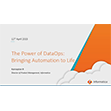
Cognitive Push: Progress Buys DataRPM
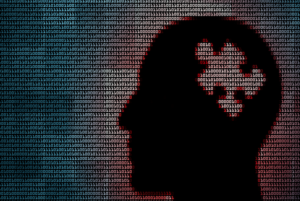
(enzozo/Shutterstock)
The acquisition of a cognitive-based predictive maintenance specialist by Progress, the application developer, may signal the beginning of a long-awaited big data consolidation, perhaps driven by emerging deployments such as the industrial Internet of Things.
The late March acquisition of DataRPM, a privately held machine learning company by Progress (NASDAQ: PRGS) is part of what the software vendor said was it’s a key component of its platform strategy for delivering “cognitive-first” applications.
Progress acquired DataRPM for $30 million, including $28.3 million paid in cash, and the balance payable to DataRPM’s founders in the form of restricted Progress stock.
Progress, Bedford, Mass., said the acquisition of DataRPM gives it a predictive analytics capability it can use to round its emerging cognitive apps platform. DataRPM’s cognitive capability would be integrated with current front-end application development tooling along with back-end application services and data connectivity, the company said.
Founded in 2012, DataRPM of Redwood City, Calif., offers a cognitive data science platform for developing predictive analytics. The patented platform uses “meta” machine learning and artificial intelligence to automate predictive maintenance used in industrial IoT deployments. The platform leverages meta-learning capabilities to improve predictions about equipment failures. Industrial customers for the prediction platform include automaker Jaguar, Mitsubishi Heavy Industries (TYO: 7011) and South Korean electronics giant Samsung (KRX: 005930).
The combination of the Progress application platform with DataRPM’s cognitive predictive maintenance tool would hasten development of “cognitive applications across multiple markets,” Sundeep Sanghavi, co-founder and CEO of DataRPM, noted in a statement.
The predictive maintenance technology is currently used to detect random and unknown failures using unsupervised and partially supervised machine learning techniques. Unsupervised learning involves presenting a machine presented with unlabeled data. It is instructed to discover underlying patterns such as a clustering structure.
In the case of semi-supervised learning, unlabeled examples are used along with a small amount of labeled data to improve learning accuracy.
DataRPM also touts its approach as addressing the data scientist shortfall by teaching machines to automate analytics by via “meta learning,” or learning from experience and feedback. The resulting automation would eliminate the need for hiring sought-after data scientists.
The startup’s approach also can be scaled across industrial operations to monitor and track a range of machines.
“The combination of application development, data connectivity, and business rules management software with machine learning and predictive analytics makes sense in terms of providing a platform that will enable [independent software vendors] and enterprises to develop operational applications that take advantage of the intelligence being generated by big data and IoT projects,” noted Matt Aslett, research director for data platforms and analytics at 451 Research.
Recent items:
DataRPM Gets $5M to Liberate Data From Warehouses
Why Big Data Isn’t Changing Everything (At Least Not Yet)