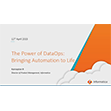
3 Key Steps to Transforming Healthcare with Predictive Analytics
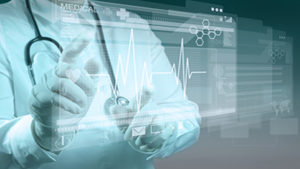
The healthcare industry has a data problem. There is an overabundance of raw data available, and figuring out the best way to manage this data has presented many challenges. For example, the data from patient records from heart-rate monitors is quickly referenced and then forgotten. The good news is, all of this raw data can actually be used to solve many day-to-day problems in the healthcare industry using predictive analytics.
Predictive analytics is the practice of extracting information from existing data to determine patterns as well as predict future outcomes or trends. Predictive analytics has a bright future in the healthcare industry because of the numerous factors already in place that are working together: the enormous amount of data, the important need to understand this raw data and its meaning, and a significant number of business-oriented applications to which data analysis can be applied.
We are only at the beginning of using data analysis effectively in healthcare; however, predictive analytics can be used to solve many of the real-world problems the healthcare industry faces today. To successfully implement a predictive analytics solution in the healthcare industry, it’s crucial to have a clear vision of the desired outcomes, to have IT systems in place that are interoperable, and also, the organizations must have a strong commitment to knowledge-sharing across departments. If a healthcare organization can meet these demands, then here are some of the key steps for setting up predictive analytics for your organization.
1. Identify Organizational Requisites
Predictive analytics are designed to make a real impact on existing organization processes and to improve efficiency. In the healthcare industry, the data already exists in abundance, so the challenge most organizations face is determining what to do with all of the data and how to make sense of it. There is simply too much data in nearly every aspect of healthcare, but where that data exists, there is also potential to implement a predictive analytics system.
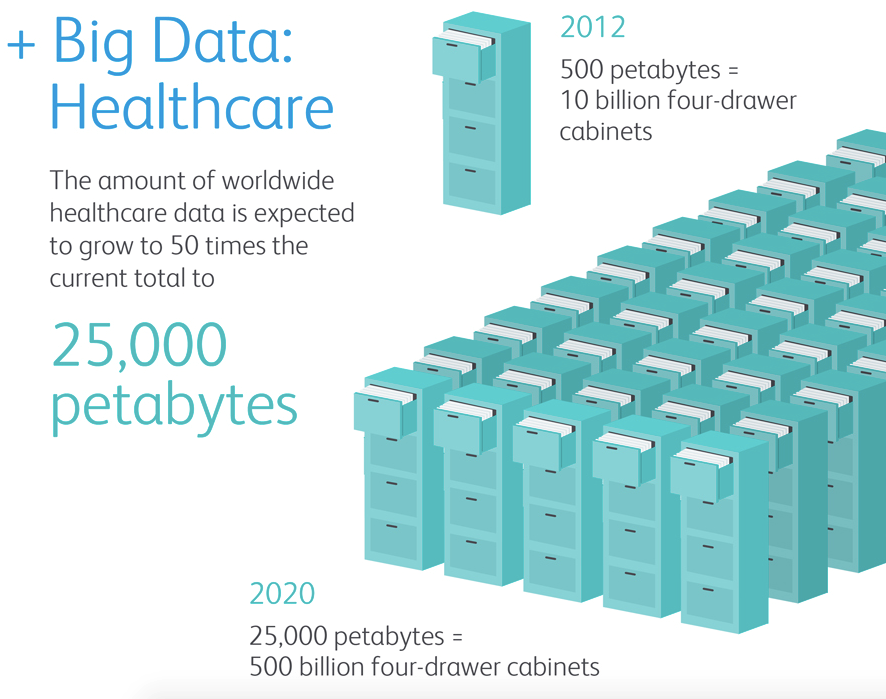
Source: The Healthcare Blog
A predictive analytics platform can provide a strong pathway to answering the question of what to do with this data, and predictive technology has a wide range of applications in healthcare. For example, it can be applied to disease management, physician profiling, or the handling of no-shows and appointment scheduling.
Tackling these types of issues requires a comprehensive analytics platform that can not only automate and visualize data but also be used as a collaborative tool. Furthermore, it is equally necessary that an analytics platform proactively informs organizations for specific reasons to help with decision-making.
2. Leverage the Available Data
After a healthcare organization has identified its analytical needs, the next step is to collect and cleanse the data. It’s important to define from where exactly the data is coming in order to cleanse it. This process includes a variety of tasks such as dealing with missing data, redundant data, and unformatted data. The most important thing is that the dataset is standardized so that the process can be automated.
Take a look at the case of the Veteran’s Health Administration (VHA), the largest health system in the United States, which has collected electronic data from its patients for over three decades. In 2006, the VHA constructed a data warehouse as a storage place for all of its patient-level data across the nation. The sheer amount of data available allowed the VHA to develop unique algorithms that reliably predict meaningful outcomes, such as the risk of hospitalization or the risk of death. Workers use the outputted information to guide and improve the quality of their care. This allowed the VHA to receive a $3 billion net return on its data investment.
3. Focus on the End User
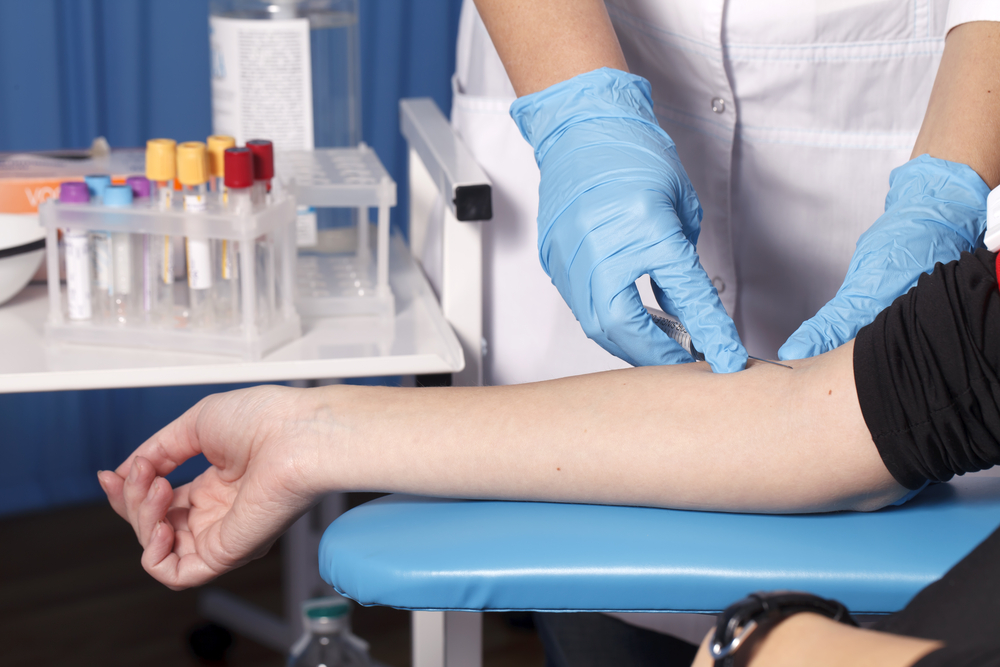
(HOMONSTOCK/Shutterstock)
Having a predictive analytics platform in place is useless if the end results cannot effectively be deployed to the appropriate organizational process or system. In the healthcare industry, analytical findings must be made available to those working in day-to-day operations, such as nurses, physicians, and insurance analysts.
Again, this is why predictive analytics platforms must be easy-to-use and accessible across an organization. The outputs are not just for data scientists but also for anyone that needs the information — including those without an IT background. Healthcare professionals deal with large amounts of data every day, so the results that come from an organization’s predictive analytics platform must be integrated smoothly into their existing daily workflows to be truly beneficial.
Predictive Analytics Is Rapidly Changing Healthcare
With the introduction of the Health Information Technology for Economic and Clinical Health (HITECH) Act of 2009, incentives for higher billing and reimbursement rates from the federal government continue to drive adoption rates of electronic health records (EHR). EMR usage has been steadily increasing over the last 15 years. From 2001 to 2014, EMR usage in physician offices rose from 20% to over 82%. What if electronic health record (EHR) data could be used to predict patient non-compliance with medicine? What if Internet of Things (IoT) sensor data in hospitals could be used to predict medical supply shortages. The possibilities for predictive analytics in healthcare are endless when this vast amount of data can be collected, cleansed, formatted, and transformed in a meaningful way.
The healthcare industry is faced with enormous challenges whose solutions heavily revolve around large amounts of untapped data. Most organizations simply have too much data to handle. However, analytics platforms have the capability to transform this raw data into powerful insights for life-saving decision making.
About the author: Pauline Brown is the vice president of marketing at Dataiku, a developer of data science software. Follow her on Twitter at @dataiku or on LinkedIn.
Related Items:
Can Big Data Deliver on the Huge Expectations of Precision Medicine?
Health Care Emerges as Hadoop Use Case
Analytics Big Role in New Healthcare Model