishgart says. “So we’re going to start to see additional cloud components and applications rolled out on Altus over time. We wanted to lead with the Data Engineering applications.”
Cloudera’s eventual goal is to allow users to run Hadoop workloads in any manner they choose — including on-premise, public cloud, and hybrid deployments – while maintaining continuity among the data, the applications, and the users. Partners are also involved with this story, and data integration software vendor Talend today announced that it’s supporting Altus.
“We want to make sure that, as we’re evolving the platform, that all of our components are interoperable with each other,” Wu says. “We want all the metadata to be shared, the security management to be shared, the Navigator lineage [to be shared] and audit information to be secure. We want basically the whole platform to still work together, regardless of what deployment model you use.”
Pricing for Altus is based on the number of nodes and the number of hours used by a customer.
Related Items:
Why Analytics Are Now Assumed to Run in the Cloud
Cloudera IPO Lauded by Big Data Community
Cloudera Gives Data Scientists a New Workbench