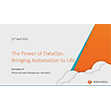
How Big Data Analytics Is Shaking Up the Insurance Business
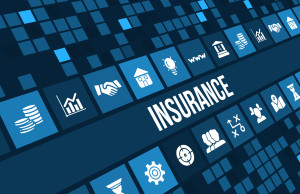
The insurance industry is–by definition and by practice–generally averse to risk. But thanks to the success of early adopters of data analytics, insurance companies in the $1.1-trillion U.S. market are scrambling to ramp up their own data analytics practices before it’s too late.
In his 25 years in the insurance business, Capgemini’s Seth Rachlin has never seen insurance companies move so quickly to change their business models.
“Traditionally insurance has been a slow-moving business,” says Rachlin, who is a vice president within Capgemini Financial Servic’s insurance business unit, and heads up its data analytics practice. “But the pace of change frankly in the past two to three years is something I’ve never seen before within the industry.”
The industry’s “aha” moment occurred when a handful of insurance companies at the bleeding edge of analytics posted stellar results. That triggered a chain reaction that is still playing out today.
“It’s driven by, broadly speaking, the capability of data and analytics to materially impact performance,” Rachlin says. “We’re seeing a tremendous desire to leverage technology broadly, and data more specifically. The business is getting it, and the business is wanting to act on it. And I think there’s even a level of fear of being left behind.”
Roots in Auto
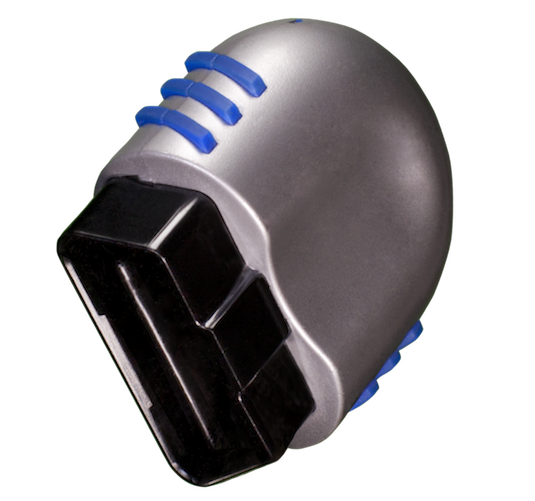
Progressive led the movement into driver informatics with its Snapshot device, which transmits data about when customers drive, how often they drive, and how hard they brake
The analytic transformation originated in the automotive insurance market, which accounts for a big chunk of the larger $500-billion property and casualty (P/C) insurance business in this country.
Traditionally, car insurance companies would price policies based on ratings classes. There were perhaps 10 to 20 variables that went into these pricing calculations–things like the age of the driver, gender, ZIP code, how many miles driven, and driving record.
But then a handful of auto insurance firms started gathering a lot more data about potential clients—such as credit scores and reputational data from Yelp–and using it to populate models that have upwards of 1,000 variables.
All this data allowed the models to be composed of a much higher number of more fine-grained ratings classes. As the ratings classes got smaller and more targeted, it allowed the early analytic adopters to not only price their risk more effectively than their competitors using traditional pricing models, but to lower their claims payouts too.
“That experience of using data and using models built on the data to better price and better select risk – that’s been going on by leading companies for a number of years now,” Rachlin says. “But everybody’s kind of got religion and they’re trying to apply them more broadly across the industry to the issues of how price effects customer acquisition and how data can influence risk selection.”
Parallel Computing Boost
But why is this occurring now? According to Rachlin, there are two main reasons: Advances in the sophistication of statistical modeling techniques and the availability of parallel computing power.
“You need to know a lot less going in [with] a lot of the statistical modeling techniques that are being used today,” he says. “You can kind of throw everything in and see what works, whereas statistical practices 15 to 20 years ago, you needed to have formal hypothesis about why these things matter in order to actually get results out of the model.”
Hadoop does play in role in allowing insurance companies to manage huge amounts of semi-structured and unstructured data, Rachlin says. But more important is “the simple availability of computing power in clustered parallel processing environments to actually make this stuff run.”
It wasn’t long ago that the smart guys at insurance companies relied on nightly batch processes–mostly running on big IBM (NYSE: IBM) mainframes–to calculate the risk or price sensitivity or other factors that would tell insurance companies to pull this lever or that one. If a model run failed or the result didn’t look right, the analyst would make a change, and it would take another day to get the result.
Those batch processes are largely gone today. “The simple ability to run these things close to real-time has had had an enormous impact,” Rachlin says. “If you think about price sensitivity analysis and relationships of price and capital and the ability to run things like Monte Carlo simulations, that was very difficult to do even 10 years ago.”
Commercial, Home, and Worker’s Comp
While data analytics got its foot in the door of the insurance market via the auto segment, it’s now coming to other insurance lines as well, including the commercial building and worker’s compensation businesses.
The commercial and home insurance sector is actively exploring the use of telematics devices to feed data over the Internet of Things (IoT), Rachlin says.
“Commercial insurance companies are looking to using building sensor technology,” he says. “There’s a tremendous amount of innovation in terms of collecting data, using data, and even changing business models based on that.”
Data from social media sites like Facebook (NASDAQ: FB) and Twitter (NYSE: TWTR) are coming in handy for spotting fraud in the worker’s compensation market. “What a lot of insurance companies are doing is crawling Facebook and other sites like that for evidence that the person is not as disabled as they claim to be,” he says.
Analytics in Health
There are not as many opportunities to use analytics in the health insurance business, largely because it’s not a consumer-driven market and health insurance companies do not choose who their clients will be.
But health insurance is still a very data-driven business, Rachlin says. “What you’re seeing is a lot of use of analytics in case management, for the insurance companies to really beat the outcome, to do proactive case management. That’s an area that’s gotten a lot of play lately,” he says.
While the opportunities are not the same, some of the analytic techniques are. Just as auto insurance companies and retailers create many micro-segments to more accurately predict what a given person might do, health insurance companies–and the healthcare providers they work hand-in-hand with—are creating highly segmented patient models based on variables like conditions, diagnoses, and outcomes.
“We know that if somebody is filing their prescription claim every month for condition X that we’re going to have a better outcome than if they appear not to be using the medication as directed,” Rachlin says. “There’s tremendous opportunity there. And there’s a lot of work being done to push the analytics around outcomes down into the treatment space.”
Data Drives Consolidation
The nature of the insurance business is changing, and a lot of that change can be traced back to big data and analytics. No longer can insurance companies simply consult the actuarial tables to calculate the risk of something bad happening to a given car, person, or building—at least not if they want to stay in business for long.
Rachlin expects industry consolidation to result from today’s analytic-driven land grab. “If you think about banking, there’s only a few big banks. Insurance is going to end up like that,” he says. “There’s a tremendous number of small insurers. They’re not going to be able to afford the technology and capabilities to actually be competitive. They’re going to adverse select bad risk and they’re going to have issues. That’s happening already.”
While the prospect of fewer providers doesn’t bode well for competitive pricing for consumers, it’s not all doom and gloom, Rachlin says. “You’re going to see an incredible level of customization at the consumer side, where people will be able to buy the insurance they want and need, priced and tailored to what they’re actually doing. It’s going to come to resemble far more what it does now a more traditional retail-oriented industry business.”
Related Items:
Insurer Embraces Data Analytics to Shape Benefits Coverage
How Better Data Empowers This Medical Billing Company
Preparing for Obamacare’s Big Data Analytics Opportunity