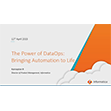
A Prediction Machine Powered by Big Social Data
What if you had a machine that could predict what people will be talking about on the Internet over the next three days. How would you use that information? The folks at Blab say they have created such a machine, and the way its customers are using it might surprise you.
There is a veritable gold rush occurring on the Web, as entrepreneurs and technologists set out to mine meaningful insights from big, messy social media data. Most of these attempts fail, largely because approaches that may work in the lab don’t work in real time and at Internet scale. But that doesn’t stop the startups from coming, because there are billions at stake for the outfit that gets it right.
One of those startups vying to do for dynamic social data what Google’s search engine did for static Web data is Blab, a 14-person tech firm based in Seattle, Washington. Blab founder and CEO Randy Browning tells Datanami that his company has solved the puzzle and come up with a novel way to monitor the world’s online conversations and then make accurate predictions about what people will be talking about for the next three days.
According to Browning, Blab continuously monitors all the public conversations that people are having across 50,000 different websites and social media properties. The company ingests 100 million posts per day occurring across all the world’s major news sites, Facebook, Twitter, Tumblr, YouTube, Flickr, Redit, and tens of thousands of blogs. Blab can not only pull in text in any language, but pictures, video, and audio clips too. So if you communicate primarily via emoticon or captioned cat pictures, Blab has you covered.
Customers pay Blab $3,000 a month for the privilege to “seed” 15 topics into its machine and view Blab’s predictions because it gives them something they can’t get elsewhere: Control.
“The value proposition this gives to customers is it gives them back control in today’s world of real-time social media,” Browning says. “Companies are not comfortable deploying their ad budgets in real time because they lose control.”
Most of Blab’s customers today are advertising and PR agencies that are looking to ride social media’s waves on behalf of their customers–or at least to avoid getting wiped out by them. “We give our customers a heads up of where the conversation is going, what will resonate, when it will resonate, and where it will resonate,” says Browning, who spent 10 years in the advertising business. “Then they’re able to get ahead of that, create relevant content, and optimize and time shift their media plans so that they can be most effective.”
Blab’s Data Science
The Blab machine works similarly to Pandora, the Internet radio station. Instead of looking at the content of news stories hashtags that are trending on Twitter, the company reduces the real-time conversations people are having into metadata, such as source, time, influencer, length of post, keywords, and tags. Trying to track conversations by their content, it turns out, is a lousy way to go about this; the natural language processing (NLP) algorithms are simply too finicky.
![]() Blab’s Web-based console allows users to see how topics will trend on different websites and social media outlets |
|
“If it was heavy mathematical equations, it would not be possible to do what we do,” Browning says. “Hadoop doesn’t work for us given the amount of real-time analytics we need to do. We can’t use NLP libraries. We can’t use traditional data science approach to predictions. We’ve had to really create a new way of going about it.”
As the world’s conversations occur, Blab uses public APIs to capture them. The company stores the conversations temporarily in a MongoDB database before they’re transformed into metadata that’s streamed it into its real-time predictive engine, which is based on proprietary algorithms that the company doesn’t discuss publicly. All of this occurs on Amazon’s cloud infrastructure, which Browning says Blab taxes like no other tenant, including Netflix.
Ingesting and normalizing such vast amounts of data is the hard part, and the part that eats the most processor cycles and GBs of memory at AWS. Once the world’s conversations are reduced to metadata, the data sizes are manageable: in the hundreds of GB range.
Once in metadata format, the conversations are compared to a historical database of conversations that Blab keeps up-to-date using machine learning algorithms. By comparing the new conversations to the historical model, Blab can say, with an average accuracy of 70 percent, what will happen to those conversations over the next three days. Will they go viral? If so, where will they go viral, and when? Or will the topics of conversation flame out and return to previous topics and pictures of cats saying clever things?
While conversation topics may appear to heat up and cool down almost randomly, there are actually patterns in the noise that can be useful for making predictions, explains Ben Bressler, Blab’s director of product management.
“What we found is that conversations go viral in the same way regardless of topic,” Bressler says. “Whether it’s Justin Bieber’s new single or war crimes in Syria, the way something goes viral online, whether it grows or shrinks or goes from this channel to that channel, is very similar regardless of topic.”
![]() |
|
Blab uses a variation of the word cloud graphic to help users see how topics are trending |
This phenomenon was demonstrated recently by a Blab customer, one of the world’s largest technology companies, Browning says. A small group of people in Italy and Germany started talking about how the latest release of the company’s software was causing tablets to crash. Nobody in the U.S. was talking about this particular problem, but based on the influence of those Italians and Germans and other aspects of the conversation, Blab predicted, with an 85 percent confidence rating, that this story was going to “break” in the U.S. in about 40 hours.
“We were giving our client a 40 hour heads up that this story is going to break,” Browning said. “This gave them time for the product team to put a patch in place, and their customer service and support teams to work efficiently. They wouldn’t have to man the phone until hour 39, and their marketers could start to mitigate the issues as they’re coming down the pipe. They weren’t even looking for the conversation, and they discovered it.”
Predicting Success
Nobody knows how Blab’s story will turn out. The company has attracted a handful of early customers, including Horizon Media, the largest independent media planning and ad buying agency in the U.S. Horizon has also invested in Blab, which has accumulated a total of $3.5 in venture capital.
For now, Blab is concentering on giving companies the capability to meld their advertising to the changing zeitgeist of the Internet. That’s something they haven’t had before, says Taylor Valentine, SVP of social media and relationship marketing at Horizon Media. “The ability to make real-time media inventory purchases on-the-fly, and in a meaningful way really flips the model on how to engage customers, and makes reactive marketing a thing of the past,” Valentine says in a statement.
Later this week, Blab will announced version 2 of its platform, which brings UI enhancements and new capabilities to filter terms they are seeding into the Blab engine. Blab is currently generating about 1 million predictions per minute for its users, and it’s up to them to be able to spot the predictions that matter to them. “It allows people to find that non-obvious conversation quickly, to get into the conversation, and spend more time creating and working, rather than spending the majority of time finding what they should be acting on,” Bressler says.
If you’re wondering why, if Blab’s prediction machine is so accurate, the company has not taking it straight to Wall Street to make a killing, that’s because the technology is so new that they are first trying it out on social media data. “Arguably social data is probably the messiest and most chaotic so it was great to cut our teeth on one of the hardest problems to solve,” Browning says. “There are multiple streams we’re going to be looking at. Financial is another one.”
Related Items:
Faceboook Gets Smarter with Graph Engine Optimization
Saffron Gets $7M to Build Brain-Like Learning Machine
Will Your Startup Succeed? Ask an Algorithm