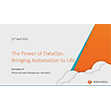
Coping with Big Data at Experian – “Don’t Wait, Don’t Stop”
In conjunction with MapR, Datanami presents Experian with this month’s “Big Data All Star” award.
Experian is no stranger to Big Data. The company can trace its origins back to 1803 when a group of London merchants began swapping information on customers who had failed to meet their debts.
Fast forward 211 years. The rapid growth of the credit reference industry and the market for credit risk management services set the stage for the reliance on increasing amounts of consumer and business data that has culminated in an explosion of Big Data. Data that is Experian’s life’s blood.
With global revenues of $4.8 billion ($2.4 billion in North America and 16,000 employees worldwide (6,000 in North America), Experian is an international information services organization working with a majority of the world’s largest companies. It has four primary business lines: credit services, decision analytics, direct-to-consumer products, and a marketing services group.
Tom Thomas is the director of the Data Development Technology Group within the Consumer Services Division. “Our group provides production operations support as well as technology solutions for our various business units including Automotive, Business, Collections, Consumer, Fraud, and various Data Lab joint-development initiatives,” he explains. “I work closely with Norbert Frohlich and Dave Garnier, our lead developers. They are responsible for the design and development of our various solutions, including those that leverage MapR Hadoop environments.”
Until recently, the Group had been getting by, as Thomas puts it “…with solutions running on a couple of Windows servers and a SAN.” But as the company added new products and new sets of data quality rules, more data had to be processed in the same or less time. It was time to upgrade. But simply adding to the existing Windows/SAN system wasn’t an option – too cumbersome and expensive.
So the group upgraded to a Linux-based HPC cluster with – for the time being – six nodes. Says Thomas, “We have a single customer solution right now. But as we get new customers who can use this kind of capability, we can add additional nodes and storage and processing capacity at the same time.”
“All our solutions leverage MapR NFS functionality,” he continues. “This allows us to transition from our previous internal or SAN storage to Hadoop by mounting the cluster directly. In turn, this provides us with access to the data via HDFS and Hadoop environment tools, such as Hive.”
ETL tools like DMX-h from Syncsort also figured prominently in the new infrastructure, as does MapR NFS. MapR is the only distribution for Apache Hadoop that leverages the full power of the NFS protocol for remote access to shared disks across the network.
“Our first solution includes well-known and defined metrics and aggregations,” Thomas says. “We leverage DMX-h to determine metrics for each record and pre-aggregate other metrics, which are then stored in Hadoop to be used in downstream analytics as well as real-time rules based actions. Our second solution follows a traditional data operations flow, except in this case we use DMX-h to prepare in-bound source data that is then stored in MapR Hadoop. Then we run Experian-proprietary models that read the data via Hive and create client-specific and industry-unique results.
“Our latest endeavor copies data files from a legacy dual application server and SAN product solution to a MapR Hadoop cluster quite easily as facilitated by the MapR NFS functionality,” Thomas continues. “The files are then available for analysts to query with SQL via Hive – without the need to build and load a structured database. Since we are just starting to work with this data, we are not ‘stuck’ with that initial database schema that we would have developed, and thus eliminated that rework time. Our analysts have Tableau and DMX-h available to them, and will generate our initial reports and any analytics data files. Once the useful data, reports, and results formats are firmed up, we will work on optimizing production.”
Developers Garnier and Frohlich point out that by taking advantage of the Hadoop cluster, the team was able to realize substantial more processing power and storage space, without the costs associated with traditional blade servers equipped with SAN storage. Two of the servers from the cluster are also application servers running SmartLoad code and components. The result is a more efficient use of hardware with no need for separate servers to run the application.
Here’s how Thomas summarizes the benefits of the upgraded system to both the company and its customers: “We are realizing increased processing speed which leads to shorter delivery times. In addition, reduced storage expenses means that we can store more, not acquire less. Both the company’s internal operations and our clients have access to deeper data supporting and aiding insights into their business areas.
“Overall, we are seeing reduced storage expenses while gaining processing and store capabilities and capacities,” he adds. “This translates into an improved speed to market for our business units. It also positions our Group to grow our Hadoop ecosystem to meet future Big Data requirements.”
And when it comes to being a Big Data All Star in today’s information-intensive world, Thomas’ advice is short and to the point: “Don’t wait and don’t stop.”