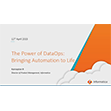
Here’s Another Option for Hadoop Enterprise Search
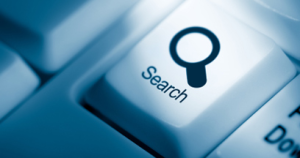
The software stacks of many Hadoop distributions feature Apache Lucene and Solr as the enterprise search component. But the folks at the French firm Sinequa say Hadoop customers will get more actual work done–and quickly analyze massive amounts of poly-structured data from dozens of other sources in multiple languages–by using its enterprise search solution.
Hadoop, machine learning algorithms, and graph databases may get most of the headlines in our big data world, but good old search engines continue to be popular because they’re relatively easy to use for non-technical users. You don’t need to be an expert in SQL or a MapReduce pro to query your data store. Instead, a basic grasp of your chosen language and data destination will suffice.
But today’s search engines are far more sophisticated than Google’s Web search, and are able to connect data from multiple locations in ways that may surpass traditional notions of what search engines can do. In the case of Sinequa, which was born out of a linguistics project that started 20 years ago, the ability to detect the meaning and associations behind words opens up powerful use cases in the area of content analytics.
Sinequa is particularly strong in the field of natural language processing (NLP) and the capability to deduce meaning from compound words and phrases in a variety of languages. CEO Alexandre Bilger, who spun the research project out into a commercial entity about 10 years ago, says his product’s capability to analyze dozens of languages adds a level richness to the core underlying index that is not found in competing solutions.
“Compound words [like part numbers and genetic expressions] are very hard to deal with in a structured manner,” Bilger tells Datanami. “This richness was in our DNA very early on. NLP is not easy to build, even if you have lots of developers. From scratch, it takes time to do that. And we have been doing that for a long time.”
Today Sinequa has 250 customers for its flagship Sinequa ES product, including big names like Astra Zeneca, Siemens, and Airbus. The Astra Zeneca implementation is especially noteworthy because it’s enabling drug researchers, scientists, and managers at the $25-billion UK pharmaceutical company to query their resources and make connections that would otherwise be very difficult to obtain.
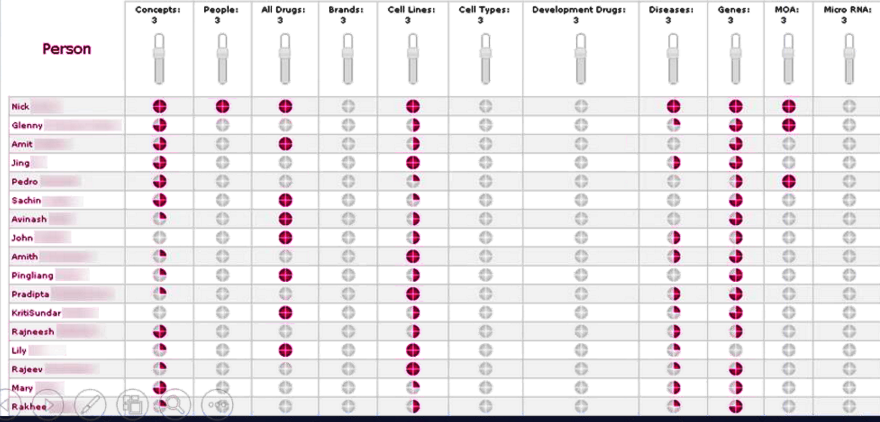
Astra Zeneca users can dial in their search settings to find experts in given pharmacological fields
Sinequa’s solution for Astra Zeneca enables users to quickly identify who are the preeminent experts in any given pharmacological field goal. More than 15,000 AZ are able to tweak the search settings by dialing up weighting factors for genes, drugs, diseases, and delivery mechanisms. The system generates its answers by pulling data out of a search repository of more than 200 million documents spread across internal SharePoint, Documentum, and Office 365 systems and various external medical database, including MEDLINE, EMBASE, and Scopus.
According to Sinequa’s vice president of sales Xavier Pornain, the software’s capability to identify and isolate people’s names among all the other pieces of textual data is critical component in its work at Astra Zeneca. “We were able to map all the documents that contain a given drug to the people who were actually cited in the document,” Pornain says. “We’re able to map the expertise that’s reflected in the 200 million documents.”
Sub-second response time for Sinequa queries gives the Astra Zeneca users the confidence to explore the facts and relationships as represented in the data, without getting bogged down by the hazy filter of human perception. “We call it an inverted social network,” Pornain says. “It’s actually the opposite of an enterprise social network, where people declare their expertise. Here we start with the documents, and we surface the expertise of the people.”
In June the company bolstered its big data bona fides with the unveiling of Sinequa ES version 9, which enables the product to search and analyze data stored in the Hadoop Distributed File System (HDFS). V9 also added a handler for Mahout, which allows the search engine to leverage the machine learning library to perform automatic classification and clustering, recommendations, and predictive analysis from applications developed in Sinequa ES. These give another layer of analytical capabilities on top of its own NLP algorithms.
Bilger sees enterprise search engines occupying one leg of an emerging big data stack that has Hadoop and NoSQL databases like MongoDB occupying the other two legs (the company offers a MongoDB connector among its collection of 140 connectors). “We think the best repository possible for text or textual data is search and a search index,” he says. “And also when you deal with that, you need content analytics because you cannot build applications without structure. So you have to create this structure, and that’s the space where we are positioning ourselves.”
Sinequa’s combination of the search index and content analytics appears to be working well for the French company, which generated about $40 million in revenue last year with just 50 employees, according to Bilgr. It’s certainly on the radar screen for Gartner, which gave Sinequa a promising rating in its 2014 Magic Quadrant for Enterprise Search, placing the French firm just in the “Visionaries” quadrant, just on the cusp of the “Leaders” box. The analyst firm lauded Sinequa for its ability to search and analyze structured and unstructured data in a hybrid manner, and for the way it integrates taxonomies, ontologies, and vocabularies into its semantic reasoning engine.
The company’s big drawback, according to Gartner’s analysis, is the limited reach of the firm outside of French-speaking countries. The company is aware of that shortcoming, and is working to build a bigger presence in the United States. To that end, Bilgr and Pornain recently completed a two-week trip to New York City, where the company maintains an office.
Sinequa is hoping that the relative ease of developing search-based applications and the interactive nature of search helps it gain traction at enterprises struggling with big data. To that end, it’s also working in the telecommunications field, where the capability to query massive amounts of data stored in multiple operational systems (like call data records, equipment logs, CRM systems, databases, and other applications) may provide a good source of targeted information for customer service reps in call centers, where cutting a four-minute call by 30 seconds translates into tens of millions of dollars of savings per year.
Sinequa appears poised to capture its share of the enterprise search market, which Gartner sees growing from $1.7 billion in 2013 to $2.6 billion by 2017, a compound growth rate of 11.2 percent. But ultimately, the vendor’s largest challenge may not be bigger competitors or a French accent, but the availability of open source products like Lucene and Solr. Today, Sinequa may holds a functional lead over the open source offerings, which Bilger characterizes as more do-it-yourself projects compared that require development skill. But it will need to continue that R&D effort, and probably build out its channel and possibly its partner network, to grow its share of the market.
Related Items:
Searching Big Data’s Open Source Roots
Splunk Gets ‘Lucid’ About Solr-Powered Search
Hadoop Distros Orbit Around Solr