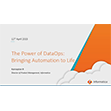
Challenge: Automating Extraction of Mapping Data
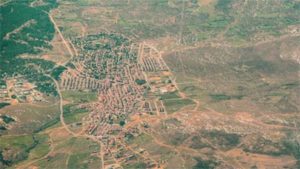
(lumokajinioj/Shutterstock)
A technology competition sponsored by commercial satellite imagery vendor DigitalGlobe will seek to advance development of machine- and deep-learning algorithms that would be applied to geospatial data for automated mapping.
The competition aims to speed development of applications such as automated mapping of changes in the urban landscape and monitoring the accuracy of maps used by self-driving cars.
DigitalGlobe (NYSE: DGI) said the Topcoder crowdsourcing community would use its SpaceNet repository of global satellite imagery along with “labeled training data” released during the summer by data scientists and developers. Volunteers will used the datasets to develop algorithms for extracting mapping data from high-resolution satellite images.
The company said its SpaceNet imagery includes about 1,900 square kilometers of high-resolution imagery collected by its WorldView-2 commercial satellite. Along with eight spectral bands, the dataset also includes nearly 220,600 building footprints derived from imagery that can be used as training data for machine learning.
The dataset was released to advance algorithm development used to automatically extract ground features such as roads, buildings and points of interest. Data formats include GeoJSON for building footprints and GeoTIFF, a public domain metadata standard that allows “geo-referencing” information such as map projections to be embedded with a TIFF file. That capability allows precise mapping of each pixel in an image to a specific location on Earth.
The imagery data is stored on the Amazon Web Service (NASDAQ: AMZN) Simple Storage Service. New imagery and ground features are updated quarterly.
Along with the crowdsourcing effort, DigitalGlobe is also working with graphics processor vendor Nvidia Corp. (NASDAQ: NVDA) and CosmiQ Works, a startup funded by the CIA venture fund, In-Q-Tel. The trio compiled the SpaceNet repository for applications such as infrastructure mapping, land usage classification and population estimates.
As part of the effort, Nvidia said it is demonstrating mapping applications based on GPU-assisted deep learning. Among the goals is demonstrating how its tool can be used to train two different types of convolutional neural networks to detect buildings using SpaceNet imagery.
Meanwhile, the SpaceNet Challenge coordinated by Topcoder is the first in a series of planned competitions focused on developing new computer vision algorithms for automated mapping. The organizers said the first challenge centers on extracting two-dimensional building footprints from DigitalGlobal imagery. The competition includes $34,500 in prizes.
DigitalGlobe collects about 3 million square kilometers of imagery a day, or about 70 terabytes of data. However, “Online map data is lacking for many places such as in developing countries and rapidly growing urban areas,” the company stressed in announcing the competition.
Since satellite imagery contains data from multi-spectral bands beyond visible light, the company said it was “eager to see how this data can be used to improve algorithm performance.” The SpaceNet dataset includes eight bands of multispectral data.
Recent items:
The Here and Now of Big Geospatial Data
5 Ways Geospatial Data is Driving Analytics In the Real World