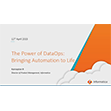
Are Data Lakes All Wet?
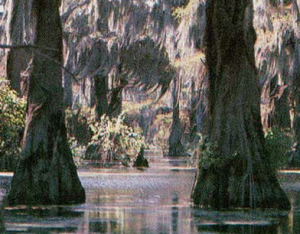
Enterprise data management platforms known as “data lakes” are being promoted as, among other things, a potential solution to “information siloes” by combining different managed collections of data in an unmanaged data lake.
The theory is that data consolidation will increase use and sharing of information while reducing storage and server costs. However, a new market study dismisses most of those claims as a “fallacy,” arguing instead that enterprises still require secure data repositories, in other words, data warehouses.
At the same time, an analysis by market researcher Gartner notes that data lakes seek to overcome big data issues related to the volumes of information required. The approach also addresses questions like the variety and type of information being analyzed and whether storing it in a structured data warehouse or database constrains future analysis.
This approach could provide short-term IT benefits since data is simply dumped into a data lake. But without some type of “information governance,” warns Gartner analyst Andrew White, the data lake “will end up being a collection of disconnected data pools or information silos all in one place.”
Hence, Gartner concludes, the gaps in the data lake model are generating confusion among information managers about precisely what the storage approach can and cannot offer and whether it represents an enterprise-wide big data solution.
Gartner concludes that data lakes, unlike traditional data warehouses, “carry substantial risks.”
One reason is that promoters of data lake technology assume most if not all potential customers are skilled at data management and analysis. Still, embattled IT managers are looking for increased agility and accessibility to data in order to boost performance and speed up data analysis.
Gartner’s White remains skeptical: “While it is certainly true that data lakes can provide value to various parts of the organization, the proposition of enterprise-wide data management has yet to be realized,” he stressed in a report released in late July.
A major flaw in the data lake approach is its inability to determine data quality or track the findings of others who have found value in data. Part of the problem is that data lakes by definition accept any data.
“Without descriptive metadata and a mechanism to maintain it, the data lake risks turning into a data swamp,” Gartner concludes. “And without metadata, every subsequent use of data means analysts start from scratch.”
These risks bring with them further headaches in the form of security and access control. Gartner analysts argued that most data lakes are filling up with data whose privacy and regularity requirements are unknown.
Nevertheless, promoters of “schema-less SQL” approaches like Hadapt argue that data handling tools could eventually be absorbed within a Hadoop-based data lake. While some see a data warehouse on the shore of a future data lake, Hadapt argued in a blog post before it was acquired by analytic data platform vendor Teradata that “data warehouse needs [will be] subsumed with Hadoop using Hadapt’s Flexible Schema to address semi-structured data with SQL.”
Gartner dismissed these claims as part of the “growing hype surrounding data lakes.” Data lakes “typically begin as ungoverned data stores,” added Nick Heudecker, Gartner’s research director. “Meeting the needs of wider audiences require curated repositories with governance, semantic consistency and access controls — elements already found in a data warehouse.
Which seems to be the point of the Gartner study: You get what you pay for. And while data lakes are cheaper, the risks in terms of data quality and security may in the case of big data projects outweigh the benefits of a catchall data storage solution.
Ultimately, the big data market will decide. For now, companies like Teradata, which acquired Hadapt in July, are betting their own money that there’s something to the data lake approach.
Related items:
Teradata Acquires Revelytix, Hadapt
Hadapt Aims at Untangling ETL with Schemaless SQL