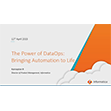
Using Emojis to Boost Sentiment Analysis
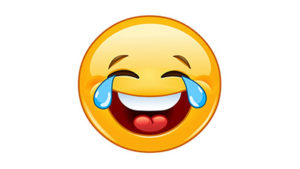
Via Shutterstock
The ongoing challenge of extracting knowledge from soaring volumes of unstructured data using text analysis tools and natural language processing technology now includes novel approaches like “emoji analytics” and parsing email threads.
The latest example comes from Lexalytics, a Boston-based cloud and on-premise text and “sentiment” analytics vendor, which released the latest version of its text analytics platform buttressed by expanded machine learning capabilities. The analytics platform targets social media marketers and “customer experience” managers sifting through customer emails as well as text related to customer reviews that include the growing number of emojis.
Lexalytics claims analyzing emojis can uncover meaning and sentiment in ways regular text analytics cannot. The vendor cited the social media example of a “nauseated face” emoji in response to a food vendor’s latest product. The discovery can be used to either alert other customers or notify marketers who can search for social media posts that mention the term “nausea.”
The latest version the company’s Salience text analyzer also ingests email databases, eliminating duplicate emails by stripping out headers and footers. The resulting email threads can be analyzed to, for example, decipher customer support emails to pinpoint the most vocal complaints.
The upgraded platform also includes a new tool that helps “train” for sentiment analysis by combining a relatively straightforward machine language system with the company’s natural language processing technology. A common problem in trying to analyze customer sentiment using a single model is that results are often skewed over time, the company said. The upgraded Salience trainer can parse text that has been “appropriately marked up for sentiment” analysis. The trainer then returns a list of phrases or suggested scoring for a body of text, thereby speeding up the process of training sentiment analysis tools.
The upgraded text analysis engine also is touted as improving “name identity recognition” as the company seeks to make inroads in Asian markets. The feature is designed to help recognize names in China, Japan, South Korea and other Asian countries.
The enhanced machine learning capabilities are intended to help train and “tune” analytics software while “improving the way machines and humans interact by expanding the capabilities of what text analytics and [natural language processing] can accomplish,” Lexalytics CEO Jeff Catlin noted in a statement.
Other unstructured data miners have taken a deep learning approach in which models run atop a combination of CPUs and GPUs to help customers analyze text and data. Another Boston startup, Indico, is taking that approach to squeeze more sentiment analysis out of unstructured data. “The main barrier to sentiment analysis is not making a better model. It’s getting more data,” Indico CEO Slater Victoroff told Datanami earlier this year.
Recent items:
Unstructured Data Miners Chase Silver With Deep Learning
Why Twitter is the Low-Hanging Fruit of Social Analytics