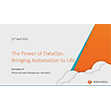
Reporter’s Notebook: 6 Key Takeaways from Strata + Hadoop World
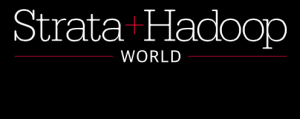
The big data ecosystem was on full display at last week’s Strata + Hadoop World conference in San Jose. At the ripe old age of 10, Hadoop is still the driving force, but newer frameworks like Spark and Kafka are gaining steam. Here are some of the top trends your Datanami editor pulled from the show based on observations and discussions with attendees and vendors.
Let’s start with the biggest news from Strata, which was the rise of Kafka and real-time streaming. As Kafka creator Jay Kreps tweeted it seemed “like every other presentation at Strata this year was on streaming data.” That’s because this was….
Kafka’s Spark Moment
If 2015 was the year of Apache Spark, then 2016 so far is shaping up to be the year of Apache Kafka. The open source messaging framework has emerged as the standard way of moving big data, no matter what the sources and targets of the stream.
Just as Databricks emerged to champion Spark, we’re seeing Confluent rising up to lead Kafka. Founded by the original LinkedIn developers who created Kafka—including CEO Kreps and CTO Neha Narkhede–Confluent is at the epicenter of a new way of thinking about big data.
Confluent made a series of announcements leading up to the show, including the launch of Kafka Connect, which provides an easy way for developers to create data connectors; and Kafka Streams, a stream processing engine that may ultimately displace other real-time analytic engines, such as Storm, Samza, and Spark Streaming.
“Our approach has been to work with developers and it should be super simple,” says Narkhede tells Datanami. “You don’t need your stream processing to dictate how you want to deploy and configure your applications. A lot of this can be built as a lightweight library, assuming Kafka is the common substrate for stream data.”
Real-time streaming data has certainly caught the attention of the Hadoop vendors, particularly with sensor data and the Internet of Things (IoT) showing such promise. Cloudera, Hortonworks (NASDAQ: HDP), and MapR each have launched significant new products in the last year aimed at the real-time opportunity. And while Hadoop may ultimately be a sink for much of this data, don’t be surprised if Kafka gains a foothold in the space. It is, figuratively, as the tip of the real-time spear.
Spark Continues Growth
As a new ecosystem springs up around Kafka and real-time streaming, we’re still feeling the repercussions of a massive shift in big data mindshare towards Apache Spark. Databricks new CEO Ali Ghodsi (who last year replaced Ion Stoica, now executive chairman) sat down with Datanami to talk about the opportunities emerging around the versatile in-memory framework.
Barely two years from its founding, Databricks has attracted about 200 paying customers for its Spark on the cloud service. That’s pretty significant, especially considering that one of those customers (whom the company could not name) has signed an eight-figure deal.
Having the creators of Spark on the payroll gives you a certain competitive advantage, Ghodsi says. “We are really the only company that can solve any problems related to Spark, because we have the committers,” he says. “We can go in and change Spark. We’ll augment it or fix it.”
While Spark has reached critical mass from a technology standpoint, there is still a big gap when it comes to data science education (which Datanami recently covered in a three-part series). “The hottest job in Silicon Valley is data science. You have to put that on your [resume],” Ghodsi says. “But how do you actually learn and get those skills?”
In the past two years, the company has ramped up its education initiatives around Spark and data science in general. In 2014, the company set out to train 2,000 people, and nearly hit its goal. In 2015, it signed up 120,000 people for two massively open online courses (MOOCs), and 22,000 people finished the course.
“We’re doubling down on that this year,” Gohdsi says. For starters, the company launched Databricks Community Edition, which includes a 6GB “mini-cluster” of the Databricks cloud environment that features Spark as well as a cluster manager, a data science notebook, and access to a MOOC.
“We call it democratizing access to Spark,” Gohdsi says. “We want to show them what a great platform it is.”
Hadoop’s Gravity Builds
While Spark and Kafka are shining examples of what big data technology will provide us in the future, Hadoop is still right in the thick of it, as a “middle-age” entering its productive years. According to Hortonworks CTO Scott Gnau, Hadoop simply gives organizations the capability to do things with their data they couldn’t do before.
“The center of gravity is shifting to Hadoop because of the capability to land the data first, then apply a schema, then figure out what the signals and the noise are, and then turn that into something that feeds a downstream decision support system,” he says. “I believe, in data and analytics, we’re truly reaching a tipping point.”
While the use cases often differ from customer to customer, the common theme connecting Hadoop users is a need to store and analyze ever growing amounts of data. One common Hadoop use case that cuts across different industries is the 360-degree view of the customer, he says.
“That 360-degree real time view of a customer is really hard to do with one traditional legacy system,” Gnau says. “It requires feeds from lots of disparate kind of places. That drives the power of the Hadoop system where you have a universal file format, you have pluggable analytic engines that can help analyze different kinds of variable data at very large volume efficiently.”
Analytic Clouds Rising
One of the other trends from Strata + Hadoop World that was tough to ignore was the rise of analytic clouds.
People have been talking about this for a long time. But it would appear that it is now reaching a critical mass, with Amazon Web Services (NASDAQ: AMZM) clearly in the lead, followed by Microsoft Azure (NASDAQ: MSFT), Google Compute Engine (NASDAQ: GOOG) and IBM‘s (NYSE: IBM) cloud also in the running.
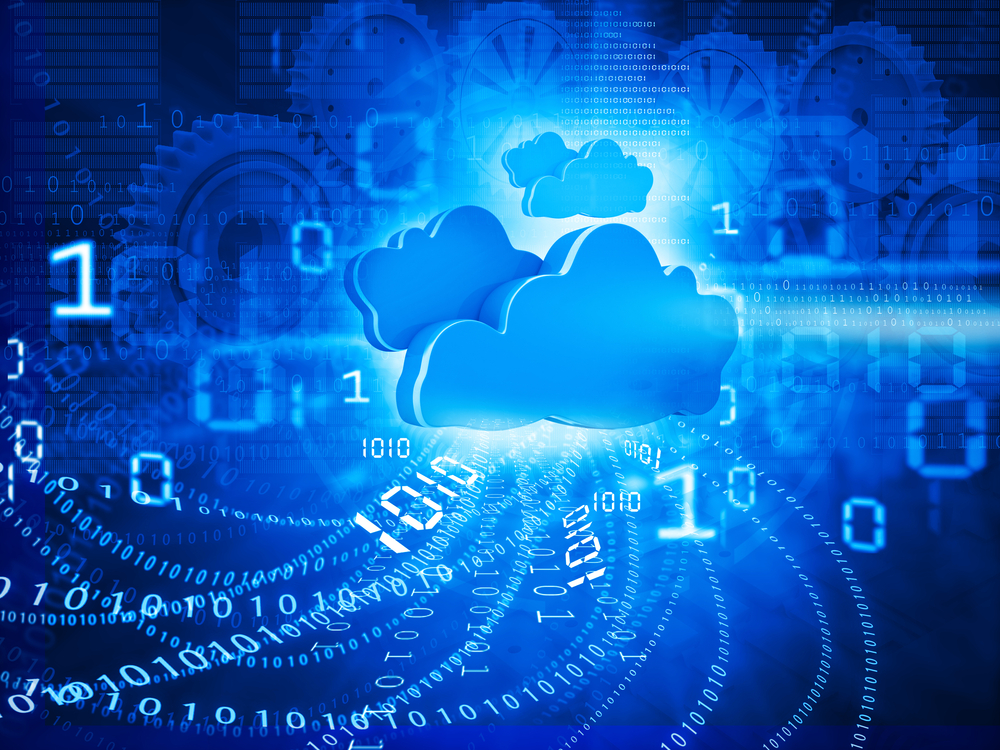
(bluebay/shutterstock.com)
“The cloud is disrupting how things are done,” says Gohdsi, the CEO of Databricks, which runs its Spark service on AWS. “This traditional way of packing all this stuff into machines on the cluster and having a resource manager for it–it’s going away. In the cloud, you buy the services you need and you compose them when you need them.”
At Strata, executives talked about how conservative companies like banks, hedge funds, and major hotel companies were moving their big data infrastructures to the cloud. “We see huge adoption, especially in the last year,” Gohdsi says. “We hear companies saying, ‘We’re moving to the cloud for security. We think Amazon or Databricks can do a better job securing our data than we can ourselves.'”
Machine Learning and Data Prep
Two of the hottest subsets of the big data analytics space are machine learning automation and automated data preparation and transformation.
In the ML space, we see companies like Dato, H2O, and Skytree helping companies to automate their predictive mechanisms in Hadoop and parallel data warehouse environments. That’s not to take anything away from existing firms, like SAS and Mathworks, which still have very viable products.
Considering the overarching trend towards the cloud, we’d be remiss if we didn’t mention the ML offerings of companies like AWS, Microsoft, Google, and IBM, which certainly showcased their wares to Strata attendees. We also noticed a newcomer, DataRobot, which closed a $33 million Series B round in November and seemed to have a good showing at Strata.
The ML space is hot and will get hotter as more organizations move from simply collecting big data to actually doing something with it (i.e. predictive analytics). That puts ML automation companies in a prime position, according to Rajat Arya, a presales technician who was employee number one at Dato (formerly Graphlab).
“The more you’ve built stuff from scratch in ML, the less interested you are in doing it again,” Arya says. “If you’ve hand-written a neural network, you don’t want to write another one because you realized how difficult that is.”
On the other end of the big data spectrum—perhaps we should call it the beginning, if you consider it to be a pipeline–are software companies making data preparation and transformation tools. At the Strata + Hadoop World conference two-and-a-half years ago, Paxata had the niche all to itself, says Nenshad Bardoliwalla, a co-founder and VP of products for the company.
Now the company has a host of competitors at the show, from pure-play startups like Trifacta, Tamr, and UNIFI Software to ETL standouts like Informatica, Talend, and Pentaho. Even big data vendors who you might know for other things, like Platfora, Zaloni, and Datameer, are playing up data prep as a big part of what they do.
It’s no wonder that Gartner says the next big market disruption in the space will be self-service data preparation, which aims to reduce the manual data munging that consumes upwards of 80 percent of a data scientist’s time. “We’ve pioneered a much larger business,” Bardoliwalla tells Datanami. It’s spread “well beyond our imagination.”
Revenge of the (Mainframe) Nerds
Before big data was its own thing, mainframes housed the biggest datasets for global corporations and national governments. Today there are tens of thousands of the monolithic dinosaurs walking the land from vendors like IBM, Fujitsu, and Unisys.
While you don’t see any mainframes at Strata + Hadoop World, the systems remain vital cogs in the information engines at the biggest companies in the world, and that means they command a certain respect (and let’s be honest, fear) from those who would dare tap a mainframe and spill out its data.
One of the vendors active in this space is Syncsort. The New Jersey-based company is doing a tidy business selling ETL software that links IBM mainframes with new distributed systems like Hadoop. During a briefing at the show, Syncsort CEO Josh Rogers explained the significance of the mainframe opportunity to Datanami.
“There’s obviously a lot of disruption happening in the analytics space broadly speaking with large enterprises rethinking what are the way architectures apply to the different types of questions they want to ask,” Rogers says.
The new systems let users query lots of new data sources at much higher volumes than they ever could before. “But what remains the same,” Rogers says, “is you need to have your core data assets in those repositories to make sense of data. IoT doesn’t make sense unless you can pair it up with core customer and transaction information.”
“So to do that what we’re doing is customers, particularly large enterprises, have to navigate this path of big iron to big data,” he continues. “They have to figure out how to get the core transaction and customer data that gets generated and stored on the mainframe into not just Hadoop but Splunk, MongoDB, Spark, and Kafka.”
Before big data, Syncsort served the ETL needs of mainframe customers. The company, which is 48 years old, has about 2,500 customers, who are predominantly big companies that have mainframes. “It’s a much bigger opportunity than we thought,” Rogers says. “When big data meets mainframe it turns out to be a hard thing.”
Related Items:
Cutting On Random Digital Mutations and Peak Hadoop
Finding Long-Term Solutions to the Data Scientist Shortage