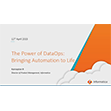
Big Data Gets Green Light for Traffic Management
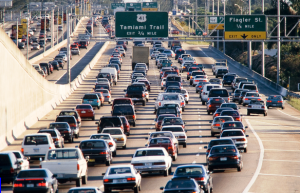
Traffic management in the United States often focuses on maximizing limited capacity. For example, a truck breaks down in the center lane during the morning rush hour. For the drivers behind the broken down truck, traffic capacity on a three-lane expressway just dropped by at least one-third.
It is unlikely that traffic capacity will increase in the United States since most jurisdictions are having a hard time finding the money to maintain existing roads, bridges and tunnels. Adding signaling smarts in congested downtown areas can help, but states and municipalities are now starting to turn to sensor networks and other data collectors as a way to get a handle on metrics like average vehicle speed, sources of acceleration and slow downs, weather conditions and other factors that can make commuting a living hell.
Big data specialists like Apache Hadoop distributor MapR are trumpeting the potential of data tools to make sense of the growing datasets related to transportation management. While traffic management remains the leading application, MapR stressed in a recent blog post that it is also being used to manage rail traffic, long-haul trucking and delivery, even road repair.
Moreover, the volume and “velocity” of traffic data is growing as the networking of devices—the Internet of Things—jumps on the entrance ramp in large metropolitan areas. These networks are collecting sensor data from devices ranging from radio-frequency identification tags to embedded road sensors.
Since everyone complains about traffic but little is done about it, traffic management has emerged as a key application for data analytics. MapR sited a use case in New Jersey where 22-foot-tall “sensor screens” collect cellphone and GPS signals from passing commuters. That traffic data is correlated to create a 2,600-mile live-data traffic map of the state’s highways.
Before New Jersey officials began using traffic data, a rush-hour accident would have tied up traffic for hours. MapR claims state highway officials can use the real-time data to dispatch emergency vehicles to clear highways within 30 minutes.
Commuter rail systems are also said to be integrating big data tools like Hadoop into the operations. These systems can collect data from GPS units to record train speed, distance between trains and projected arrival times. Other railway data are collected by acoustic sensors on train brakes, rails and switches.
One unidentified railway operator is said to have recently invested in an automated system that uses big data to manage the rescheduling of more than 8,000 trains, MapR said.
Trucking companies were one of the earliest adopters of tools like predictive modeling to select fuel-efficient trucks and find the most direct shipping routes. These models are being used to make multimillion dollar decisions about what trucks to add to a fleet as well as how to shave a mile per day per driver in shipping costs. A one-mile reduction per day can sometimes translate into fuel savings as high as $50 million a year, big data proponents estimate.
Meanwhile, northern U.S. cities with two seasons (winter and road repair) are trying to leverage big data to prioritize repair operations. In a Boston trial, drivers can use an app known as “Street Bump” to detect the unmistakable jolt of a pothole. This data, rather than road surveys by traffic engineers, can be used to identify roads in greatest need of repair.
Telecommuting remains one of the best ways to avoid the hated morning commute. As highway maintenance budgets are slashed, the solutions being promoted by big data vendors represent another alternative to keeping the nation’s rail and commuter traffic moving and its surface distribution network humming.
Recent items:
Big Data Helps Drive Transportation Planning