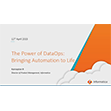
Tracking the Opioid-Fueled HIV Outbreak with Big Data
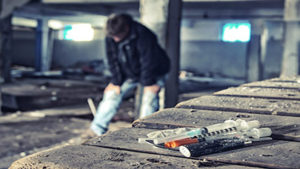
(vidguten/Shutterstock)
As the opioid epidemic spreads across the country, it leaves a trail of death and destruction in its wake. In the Midwest, needle use related to the epidemic is closely linked to a surge in HIV infections. Now the Centers for Disease Control is using big data tools and techniques to understand the macro mechanics of HIV’s needle-tipped spread in the hopes of slowing it down.
In late 2014, a public health nurse in rural Indiana noticed something odd. There was a sudden surge in HIV cases in Scott County, a small county with a population of just 24,000. While the county had long had a cluster of opioid drug addicts with high levels of Hepatitis C (HPV) infection, the HIV virus was practically nonexistent there. Considering that HIV has mostly been detected in urban areas, the spike in cases out in the country was very puzzling, not to mention extremely worrisome.
However, once HIV was introduced to the small, tight-knit group of opioid drug users in Scott County, it quickly spread. By the time the HIV surge ended in mid 2015, nearly 200 intravenous drug users in the county were infected with HIV, the virus that causes AIDS.
Thanks to the work of the public health nurse, the situation was brought to the attention of the CDC, the federal agency tasked with limiting the spread of diseases. The Atlanta, Georgia-based agency brought a number of tools to bear on the problem, including the use of big data analytics.
One of the analytic tools the CDC used to investigate the HIV outbreak was Collaborative Advanced Analytics & Data Sharing (CAADS). Originally developed by Lockheed Martin (NYSE: LMT) Information Systems and Global Solutions, which was recently acquired by Leidos (NYSE: LDOS), CAADS is a Hadoop-based big data framework designed to help customers analyze large, disparate data sources in a collaborative manner.
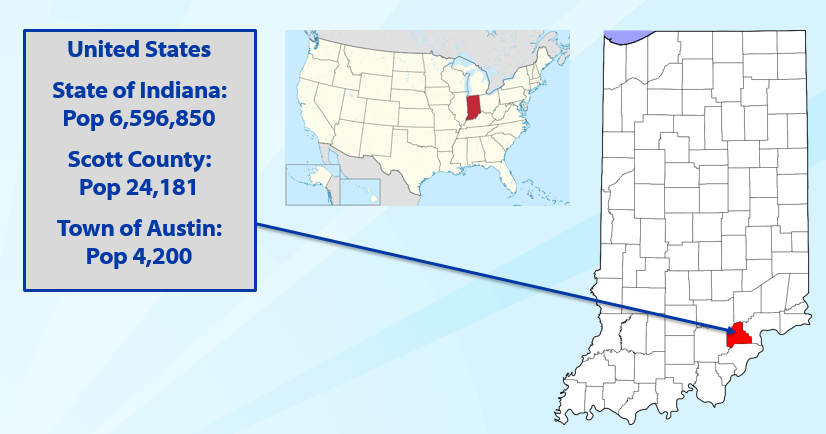
The epicenter of the recent opioid-fueled HIV outbreak (Source: CDC)
CAADS–which incorporates tools from big data partners like Alpine Data, Trifacta, Tableau, Arcadia Data, and Centrifuge Systems–was used by CDC in a pilot project aimed at helping the agency and public health officials to make more informed decisions about the potential of the HIV outbreak to spread, according to Ryan Weil, a principal scientist at Leidos who worked with the CDC.
“CAADS played a role….as force multiplier,” Weil says. “We were actually able to shave the amount of time it took to do the analysis by [a factor of] six. Rather than having to do a tremendous amount of analysis, we’re actually readily able to start getting incremental data products out quickly.”
The CDC used CAADS to ingest, cleanse, analyze, and model a range of disparate data related to this outbreak, including HIV outbreak clusters, geographic factors, epidemiological patterns, and drug resistance data, according to a case study on the Leidos website. “Hidden within those general datasets were even more variables for analysts to consider as potential causal factors for the unprecedented rate of HIV transmission,” the case study says, including factors like transmission through sexual encounters, the role of commercial sex workers, and use of shared needles.
Another factor to consider is that long-time drug users may not be the best source for unbiased information on their addictions, according to Weil, who is based in Atlanta. “Through the use of big data, we’re actually able to fuse the social and interview aspects of the contact tracing with the genetic data, and get a much better picture of the transmission dynamic,” he tells Datanami. “Data analytics has been very empowering for understanding the geo-temporal distribution and onward transmission.”
The machine learning component of CAADS, which is powered by technology from Alpine Data, was particularly helpful in running different scenarios on the potential spread of HIV among the intravenous drug-using populations. This use of predictive analytics was used to come up with a set of recommendations that the CDC could pass down to front-line public health personnel.
“Machine learning helped us with the resources to do GIS-fused link analysis,” says Weil. “The ability to build social networks with the data, and then fuse in other data to link in additional things, was very impactful.”
By having all the different analyses centralized within CAADS, the researchers were able to test various hypotheses, and generate actionable recommendations that could be put to use in the real world.
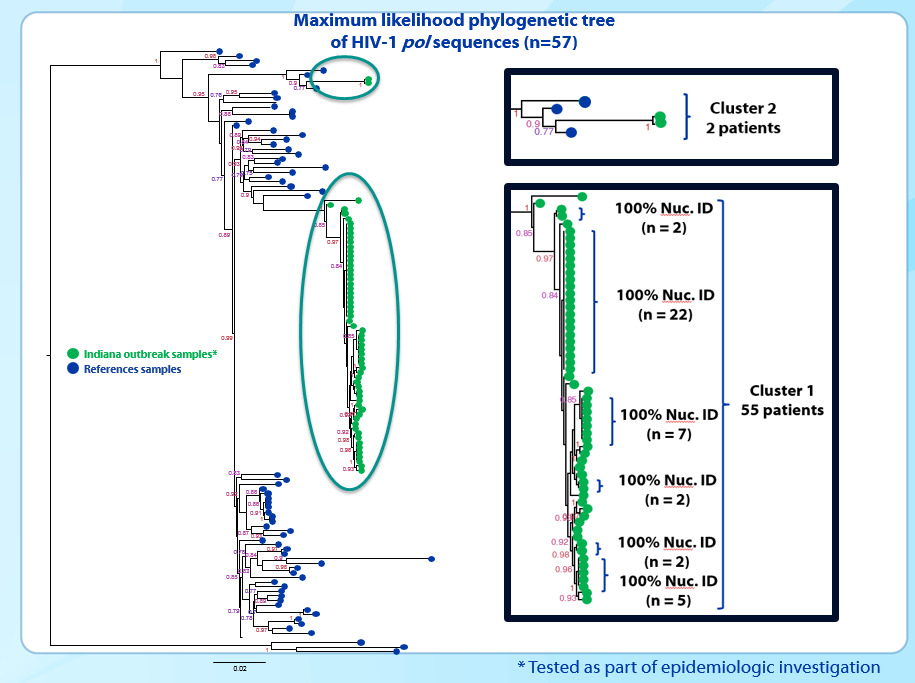
The phylogenetic tree of the 2014/2015 Indiana HIV outbreak (Source: CDC)
“They would be on a call discussing the outbreak and someone would say ‘What if we did it this way?’ They’d step away for a minute, run the analysis, and say, ‘No that didn’t work. But if we do it this other way, here’s the result,'” Weil says. “CAADS and big data allow us to understand both the nature of transmission of the disease, as well as the nature of the opioid epidemic and the prescription drug diversion epidemic.”
By the middle of 2015, the HIV outbreak among Scott County drug users had started to ebb. While Weil could not confirm whether the CDC is using CAADS to study the spread of HIV in specific areas of the Midwest where intravenous drug use and opioid addition continues to be a grave concern–such as in areas of southern Ohio and northern Kentucky–he did confirm that the company is still working with the CDC to study the spread of HIV and other diseases, such as tuberculosis.
“By the end of this, they were able to go in and tell you, okay if you have this number of sexual partners and this number of injection partners, it’s very high risk and we should do immediate and dedicated follow-up for intervention, versus people who were at lower risk,” Weil says. “The power of that was understanding…how it’s spreading, what the factors contributing to that spread are, and how to stop onward transmission, especially with individuals who don’t know they’re infected.”
Leidos, which was spun out of Science Application International Corporation (SAIC) several years back, continues to develop and sell CAADS. The software, which typically runs on Cloudera‘s distribution of Hadoop, is a general-purpose framework that can be used in a range of industries, including cybersecurity and logistics, in addition to public health.
“CAADS is very much built to the support the range of public health aspects and to allow people who would otherwise not be able to get into data analytics to have access and do so in a responsible way,” Weil concludes.
Related Items:
How Data Analytics Is Helping to Fight Human Trafficking