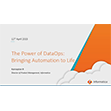
For Data Scientists, What’s in a Name Really Matters
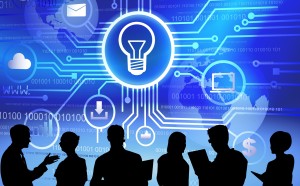
(Rawpixel/Shutterstock.com)
Shakespeare once pondered the nature of names, pointing out that “a rose by any other name would smell as sweet.” For data scientists, the meaning behind the title is not just an epistemological exercise, but a practical problem that has consequences upon that delicate dance between employer and employee.
The data scientist shortage is having all kinds of impacts on how organizations approach big data projects. As we explored in the previous story in this series, the shortage is leading many organizations to consider data science as a team sport. That’s the approach espoused by Bill Schmarzo, a VP at EMC (NYSE: EMC), who is encouraging business managers to “think like data scientists” to identify potential metrics and variables that data scientists (and data engineers) can then validate and put into production.
This approach bears similarity to the rise of so-called “citizen data scientists,” an approach that has been backed strongly by Gartner. Citizen data scientists differ from full-fledged data scientists in that they lack the full suite of skills (math/statistics, programming/computer science, and business/industry experience) that a data science is traditionally expected to have. Citizen data scientists are essentially data analysts who possess light statistical and programming skills, and lean on sophisticated software to fill in the gaps compared to full-fledged data scientists.
One supporter of the citizen data scientist movement is Peter Schlampp, the vice president of products for big data analytic solution provider Platfora. “We identified years ago we need more data scientists and universities and companies have caught onto this and they’re educating and training people to do this at school and on the job,” he says. “But the gap will remain. Before we get there, we need to do something.”
That something is backing the citizen data scientist movement. “A citizen data scientist is somebody who’s on the spectrum between information analyst and data scientists,” he says. “There’s a category of traditional information analyst, the person who uses Tableau or Excel, who is curious and wiling to use new tools. They might know SQL, they might know a little bit of R or Python, but they’re not a stats expert or programmer necessary. They’re not really a true data scientist.
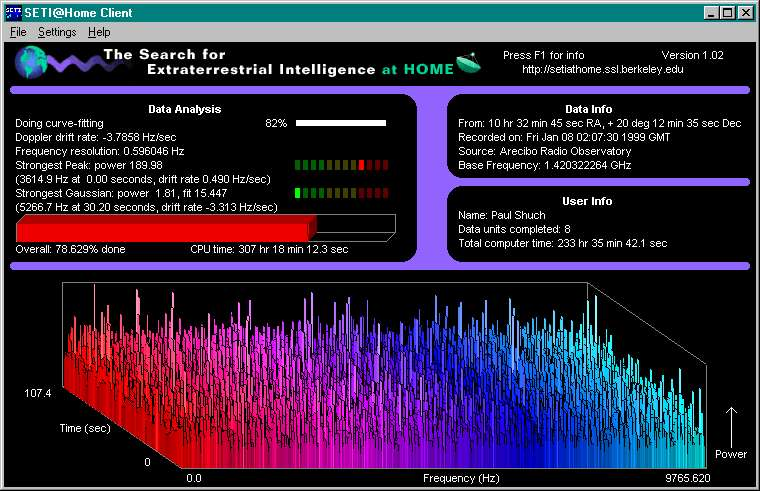
The SETI at Home project started in 1999 is considered to be among the first citizen data science initiatives.
Gartner recently predicted that the number of citizen data scientists is going to grow five times faster than the number of true data scientists through 2017. Platfora and other companies like it are fully onboard with the idea of empowering citizen data scientists to handle some of tasks that a traditional data scientist would normally do. “We think about how to essentially use things like machine learning or visual analyses of big data to help people understand big data without necessarily having all the skills you need to be data scientist,” Schlampp says.
This wider view of data science as a team sport jibes with how Kirk Borne, the principal data scientist at Booz Allen Hamilton, sees the market shaping up. “The data scientist’s job is becoming better defined in more and more industries, though there are still many cases where organizations are casting a wide net for any type of data science expertise,” Borne tells Datanami.
“The expectations are becoming more realistic,” he continues. “Nobody expects a unicorn anymore (if they ever did)! Most organizations now realize that data science is a team sport. Consequently, they need many different ‘position players’ (with different skill sets) in order to field a successful team. Since most organizations cannot afford to hire a whole new team, there is more internal identification, recruiting, training, and development of existing staff.”
The data science gap is changing quickly, as university programs, bootcamps, and online courses ramping up to educate and train people with data science skills, Borne says. In the meantime, employers are becoming more defined in the job titles they use to attract talent and build a data science team, he says.
“There are many more data scientist training programs…but the demand is growing at least as fast,” Borne says. “The difference now is that, while there are many more job openings, the jobs are becoming better defined.”
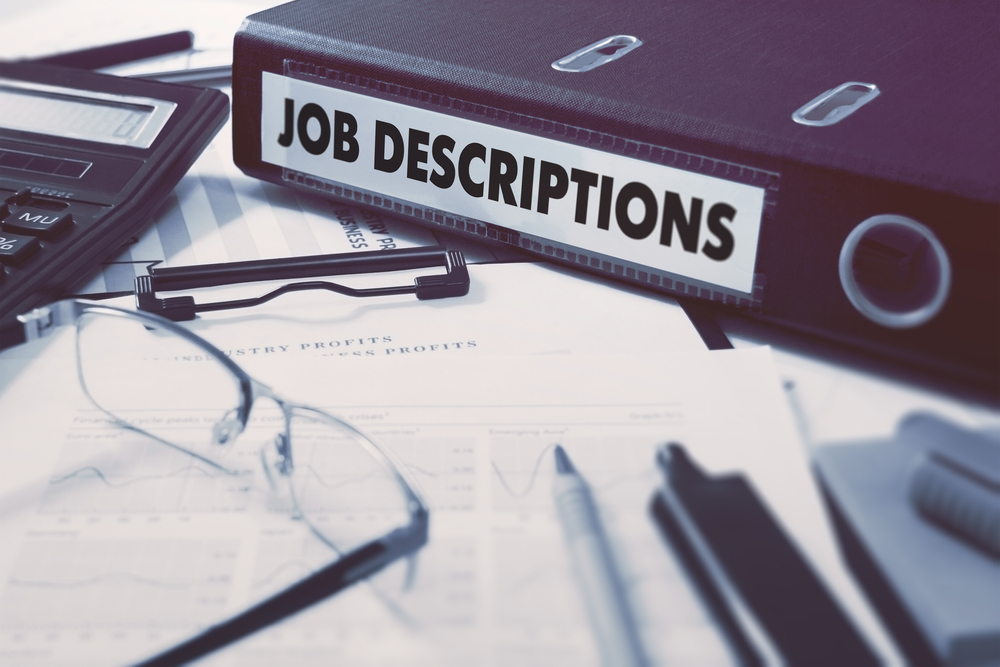
The job description for “data scientist” is currently in flux (Tashatuvango/Shutterstock)
Companies are getting more fine-grained in how they differentiate among people who fall into categories like: data engineers, predictive modeler, cloud analytics programmer, natural language processing (text mining) experts, data visualization (visual analytics) expert, Borne says. “If I had to pick one,” he says, “I guess I would say that the gap is growing: the demand is growing faster than the candidate pool.”
This diversification and greater parsing of what it means to be a “data scientist” is not always a good thing, and may lead to confusion about what a job really entails. Chris McKinlay, a senior data scientist at the Los Angeles-based data science consultancy Data Science, says employers are misleading in job descriptions. “It’s not a job that has a standardized description,” he says. “You have hiring mangers who take something that’s like an analyst job and try to put a sexy name on it like data scientist.”
McKinlay backs a more traditional definition of data scientist as someone who can not only build predictive models and put them into production, but have a grasp of the industry they’re working in. “They’re people who can make strategic decision and bold products that will change the course of the company,” he tells Datanami. “Those people are really operating at the edge of what the company knows. You have to parse enormous amounts of data to really get a solid picture of anything.”
The industry is clearly grasping for solutions to the data scientist shortage. Universities and bootcamps are scrambling to ramp up production of data scientists, and are making a dent in the supply gap. In the meantime, we’re seeing how business managers are being asked to “think like data scientists,” how analysts are being promoted to “citizen data scientists,” and how advanced analytic software can give mere analysts data scientist-like powers.
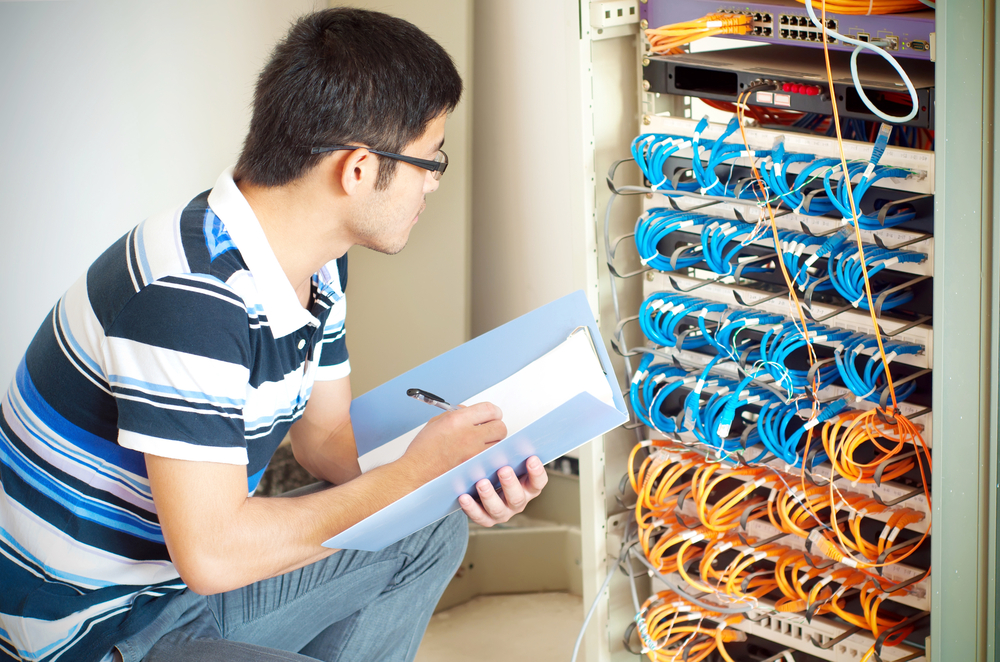
Engineers are becoming vital team members for big data projects (gyn9037/Shutterstock)
But at the end of the day, there are no tricks or shortcuts for people who desire a long career in as a data scientist. Dr. Kirk Borne, who is an advisor to Datanami‘s Leverage Big Data event, says that while bootcamps are great, “…[A] data scientist who plans to make a career in data science better plan to continue lifelong learning in the field, long after their 12-week bootcamp is finished. Consequently, the Master’s degree programs are still the best option for long-term career success.”
And while skills like Spark and SAS and Python and Hadoop are hot at the moment, a successful data scientist will always display certain aptitudes, such as data literacy, curiosity, creativity, communications, critical thinking, problem solving, and computational literacy. Those personal attributes will always be in demand, Borne says, even when the next programming paradigm emerges.
Related Items:
Finding Long-Term Solutions to the Data Scientist Shortage
Tracking the Data Science Talent Gap