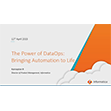
Getting MDM Off the Ground
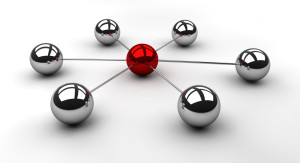
Anyone who has been exposed to Master Data Management (MDM) realizes that the practice often comes with a price. While most individuals applaud the concept of implementing MDM, too often what initially appeared to be an adequately funded and well-planned project starts to miss key deadlines and milestones while accumulating unexpected costs.
Before trying to tackle MDM, it’s important to understand exactly what MDM entails and how it will work within a larger big data management framework. This may sound simple, but it is a critical first step towards implementing a flexible architecture that will grow along with the organization’s needs. Essentially, MDM entails cleansing, rationalizing and integrating data across systems into one ‘system of record’ for core business activities.
There are a plethora of reasons that cause MDM projects to go off the rails. Businesses often face huge initial costs while simply defining and developing the project. Upfront costs for MDM licenses and services can run into the millions–and, even before the system is live, upgrades and license renewals can add even more millions in program costs without returning any value. Still more upfront costs may arise when vendors add various tools to the mix. For instance, the addition of data quality, data integration and SOA tools can triple or quadruple the price.
Given the high cost typically associated with getting an MDM project underway, project teams are under extreme pressure to garner as much value as they can, as fast as possible. However, these teams quickly realize that the relevant data is either buried in hard-to-access silos or is of poor quality – inaccurate, out of date and riddled with duplication. This means revising the project schedule and even more of a cost increase.
The Consolidation Method
One way of avoiding these issues is starting small, using the MDM Consolidation method. This approach entails pulling data into the MDM Hub (the system’s repository) in order to perform cleansing and rationalizing. The benefit to this approach is that consolidation has little impact on other systems.
Consolidation is a good way to begin learning critical information about your data, including duplication levels and data quality issues. The downside is that such learnings can necessitate refactoring and rebuilding the MDM Hub, which can take several months. This is another highly expensive proposition and involves bringing in a team of systems integrators and multiple software vendors.
In order to achieve a rapid return on MDM investment, project teams often skip the consolidation phase and go directly to a co-existence type of MDM. This solution includes consolidation but adds synchronization to external systems to the mix. Typically, data creation and maintenance will co-exist in both the MDM system and the various data sources. However, this approach introduces challenging governance issues related to data ownership, along with data integration challenges such as implementing a service-oriented architecture (SOA) or data services.
There are other types of MDM, each with its own set of problems. As a result, the company implementing an MDM system winds up buying additional software and undertaking supplementary development and testing, incurring even more expense.
A Different Approach
Rather than getting tangled up in time crunches and overbearing implementation costs, companies should look to get MDM projects underway slowly and with a minimum amount of upfront costs.
Using open source tools can allow companies to build data models, extract data, and conduct match analysis while simultaneously building business requirements and the preliminary MDM design, at a fraction of the resource costs associated with more traditional approaches.
This approach to MDM deployment is particularly effective when combined with other open source tools that form the foundation of a comprehensive big data management solution. These include big data integration, quality, manipulation and governance and administration. Following this path to affordable, effective MDM that works within a larger big data management framework will enable implementation of a flexible architecture that grows along with an organization’s needs.
With the preliminary work in place, this alternative approach also provides the tools needed to subsequently scale the project. It is efficient enough to support the heavy development work necessary to create a production version MDM system without breaking the bank. Once in an operational state, a company can scale up or down depending on changing MDM requirements. And, when the major development phase is over, it is possible to ramp down to a core administrative group, which will significantly reduce the cost of the application over time.
About the author: Mark Balkenende is a Sales Solution Architects Manager at Talend. Prior to joining Talend, Mark has had a long career of mastering and integrating data at a number of companies, including Motorola, Abbott Labs, and Walgreens. Mark holds an Information Systems Management degree and is also an extreme cycling enthusiast.
Related Items:
Connecting the Dots on Dark Data
The Land of a Thousand Big Data Lakes
How Big Data Keeps Yellow Pages Group in the Black