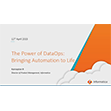
Data Analytics in Higher Education: A Mixed Bag
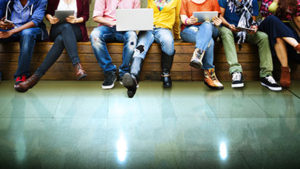
(Rawpixel.com/Shutterstock)
Like many companies in the United States, American universities are looking to big data to give them a competitive edge in attracting, educating, retaining, and ultimately graduating students. However, the actual application of data analytics is not balanced across the board, and in some cases is actually hurting students, a new report suggests.
In “The Promise and Peril of Predictive Analytics in Higher Education,” New America policy analysts Manuela Ekowo and Iris Palmer cover both ends of the analytics spectrum in higher ed, including universities that have found positive uses for big data tech, as well as some that may be abusing the insights they deliver.
On one end of the spectrum is Georgia State University, which used analytics to identify (mostly minority) students with a higher risk of dropping out, with the goal of getting them the help they need to get back on track. The college’s so-called “GPS system” tracks more than 800 different metrics in real time, and has thrust the university into a leadership role in the use of analytics on campus.
On the other end of the spectrum is Mount Saint Mary’s University, which also attempted to get a better view of how students were doing by using predictive analytics—but with the goal of encouraging students on the bubble to drop out, thus helping to boost the college’s retention rate. While the president of the private Catholic college says his goal was to help struggling students get a fresh start and avoid high tuition, the fact that the college didn’t inform students of the purpose of the survey (the data collection method) is troubling.
“As these examples show, predictive analytics can be used to help low-income and minority students overcome the odds and succeed in college, or it can be used to shut them out altogether,” Ekowo and Palmer write in the report.
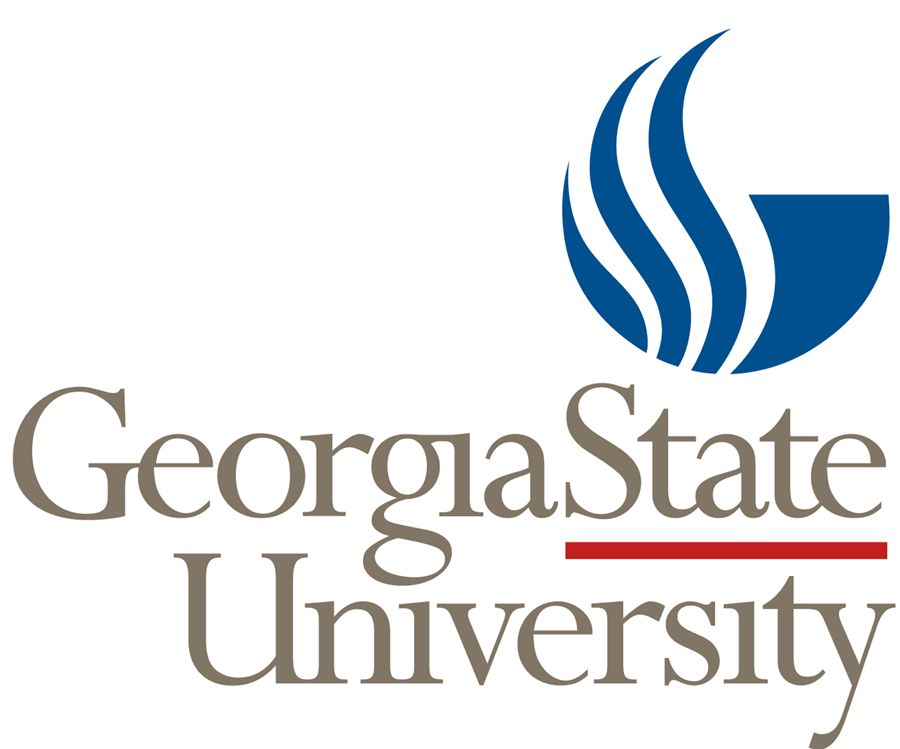
Georgia State University was lauded for its use of predictive analytics to keeps students on track
What’s more, if an algorithm is created to model the world as it exists today, that can help perpetuate injustices. “Predictive tools can also produce discriminatory results because they include demographic data that can mirror past discrimination included in historical data,” the authors write.
Colleges that use predictive analytics in the enrollment process “run a serious risk of disfavoring low-income and minority students, no matter how qualified these individuals are for enrollment,” Ekowo and Palmer write. “Predictive models that rely on demographic data like race, class, and gender or do not take into account disparate outcomes based on demographics may entrench disparities in college access among these groups.”
The fears of big data bias are not new. The Federal Trade Commission touched on that very subject in a report issued earlier this year titled “Big Data: A Tool for Inclusion or Exclusion.” However, the risk is somewhat mitigated by the fact that predictive analytics are not yet in widespread use among colleges and universities. The report cites a KPMG study that found 41% of colleges were engaged in predictive analytics. That’s a bit behind overall 62.5% adoption rate of predictive analytics in the private sector, as measured through a NewVantage survey of Fortune 1000 firms this January.
And not all colleges use analytics in the same way. Some institutions, like the two mentioned above, use analytics to intervene in students’ paths to becoming a graduate or a dropout. But there are other uses, such as learning analytics, course recommendation systems, and enrollment management.
Adaptive Learning
Adaptive learning systems are fairly new, the authors write. But one such system exists at Colorado Technical University, a for-profit university owned by a publicly traded company.
The university’s “intellipath” system was launched in 2012 to help fine-tune the content and curriculum presented to math and science students. The machine learning-based program, which was developed in partnership with Realizeit, resulted in a 27% increase in the graduation rate for an Accounting I class. What’s more, the average grade of students in that class went from a D+ to a C+.
Today, about 30,000 CTU students use the intellipath program, primarily students taking online classes. All students, faculty, and staff have been trained on it, the authors write.
Recommendation Systems
Recommendation systems—a staple of big data analytics in the retail world–are also finding their way into universities.
Five years ago, Austin Peay State University, a public university based in Tennessee, rolled out a program called Degree Compass that helps direct students to majors in which they are more likely to be successful. The system uses a collection of 100,000 past student grades to chart a class progression through degree programs.
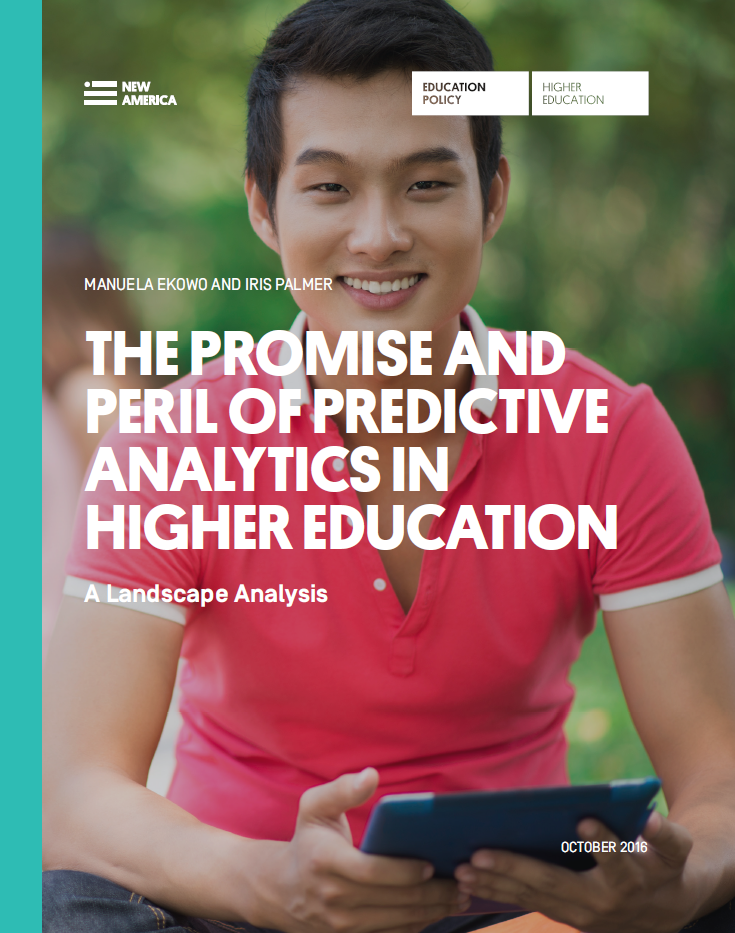
New America analyzed the use of big data analytics in universities in this new report
Since Austin Peay adopted the program in 2011, four other Tennessee universities have adopted it, the authors of the report write. The university also launched a related program called My Future that mines student data to find courses that are important for each major.
Enrollment Management
Enrollment management is another use of predictive analytics in higher-ed. By getting a better idea of the size of an incoming class, colleges are better able to determine what its financial needs will be.
Colleges also use analytics to narrow recruitment and marketing efforts, the authors write. Analytics are helping colleges to find students who are most likely to apply, enroll, and succeed in the four-year programs. This is beneficial for the students in light of the high cost of college fees and the student loan debt that graduates often carry. But it’s also beneficial for colleges, which spend more money recruiting new students than retaining existing ones.
Common factors that go into the recruitment and retention analytics, the authors write, are race, ethnicity, zip code, high school, anticipated major, and level of interest (as measured through requests for campus tours or promotional materials).
One college that’s brought data science to the scholarships process is Jacksonville State University. The Alabama-based school adopted the Illume platform from Civitas Learning, and discovered that many of the students it had given scholarships to were transferring to other schools. JSU revised its scholarship policy as a result.
At the end of the day, data analytics is only a tool, and it’s up to the user to wield the tool effectively. Proper training is essentially to ensure that the tool is not misused, and to stress that the analytic techniques can only illuminate probabilities and can’t predict the future. The authors also stressed the need for greater transparency, particularly when using analytics for enrollment matters.
Related Items:
Data Science Education Evolves to Meet Surging Demand
Beware of Bias in Big Data, Feds Warn
Is Big Data the Next Big Civil Rights Issue?