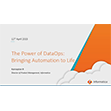
Machine Learning and Airport Security See Eye to Eye
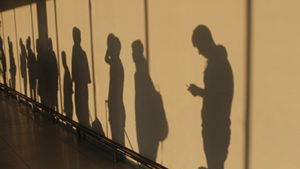
(Gabriel Petrescu/Shutterstock)
Who hasn’t groaned at the sight of a long airport security line? The prospect of standing for hours on end has become all too common at airports around the world. But soon, airports may be piloting security programs based on behavior recognition and machine learning, instead of asking passengers to practice patience.
As we know, patience is becoming a lost art, but predictive analytics based on sensor, device, and video data is a technology art form that airlines and airports are exploring.
The 9/11 attacks and the 2001 Shoe Bomber’s attempt are among the most well-known security threats, and they upended how we travel. To protect passengers and crews, airports have made finding dangerous items their primary objective. Causing long lines at entry points as travelers remove shoes and give into full-body scans to keep flights safe, but a more thorough cross check is possible by analyzing passenger behavior. Recognizing dangerous behaviors with predictive analytics complements current security measures and promotes better flying conditions.
Linking Data Sets Identifies Risky Behavior
The theory behind behavior recognition is based on the premise that when someone is in the process of carrying out a criminal or terrorist act, that person exhibits behavior that is out of the norm. For example, take the Underwear Bomber, known for a thwarted 2009 attack. He wore his bomb-laden underwear for three weeks leading up to the attempt to make sure his behavior appeared normal. And yet his behavior was far from normal once he entered the airport.
Video and eyewitness accounts of the underwear bomber’s behavior and demeanor before he passed through security clearly showed that he was acting extremely nervous, showing an unusual amount of stress (such as sweating), closely watching the security procedures, and had almost a “tunnel vision” as to what was being checked and not checked.
The 9/11 hijackers’ behaviors were similarly strange. Based on personal discussions with former airline employees who now work for the TSA, the demeanor of the 9/11 hijackers who transited through Newark airport was so worrisome that agents kept the terrorists’ boarding passes, rather than discarding them as usual. Drug smugglers also have a standard behavior MO (modus operandi). They have a keen interest in security procedures and scan the checkpoint before entering, and once there, maintain rigid posture, minimal body movements, and tense facial expressions.

Big data analytics has the potential to dramatically change airport security (rawpixel.com/Shutterstock)
These types of behaviors are often tip-offs that something is wrong, and they can be split into two categories – micro behavior and macro behavior. Facial expressions, perspiration, lack of eye contact are micro examples. Macro behavior is broader movement throughout the space, such as attempting to hide his or her face by turning away when someone approaches; trying to stay out of sight, behind obstructions or shadows to avoid being seen; or leaving an area when the person in question believes he or she has been detected.
Today, humans are responsible for behavior detection, but detecting these behaviors could be more accurate if it was automated with technology. In the ideal scenario, airports would build a 360-degree view of each person. Data collected would be security screenings, behavior tracking, information from other sources such as bookings, travel history, and so on. By applying predictive analytics and reviewing these large sets of structured and unstructured data, airport security would grade each person on their risk potential.
Current security solutions evaluate at a ‘single point in time’ rather than a summation of a person’s entire behavior over an extended period of time. Tracking the full set of passenger data sets has been too expensive and difficult—until now. Recent analytics advancements make it possible to build the 360-degree view. Add in rich graphical interfaces, mapping tools, and geolocation information, and the security team has the resources and insight to understand which passengers in crowded airports are likely security threats.
Ironically, technology is often better than humans at recognizing atypical human behavior. We’ve trusted security, staff, or another traveler to spot something out of the ordinary, but studies show that we are poor observers when it comes to being aware of our surroundings and what’s happening in them. Plus, passengers greatly outnumber staff. Predictive analytics evens out the odds—and gives the advantage to the airport security team.
What is most difficult for staff is tracking all the acts that combine to denote terrorist behaviors. Each of these acts on its own may not raise suspicion. It’s only when we can see their behavior end-to-end that we can get a complete view. Technology makes that end-to-end view–from arriving at the airport parking lot to traversing the airport and boarding the plane–possible. Once predictive analytics identify an individual as high risk, the security team can request a private interview to find out if they need to investigate further.
High-Risk Threats from Within
While threats can come from outside sources such as terrorists or passengers, they can also be instigated from within, planted by a disgruntled airport employee, vendor/tenant, or contract worker. By using predictive analytics, security operations managers can monitor both access and behavior of internal employees and contractors, identifying dangerous insiders and halting an attack before it happens.
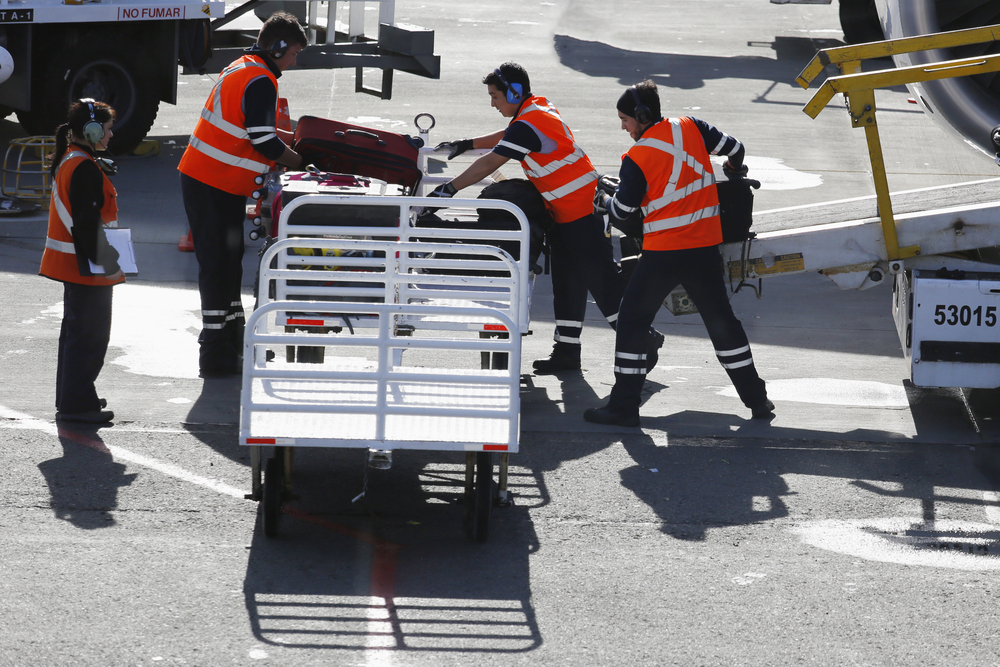
Predictive tools can be used to monitor airport employees, as well as passengers (Leonard Zhukovsky/Shutterstock)
The security team should know which airport employees have access to assets, including physical ones (luggage, airplanes) and cyber information used in daily operations. By gathering big data and using predictive analytics, the security ops team can automatically monitor and note suspicious behavior or irregular employee movements. For example, is an employee assigned to one area of a terminal using a card key trying to enter another terminal? Is a baggage handler at the airport entering a restricted area on their assigned day off?
Using predictive analytics, the security team can correlate data sets on employees from disparate sources and analyze blended threats. The team can use analytics to track: HR (human resources) flags, such as an employee who has a history of performance issues; personal data such as criminal records; information system access including on-site VPN (virtual private network) usage; and physical movement within the airport terminals from badge scans or IoT-based door locks and geo-spatial scanners.
Machine Learning platforms can process these large data sets, and can tie together and connect the dots across multiple behaviors and employee actions. Using in-memory computing, the security team has the speed capabilities—minutes vs. days—needed to use analytics and determine a real-time response that can prevent internal incidents from happening.
Data—The New Crime Stopper
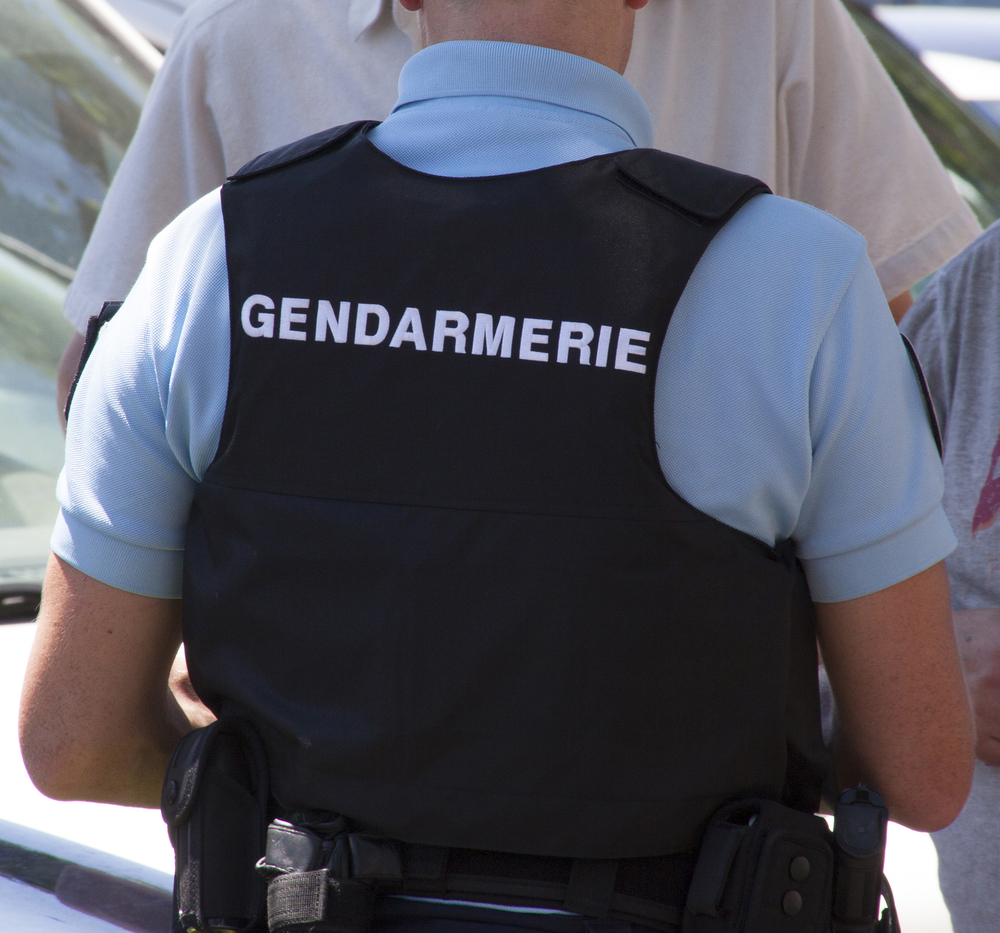
The National Gendarmerie is using SAP software for a big data analytics initiative (JPstock/Shutterstock)
The National Gendarmerie, one of two national police forces in France, is responsible for enforcing laws and protecting citizens in smaller towns and rural areas, and it has adopted predictive analytics for multiple crime stopping duties. Looking to build a new internal culture based on data-driven decisions, the National Gendarmerie re-architected its data platforms so that structured and non-structured data, text, spatial data, pictures and more were accessible for processing.
Now that business intelligence and machine learning are readily available, the National Gendarmerie can predict car thefts, identify national trends for burglaries, and forecast staffing requirements. The team relies on mapping tools to visualize and decide which areas most need patrol teams. Another graph quickly lets the staff understand where department vehicles are allocated and which areas need more resources. To reduce car thefts and burglaries, the operations team uses predictive analytics to identify hot spots and patterns by the location and timing of the crimes. Sentiment data, data based on social media feed, is also added to the mix so that the communications department can track the group’s online reputation.
Like other police departments in the United States, the National Gendarmerie is adding data analytics to its armory of crime fighting weapons. They are not abandoning proven techniques, but modernizing the force.
Criminals get more wily by the day and their schemes get harder and harder to unravel. When law enforcement has the best resources available to them, they are in a much better position to prevent crime and keep innocent passengers and residents safe and secure. Detecting dangerous passenger items was a good first step for airports. A smart next step is to identify the patterns that exist in the huge volumes of data that is out there. With data readily available for analysis, security ops can truly see both who and what pose the biggest risks.
About the author: David Jonker is a Senior Director of Analytics at SAP, where he is responsible for thego-to-market initiatives across SAP’s data management and analytics platforms. Previously, David led product marketing teams for Sybase’s data management & analytics product lines offering solutions for Big Data, data warehousing, cloud, mobility, and embedded systems. David’s career also includes more than 14 years in software engineering and product management roles. David has a degree in Systems Design engineering from the University of Waterloo.
Related Items:
Why Machine Learning Is Our Last Hope for Cybersecurity