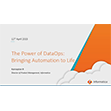
Biomedical Text Mining Tool Gets the Lead Out
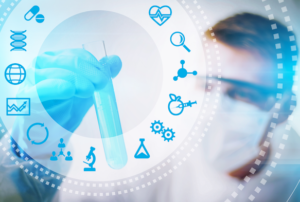
via Shutterstock
Approximately 100 lines of Python code serve as the basis of a new predictive text-mining tool designed to accelerate the scanning online biomedical research papers for clues on everything from repurposing existing drugs to advancing stem cell treatment.
Coders from the Morgridge Institute for Research working in partnership with the University of Wisconsin at Madison reported on their “KinderMiner” algorithm during a bioinformatics conference in San Francisco this week. The researchers said the 100-line algorithm was “within hours” able to scan more than 30 million online papers to provide ranked and relevant associations based on key words and phrases.
“Most often, researchers are running manual Google searches and combing through millions of hits to find, for example, certain genes that are important to a biological process or disease,” explained Ron Stewart, associate director of bioinformatics at the Morgridge Institute. “It’s often based on hunches and intuition. We’re trying to automate and formalize that process.”
Alternative techniques require much data wrangling, added Finn Kuusisto, a postdoctoral researcher at the Morgridge Institute. “We write about 100 lines of Python code, and our users can be given answers that may significantly speed up their scientific process.”
There is no shortage of online biomedical research to sift, and the researchers noted that their algorithm could be used for any scientific discipline generating lots of online research papers. Missing are better and faster text-mining tools. The researchers said their next step is creating an online search tool that can be widely used by the biomedical community.
An early application is finding new applications for drugs already approved the U.S. Food and Drug Administration. “Repurposed” drugs account for about 30 percent of all new drugs and vaccines approved by the FDA.
“You could spend all your time—and all your students’ time—scanning the literature for this kind of secondary drug effect and only scratch the surface of what’s out there,” explained study co-author David Page, a professor of biostatistics and medical informatics at the University of Wisconsin. “It’s better to write an automated machine learning package to do it instead.”
Along with literature searches related to potential drugs with off-label benefits or adverse effects, the researchers tested their algorithm on another time-consuming process: identifying relevant factors used to “reprogram” stem cells. The search of about 2,000 known stem cell transcription factors—the process used to change a cell from one state to another—was purposely narrowed to boost the predictive power of the KinderMiner algorithm.
The researchers reported that three test searches identified stem cell factors in the top 20 hits, and KinderMiner ranks those factors so the most relevant show up among the top 10 to 20.
By comparison, traditional methods requiring stem cell researchers to test ten factors in groups of four. That method would require more than 200 “manageable” experiments. Hence, the data-mining tool represents a kind of “time machine for biology, where we can go back before any of the big publications came out on reprogramming, and still make a good guess about what genes are most important,” Stewart noted.
Meanwhile, the researchers have gained approval to use the electronic health records of about 10 million military veterans with patient names’ removed to search for drug effects such as lower blood pressure or cholesterol levels.
Recent items:
Text Analytics and Machine Learning: A Virtuous Combination