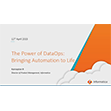
Dato Aims to Unleash Machine Learning
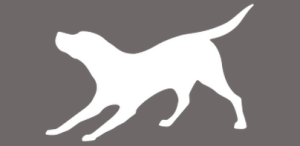
The new year started with a bang for GraphLab, the big data analytics startup headquartered near Seattle. Today the company announced the completion of an $18.5 million Series B round of venture funding. It also just changed its name to Dato. CEO Carlos Guestrin gave Datanami the low down on the new name and the state of machine learning in a recent telephone briefing.
“Our goal has been to make machine learning accessible to more and more people, to allow anybody to build intelligent applications,” says Guestrin, the Carnegie Mellon professor who founded GraphLab (now Dato) in May 2013.
“We started as an academic project at Carnegie Mellon, then the University of Washington, and were initially focused on machine learning on graph data,” he continues. “But as we built the company over the last 19 months, we’ve broadened the scope after engaging a variety of customers and realizing that they have different data sets that they need to integrate.”
That realization led GraphLab to overhaul the core analytic engine with the version 1 release of GraphLab Create last July. Instead of just running machine learning algorithms atop graph data with its screaming fast graph analytic engine, Guestrin decided to expand the product’s focus, and to allow it to work atop tabular, text, and image data as well. Today the company helps customers build parallel predictive analytic applications that work with all of those types of data.
Since graph data was no longer the product’s exclusive focus, the company felt a name change was in order. Dato (the company pronounces it “dah-tow”) means “data” in Spanish and Portuguese, which form part of Guestrin’s heritage. “Over the last month or so we really put a lot of effort into thinking about what kind of company we wanted to be and how we want to be known,” he says. “When we got to the name Dato, it was just great. It’s short and beautiful. It also has a great meaning….”
That doesn’t mean Dato’s product, which is still called GraphLab Create for the time being, won’t work on graph data, such as social media data. The highly scalable, parallel machine learning processes that the software implements can deliver results with latency measured in the millisecond range.
“We’re two to four orders of magnitude faster than anything out there. We’re still the highest performing system when people compare” the top graph analytics products,” Guestrin says. Today we have a very powerful platform that allows folks with tabular data, graph data, text, and image data to be able to combine those, analyze them, and build and deploy intelligent applications.”
Machine Learning on the Rise
The future of machine learning looks quite bright, particularly for those people that can mask the underlying complexity and make it easier for developers create what Guestrin likes to call “intelligent applications,” but which others may simply call predictive analytics. “You’re going to see in 2015, in our opinion, as the year where intelligent applications really take hold,” Guestrin says. “I’ve been working with this for 20 years. For me it’s really super fun.”
Guestrin, who authored the seminal 2009 paper on GraphLab, says the technology is advancing to the point where any company will be able to build the types of intelligent, predictive applications that Web giants like Google, Netflix, and Amazon have built into their services. That day is not yet here, but it will be soon, he says.
“They had a really big talent hole they had to fill to be able build [those predictive applications] in a robust and scalable way,” he says of the Web giants. “This type of tool is really not broadly available today….So the question is, how can we make the technology that typically requires a big engineering team or multiple engineering teams to deploy this successfully, available in a simple way for a broader audience?”
Dato has already racked up several big wins with its commercial product, which is largely based on the open source GraphLab project but contains additional algorithms not available via the Apache license, as well as the automated algorithm selection routine and deployment capabilities. Zillow, Paypal, Adobe, Pandora, and Exxon are listed as customers on the company’s website. Dato will not disclose the number of paying customers, but says it had 30 percent year-over-year revenue growth.
While machine learning is not an overly crowded field at the moment, there are plenty of other avenues an organization can go down to get machine learning functionality, including using the Mahout library in Hadoop, the MLLib library in Spark, and any number of proprietary toolsets from the likes of Skytree, Rapid Miner, Knime, Revolution Analytics, and Alpine Data Labs, not to mention established analytics vendors like Microsoft, IBM, and SAS.
Guestrin hopes that the ease-of-use that GraphLab Create brings to the table–both from the development and deployment standpoints–will help it stand out against the other options.
“Things like Mahout on Hadoop, power tools, or even Python–those are a great way to get started,” he says, adding that GraphLab Create works with both Hadoop and Spark. “But they require both a lot of hand tuning, a lot of expertise, and they’re typically not production ready. So what we provide is really a simple way to get started with machine learning. Even if you don’t know what the algorithm underlying those are, we take care of that for you.”
ML Algorithms Unchained
As more organizations realize what machine learning systems can do, Guestrin’s hope is they’ll find new ways to utilize the capabilities. Most of the big machine learning use cases today revolve around familiar stories, such as building recommender systems, targeted advertising, fraud detection, and financial modeling. But down the road, all sorts of possibilities will open up.
“What’s exciting to me is this is all about creativity,” Guestrin’s says. “It’s not about technology, but the doors that it opens. If we can make machine learning accessible to more people, I can’t even imagine what kinds of cool new apps people will come out with.”
Guestrin compares what’s about to happen in the field of machine learning to what occurred with the introduction of the Apple iPhone in 2007. “I think the iOS is the big innovation” compared to the phone itself, he says. “It enables software engineers to come up with a cool phone app and then distribute it in a way that has a tremendous amount of impact. So it really unleashed a wave of creativity on the phone that is tremendous. And to be able to do the same for intelligent applications will really be transformative to us.”
The $18.5 million Series B funding round includes previous investors NEA and Madrona Ventures, as well as new investors Vulcan Capital and Opus Capital Ventures. The round brings the Bellevue, Washington company’s total venture funding to $25.25 million. The funding will allow the 37-person company, which took home third place in last falls’ Strata Startup Showcase, to continue product development and scale the operation to meet new demand.
Related Items:
Top Big Data Startups Honored at Strata
GraphLabs Wises Up Machine Learning Platform
Inside Sibyl, Google’s Massively Parallel Machine Learning Platform