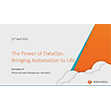
Elusive Seizure Signal the Subject of Kaggle Competition
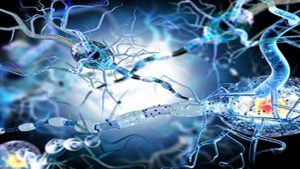
(Ralwel/Shutterstock)
Epilepsy is a debilitating neurological disease that impacts the lives of 1 to 2% of the world’s population, who generally have no idea when their next seizure will happen. Medical researchers who have been stumped so far in using brain-wave data to predict seizures in a large segment of the population are hopeful that a data science competition at Kaggle will point them in the right direction.
Epilepsy has been a medical mystery to humans since the dawn of time. The ancient Babylonians thought epilepsy was caused by demon possession, while Greeks considered it a curse from the gods. Royalty in the Middle Ages considered it a sign of great intelligence, while others considered it a form of witchcraft.
It wasn’t until the late 19th century when epilepsy’s electrical connection was discovered. Since then, there has been some success in treating epilepsy, particularly with drugs. But some people do not tolerate the drugs well and opt for the occasional seizure instead. Researchers hoping to provide more targeted treatment of seizures now are turning toward the troublesome problem of seizure prediction.
So far, researchers have had mixed success in predicting seizures. In one long-term clinical trial, researchers at the University of Melbourne were able to successfully predict seizures with a high degree of accuracy in about half of the research participants who had their brainwave data, called electroencephalography (EEG), collected from electrodes. The early research involved a device with electrodes placed on the surface of the brain that could give the patient a warning if it detected the onset of a seizure.
“This worked fairly well for about half of the 15 patients who participated, but not so well for the others,” says University of Melbourne Professor of Engineering David Grayden, who has researched epileptic seizures for the past decade. “We’ve been analyzing this data and trying to learn as much as we can, especially for the patients for whom the prediction wasn’t so good. We’ve decided to take the data from three patients and put it onto the Kaggle website as an international competition to see what can be done by anybody that wants to have a try.”
So far, more than 630 teams of data enthusiasts from around the world have participated in the Kaggle competition, which is titled “Predict seizures in long-term human intracranial EEG recordings.” With 28 days left and $20,000 in prize money on the line, the competition’s sponsors are optimistic that even more progress will be made in predicting seizures. Already, the leaderboard shows the top teams have achieved a 70% to 75% accuracy rating in using various machine learning approaches to seizure prediction.
“That’s one the best benefits of using Kaggle to do a competition,” Professor Grayden tells Datanami. “It’s a community where people try to solve many different kinds of problems. Most of them will not be at universities or med-tech companies. They’ll be people who have done a lot of business modeling or economic modeling or many other things. They’re going to see this and want to have a crack at it.”
What makes this particular Kaggle competition unique is the data. Never before has such a large amount of continuously EEG recordings from single patients been made available to the public. The data itself is composed of 10-minute windows of data from the three epileptic patients who participated in the study for between six months and three years. Half of the 10-minute data sets are EEG data just before the patient had a seizure, while the other half is for EEG data that occurred far from seizures. Each patients had 16 electrodes placed on their brains to collect EEG data.
The goal is to find some commonality between the two batches of data. “If you can discriminate between the ones that are close to seizures and the ones that are far away from seizures, then you’re effectively doing predictions,” Professor Grayden says.
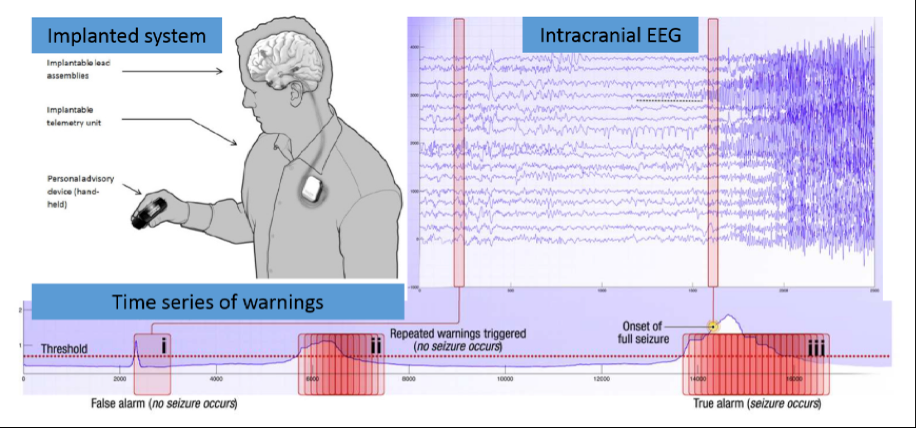
There are 28 days left in the Kaggle competition to predict seizures from brain-wave data
That’s easier said than done, of course. “It looks like white noise pretty much,” says Levin Kuhlmann, a Senior Engineering Researcher and Research Fellow in the NeuroEngineering Laboratory at the University of Melbourne’s Department of Electrical and Electronic Engineering.
Kuhlmann and colleagues successfully employed a K-nearest neighbor algorithm to extract features from the EEG data for the 50% of patients whose seizure could be predicted. But that approach, for whatever reason, just doesn’t work for the other half. The Kaggle contest will give people a chance to see how other features and classifiers pan out.
This isn’t the time the University of Melbourne has hosted a data science competition to try to further the study of seizure prediction. Two years ago, the university and Mayo Clinic put together a hackathon at bi-annual meetup of epilepsy researchers to study long-term EEG data collected from dogs. That competition resulted in the assembly of a generalized linear modeling (GLM) classifier that could successfully predict seizures in dogs about 80% of the time. The results were recently published in the journal Brain.
Now the researchers hope to build on that success with the current Kaggle competition. To that end, MathWorks, the company behind the popular MATLAB line of statistical tools, is providing all Kaggle competitors with the GLM-based model that was used to predict seizures in dogs.
“What we’re doing for this competition for long-term human data is encouraging people to reproduce the methods to see how they perform on this data set,” Kuhlmann tells Datanami. “Mathworks has actually created starter code using some of the algorithms from the winners of the last contest, so people can try out the winning algorithms.”
While the University of Melbourne researchers prefer MATLAB for the ease of prototyping models, the Kaggle participants are free to use whatever approaches they like. In fact, that’s the whole point of providing this data set and opening the competition to anybody in the world—to let a new group of folks with different backgrounds get a fresh look at the data.
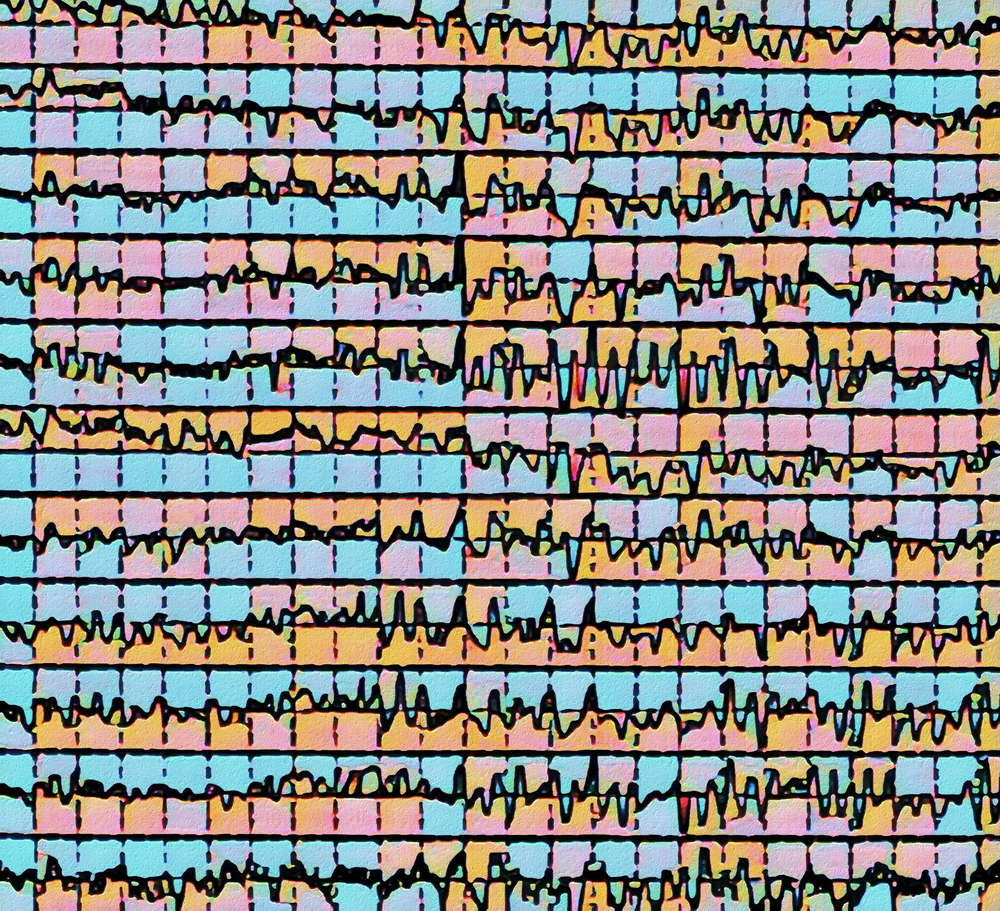
Sample of EEG brain-wave data (xpixel/Shutterstock)
“There are so many people working on this….who are likely to use their pet algorithm or pet machine learning approach on it,” Professor Grayden says. “As a single research group here in Melbourne, we can’t be learning about and trying everything like that. It’s allowing us to sample the different approaches the community has already been trying on different things and see if it works on this one.”
Deep learning networks is one of the most popular statistical approaches these days. Some of the Kaggle participants will undoubtedly apply deep learning techniques on neural networks to the problem. Will it work? The jury is still out.
“Whether [deep learning] is going to be useful for EEG data is still not certain yet,” Kuhlmann says. “It’s been used for things with a lot of strong structure in the data, things like images and speech. But EEG–there’s probably strong structure in the data, but it’s not clear if the brain is sampled well enough to go and build that structure. You can only have so many electrodes in your head.”
Bringing a “pure data” approach to a challenging problem in medical science is another intriguing aspect of this Kaggle competition, says Vijay Iyer, the neuroscientist community liaison for MathWorks.
“There are a lot of people who have looked at this problem for many years with a lot of signal processing expertise, who are somewhat new, perhaps, to deep learning and machine learning and those kinds of pure data approaches,” Iyer says. “Maybe by bringing them in, we may get the best of both worlds and start making progress on this problem.”
At the end of the day, it seems likely that we will eventually be able to predict seizures in most of the population. It’s just going to take a lot of hard work by a large cross-disciplinary group of experts, ample funding, and the perseverance to leave no data science stone unturned. A little bit of luck wouldn’t hurt either.
Related Items:
How a ‘Nuisance Variable’ Turned Into Potential Lifesaver
Accelerating Drug Discovery with Machine Learning on Big Medical Data
Data Miners Excavate Human Brain
Editor’s note: This story was corrected. The data involved in this research was not collected from stentrodes. Also, the stentrodes were not developed by NeuroVista. Datanami regrets the errors.