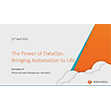
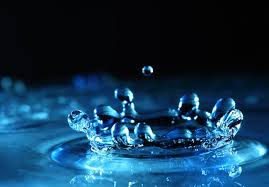
The prospect of storing and processing all of one’s data in an enterprise data lake running on Hadoop is gaining momentum, particularly when it comes to today’s massive unstructured data flows. However, given what we know of technological evolution and human nature itself, the chance of eliminating data silos and centralizing storage and compute is slim this big-data age.
Data lakes make a lot of sense conceptually. Instead of allowing silos to perpetuate, an organization pools all of its resources together into a giant shared repository for structured, semi-structured, and unstructured data. When data gets to a certain size, just moving it becomes a burden. It’s better to keep it all in one place where it can be managed, secured, and made available to users in a controlled and predictable manner.
But as attractive as the data lake proposition is, the whole thing melts down upon closer inspection. Yes, Hadoop’s rapid evolution is lowering the barriers of entry to the types of scale-out systems that companies like Google and Facebook use to run their businesses. The Hadoop stack is the embodiment of decade’s worth of data science and represents the future of big data analytics.
However, just as technological barriers have lowered and big storage needs have skyrocketed for entire companies and organizations, these same dynamics are occurring for individual departments and groups. And therein lies the dilemma. What makes sense for the company or organization also makes sense for smaller groups within.
Big corporations have been working to solve the problem of the proliferation of data silos and the lack of unified master data management (MDM) for the past 30 years. So why would one think they’ve now been sufficiently solved to the point where it’s feasible to build a single all-encompassing data lake that serves the entire company?
Gartner dismissed the data lake concept with a report earlier this year that suggested users beware of the “data lake fallacy.” “The need for increased agility and accessibility for data analysis is the primary driver for data lakes,” said Andrew White, vice president and distinguished analyst at Gartner. “Nevertheless, while it is certainly true that data lakes can provide value to various parts of the organization, the proposition of enterprise-wide data management has yet to be realized.”
In lieu of the master data management (MDM) silver bullet, companies and organizations will continue to collect and analyze data in the same ad-hoc manner they’ve been doing all their lives, says Theo Vassilakis, the co-founder and CEO of Metanautix and one of the developers of Google’s Dremel, the distributed query engine that powers Google’s BigQuery.
“The message isn’t so much there won’t be lakes, but much more that each area [of the business] will want their own, just as they wanted their own warehouse and they wanted their own marts and things of that nature,” Vassilakis tells Datanami in an interview.
The big data boom is much more than Hadoop, and is a force that’s powering the burgeoning data economy. The more creative ways that groups and individuals can come up with to generate and consume data, the more successful that group or company is going to be in this emerging data economy.
“So many more parts of a business are creating data now,” Vassilakis says. “That can be as simple as making spreadsheets online or fielding polls on Survyemonkey or using a SaaS app or developing their own mobile apps. Even getting the sense of all the data that’s been generated in the enterprise is hard and it’s accelerating.”
But it would be a mistake to assume that all this data-centric work is going to be scripted or orchestrated from the top down in an organized and controllable manner. Instead, each group is going to lurch forward in haphazard fashion, following the mantra of continuous iterative development and “fail fast” that’s burned into the new data-centric economy.
The way Vassilakis sees it, all of this new data analysis work is not going to happen in a single data lake, and it’s not going to happen in just Hadoop. Sure Hadoop will be involved, but it’s also going to involve DB2 and mainframes and Oracle and Teradata and Google Analytics and Salesforce and wherever else the data resides.
“The analyst is going to want to join that piece of data they made with whatever their established thing is,” he says. “Are you now prepared to do the work of pulling it into a Hadoop cluster to combine it with the other data?…Our view of the dynamic is, chances are you’re probably not. If you have an easy way to access that bit of data, your path will be that.”
That decentralized data architecture was the norm at Google, where Vassilakis worked, and at Facebook, where Metanautix other co-founder, Toli Lerios, worked. The notion of developing a single centralized data lake is at odds with the practical boundaries and momentum of the workplace.
This tension is evident with SaaS vendors, such as Google Analytics. Google is more than happy to help you analyze your website traffic, provided you analyze the data in Google’s application. “It’s your data. You own it,” Vassilakis says. ” But you can’t download it. There’s no immediate provision to download it. Google will be happy to help you push it into Google Compute Storage and use Google BigQuery to analyze it. It’s a way to build on the competitive value you have by giving people a richer way to interact with the data you helped them create.”
Eventually that Google Analytics data needs to meet other data to get the highest value from of it. So either the company uploads other data into Google’s cloud, or Google lets the customer download some data to their premises. Or–more than likely—it’s all of the above.
“The dynamics between that SaaS provider and the customers are comparable to the dynamics of different divisions of a corporation and its EDW,” Vassilakis says. “That story will play out again and again because it’s the same actors, but in different roles. And how it ended last time was there was no central EDW. There were different data marts. There was some level of centralization for some kinds of things, and there was some level of fragmentation as well, and each business tolerated some different factor of those two endpoints, depending on how they needed to operate.”
Analysts will take the path of least resistance when merging data from different lakes or silos, and that’s where Metanautix’s new SQL-based tool, called Quest, comes in. The software functions a bit like a distributed ETL tool, and enables analysts to grab and merge the data they need when they need it. Quest is built atop a column-oriented database, but it doesn’t store any data beyond what it needs to cache to execute its SQL queries, and it will run wherever there are Java-compatible resources, be it Hadoop, an EDW, or even a mainframe.
Quest is able to gather and join data from thousands of machines. “One of the demos we do for people is we show them instances of Quest running in different places,” Vassilakis says. “We’ll show 1,000 clusters on AWS and 10 machines in our office and 100 machines on something else. Then we’ll run a single query that goes and hits each of those three clusters.”
In the real world, each of those three clusters might be run by different organizations. “And those organizations might not be prepared to give you their data outright maybe because they don’t want to or their polices or security requirements don’t let them,” Vassilakis continues. “But maybe they’re willing to let you run queries from time to time, provided they can see what the query is and can control how often you’re running it and they can block them and log them and be able to tell which queries you ran. We think these scenarios are going to be a lot more pervasive.”
Real life is messy and rarely unfolds the way we script it. That’s not to say we shouldn’t try to improve upon the past. But when it comes to the way we create and store and analyze data, don’t hold your breath waiting for a big data lake to solve your problems.
Related Items:
The Aspirational Data Lake Value Proposition
Dremel Builder Gets $7M for SQL-Based Supertool
May 7, 2024
- Datadog Launches IT Event Management to Enhance AIOps Capabilities
- Panasas Rebrands as VDURA: A New Chapter in Software-Defined Storage for AI Applications
- Niobium Secures $5.5M in Venture Financing to Commercialize FHE Accelerator Chip
- Oracle Code Assist Can Help Developers Build Applications Faster with AI
- Redpanda 24.1 Launches with New Write Caching Capabilities for 98% Improved Latency
- Persistent Transforms Enterprise Data Management with iAURA, a Portfolio of AI-Powered Data Solutions
- BigID Launches Industry-First Hybrid Scanning for Cloud Native Workloads
May 6, 2024
- Cisco and Splunk Integrate XDR and Enterprise Security for Advanced Threat Protection
- Normalyze Innovates to Secure LLMs and Data Lakes Against Emerging Threats
- Couchbase Survey Finds Enterprises Plan Massive Spend on AI, with Over $21M Allocated in 2023-24
- Elastic Enhances Security Operations with AI-Assisted Attack Discovery and Analysis
- UCSD Researchers Develop New Framework to Curb Undesirable AI Outputs
May 3, 2024
- IDC: Structured Database Workloads Drive Major IT Infrastructure Spending in Late 2023
- Oracle Database 23ai Brings the Power of AI to Enterprise Data and Applications
- Solodev Debuts Advanced AI Services to Boost Cloud-Based App Development
- Sigma Announces AI Toolkit for Business and Launches Sigma Actions to Support Custom Data Applications
- New Salesforce White Paper Tackles LLM Security Risks
May 2, 2024
Most Read Features
Sorry. No data so far.
Most Read News In Brief
Sorry. No data so far.
Most Read This Just In
Sorry. No data so far.
Sponsored Partner Content
-
Get your Data AI Ready – Celebrate One Year of Deep Dish Data Virtual Series!
-
Supercharge Your Data Lake with Spark 3.3
-
Learn How to Build a Custom Chatbot Using a RAG Workflow in Minutes [Hands-on Demo]
-
Overcome ETL Bottlenecks with Metadata-driven Integration for the AI Era [Free Guide]
-
Gartner® Hype Cycle™ for Analytics and Business Intelligence 2023
-
The Art of Mastering Data Quality for AI and Analytics
Sponsored Whitepapers
Contributors
Featured Events
-
AI & Big Data Expo North America 2024
June 5 - June 6Santa Clara CA United States -
CDAO Canada Public Sector 2024
June 18 - June 19 -
AI Hardware & Edge AI Summit Europe
June 18 - June 19London United Kingdom -
AI Hardware & Edge AI Summit 2024
September 10 - September 12San Jose CA United States -
CDAO Government 2024
September 18 - September 19Washington DC United States