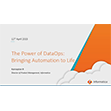
A Visual IDE for Big Data That Promotes Collaboration
Being successful at big data analytics today typically requires a collection of business, computer, and statistical skills. It’s rare to find somebody who possesses all three—that’s why data scientists are called sometimes called unicorns. Now a French company named Dataiku says it’s democratizing analytics by helping individuals who possess pieces of the skill puzzle to come together and work collaboratively.
Dataiku was founded 2.5 years ago by a group of four data enthusiasts who saw a need for better tooling. Where most products on the market focus on a particular piece of the big data solutions, the company saw its flagship product, called Data Science Studio (DSS), as an integrated development environment (IDE) for building big data services.
To that end, DSS it handles everything from data ingestion and cleansing, to machine learning and advanced SQL analytics, to building repeatable pipelines and visualizations. The Web-based software deploys services to a variety of back ends (from Hadoop to Cassandra to SQL data warehouses) and leverages. “Let
Florian Douetteau, CEO and co-founder of Dataiku, described the company’s product development philosophy in a recent interview with Datanami.
“Firstly we have a huge focus on collaboration,” Douetteau says. “We’re seeing projects are a collaboration of several people–data analysts, data scientist and engineers. The product is fully Web based, so people can collaborate in real time on data projects. It’s all about making the transition to allow all the people to meaningfully collaborate at the same time.”
In the real world, it’s common to have one person who knows the business data very well but who isn’t up on the latest algorithms. Antoehr team member will often be very good with data sicnece and algorithms, but who just doesn’t have the same grasp of the data.
“The challenge is to make those two people work together,” Douetteau says. “In a lot of situations it’s not practical to imagine that the data analyst can actually acquire [data science expertise] and it’s not realistic also to imagine that each and every data scientist or statistician can learn all the business implications of whatever they do. So it’s about making people collaborate interactively just because they cannot share all the information.”
A second major design point for DSS is making things visually simply and intuitive. Dataiku lets users do data work—including designing workflows and transformations, and setting up supervised machine learning models—all in from the graphical user interface (GUI), which lowers the skills required. More advanced users who are proficient in R and Python can open code editors in DSS if they need to, but it’s not necessary.
“We built an interface that gives you this ability to manipulate data in a spreadsheet fashion,” Doutteau says. “This way of mixing data management with the preparation and the predictive analyses is I think unique in Dataiku.”
The It’s not an easy problem to solve. I think that in order to solve it in proper fashion you have to have a product and a stack where execution, data connectivity and colaboaration are designed together from the very beginning.
Dataiku has about 60 customers around the world, most of them in France. The company, which raised $3.7 million earlier this year, recently opened an office in New York City to serve the big data analytics needs of the North American market.
Today at the Strata + Hadoop World conference, the company announced support for Apache Spark. This gives Dataiku customers another place to execute analytic applications developed in DSS, and provides a big speed-up compared to DSS apps designed to run atop Apache Hive or Cassandra, the company says. Support for Spark also gives DSS users the capability to use both MLlib and Scikit-Learn machine learning libraries; Spark’s Python and Spark SQL will also benefit customers, the company says.
At the end of the day, Dataiku is swimming in the same big data waters as many other vendors. But whereas other vendors are attacking a piece of the puzzle, Dataiku hopes its message of end-to-end big data simplicity resonates with users.
“You’ve got this huge discrepancy between the big data hype and everything we’re told about big data, and the day to day burden of the data analyst, who has to cleanse his data,” Doutteau says. “At Dataiku, we basic lay want to work on that discrepancy, to turn big data into small problems that can be easily solved.”
Related Items:
Cutting: Spark an ‘All-Around Win’ for Hadoop