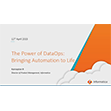
Finding Value in Data Through Life Saving Applications
Big data is not about big; it’s not even about data…It’s about the value that can be found in the information, argued CTO of Fujitsu Technology Solutions, Joseph Reger, during a talk at the International Supercomputing Conference (ISC’13) this week. Taking the stage with his colleague, Kozo Otsuka, the pair discussed some of the experimental applications that Fujitsu is working on in trying to sift the value from data for the purposes of saving lives.
The chief challenge in big data, said Reger, is the discovery process in which you find the hidden value in it. Reger discussed the applications happening at Fujitsu Laboratories and Fujitsu Limited as they explore for ways to combine data from the past, present, as well as data about the possible future ( through simulation) to create new applications for public safety and disaster management/prevention.
Here is a summary of the four use cases that Reger and Otsuka presented at the conference this week…
Using Predictive Analytics for Early Disease Detection
There are some diseases, explained Reger, such as diabetes, which can be seen as lifestyle related diseases where the condition can be delayed or prevented given the right habits. According to the National Center for Biotechnology Information, Japan is one of the nations that is most affected by diabetes with almost 15% of the population with either type 2 diabetes of impaired glucose tolerance. They report that it ends up impacting 6% of the total healthcare budget in Japan.
To help combat these trends, Fujitsu has implemented a unique, experimental health care program for a test group of employees. Otsuka explained that 26,000 of Fujitsu’s 150,000 employees in Japan have volunteered to take part in this program, a new kind of healthcare system which requires them to be active in providing data into the data pool and having it checked against it.
In order to be part of the program, the employees are asked to provide at least 5 years of their most recent healthcare records. The program participants are then asked each year to get a comprehensive health examination in which several data points are collected, including such things as HDL cholesterol levels, platelet counts, total protein levels, white blood cells, and much more – the list is extensive. Finally, the participants must provide a sampling of their vital data approximately once every six months.
After the data is collected and analyzed, Fujitsu uses the data to build predictive models that help them determine probabilities for diseases. In the example given, Fujitsu was using all of the data to create a predictive algorithm to detect diabetes probabilities. Using the individuals data profile in contrast with the aggregate models, they can determine probabilities on each person’s susceptibility to diabetes. With early detection, they can make recommendations on what the individual can do to avoid a negative outcome.
“That’s a big advantage for society, and a big portion of people are susceptible to get diabetes as they age,” said Reger. It’s easy to see how such a program has the potential to provide benefits in terms of overall costs, but provide substantial improvements to quality of life, perhaps even extending mortality rates over the long term.
NEXT – Social Sensors and a Real-Time Criminal Activity Map — >
Social Sensors and a Real-Time Criminal Activity Map
There are over 12 million people in the Tokyo metropolitan area, which calculates into over 14,000 people per square kilometer in some places. With such small personal space, there is a higher risk for human conflicts, explained Otsuka, and yes, crime.
Wondering if there is a way to inform people about area crime hotspots so that they might avoid them, the researchers at Fujitsu turned to social media. Otsuka says that there are over 6 million people in the Tokyo metro area using mobile phone technology – with many of them using Twitter. According to Otsuka, there are over 40 million tweets per day in Japan.
Hidden in these tweets is a treasure trove of information about regional happenings, Otsuka explained. Using the people of Japan as “sensors” in a distributed network, Fujitsu has created an algorithm which synthesizes this information into detected events that they are able to map to geographic locations. Tweets are filtered and selected for larger topics, correlated to crime types, times and locations. This data is then overlaid onto a map in which regions are color coded to create a real time map of crime hot spots in the region. In much the way people can use a weather forecast to predict rain and plan accordingly, people can use this real time crime forecasting map to avoid trouble areas.
Reger pointed out that keywords play a big part in the machine learning semantic analysis, but also contextual clues about when and where things might happen to forecast a hot spot in advance.
While the traditional big data use cases around twitter generally focus on customer behavior analytics, this example shows that there are other ways that value can be brought out of social media. The next application works similarly, but instead of other people being the culprits, Mother Nature is the offender.
NEXT – Using Social Data to Save Lives During Climate Disasters — >
Using Social Data to Save Lives During Climate Disasters
The rigors of nature are alive and well in Japan. There are two major ocean currents that affect the country, one being the warm Kuroshio Current, and the cold Oyashio Current. The interplay between these two currents and other geographic factors turns the mountainous island into a generally rainy place where torrential rans, typhoons, and flash flooding are not so uncommon.
According to Otsuka, over 4 billion dollars of property damage occurs annually in Japan due to these factors. The combination of Japan’s population density with the dangers of torrential rains, flash floods, and landslides is a dangerous one to both life and property. And it’s a problem that is only getting worse, said Otsuka, saying that the precipitation figures increase every year. Recognizing that there is a strong need for an early warning and prevention system, Fujitsu is working to create just a system leveraging XRAIN radar and social media.
To help combat the dangers and better react to disasters, Japan has invested in a system called XRAIN, an X band radar which efficiently detects everything from rain, snow, fog, dust, and smoke. Leveraging this data, Fujitsu has taken the added step of combining it with machine learning algorithms fed by social media outlets like Twitter.
Using these algorithms, they are able to analyze a user’s disaster related post, analyze it against the user’s history of posts in order to draw a rough prediction of the area where the user spends his/her dialy life based on the landmarks mentioned in past posts. Then combined with an algorithm that scores the post in terms of reliability, social network analysis is combined with the XRAIN data in order to detect where a disaster may be happening in real time.
The end goal is a higher fidelity, real-time disaster detection system. According to Otsuka, a test run in 2012 for towns in Osaka and Kyoto prefects raised disaster alerts 3 hours earlier than conventional warnings did, with the combined data providing a much more precise analysis of location information when disasters were occurring.
NEXT – Using Big Data For Accurate Early Tsunami Warning — >
Using Big Data For Accurate Early Tsunami Warning
The sudden earthquake and subsequent tsunami wave is a very real and present danger in Japan as we were reminded recently by the March 2011 Tohoku event. Japan was hit with a waves reaching as high as 133 feet (40.5 meters) with the world bank estimating over $235 billion in damages.
While data can’t prevent a tsunami from happening, Otsuka says that they are working on ways to be able to predict the approach of a tsunami as well as its impact. This presents a serious challenge as there are no sensors to measure the amount of a fault line slide, and thus no accurate way to predict the tsunami speed or power.
While the long term goal is to have sensors on the surface and the bottom of the ocean with the use of GPS buoys, seabed wave and coastal tide gauges for accurate real-time analysis, they are taking early steps based on historical data. The first step, says Otsuka, is to develop a solid simulation algorithm based on Tohoku earthquake data. Using the historical data combined with the impressive processing power of the K computer, models are being constructed to help figure out such things as when a tsunami will hit, and with how much power based on different seismic scenarios. Otsuka says that the K computer is able to run the simulation in under 30 minutes.
Using this data, they are able to project what the initial wave probabilities look like, but also subsequent waves. They can then use this information to plan evacuation strategies that take people out of harm’s way.
This forecasting includes examining how the infrastructure of the inland behaves during a tsunami event – such as whether there is enough force to destroy a bridge, leaving people stranded. With such information in hand, officials can know where the danger hot spots are likely to be, and plan rescue strategies accordingly.
Related items:
IDC Talks Convergence in High Performance Data Analysis
HPC Analysts: Hadoop is a Tall Tree in a Broad Forest
Turning Big Data Into Information with High-Performance Analytics