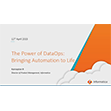
Self-Service Data Mining, Hold the Bottlenecks
Self-service data exploration by line-of-business analysts is an ideal that has been elusive in the world of big data. Whether hampered by issues with hardware or data-set tuning, business analysts often find themselves bottlenecked and caught in gyrations between the database admins and the data.
In a recent article, Platfora’s CEO, Ben Werther says that Cloudera has at least partially answered the challenge with their Impala release by allowing the business-level analyst the ability to do faster ad hoc queries on smaller data sets than had previously been possible. However, says Werther, Impala currently falls short in eliminating the bottlenecks that too often occur between the business level analyst and the DBA.
The weakness, explains Werther, is that Impala relies on what he refers to as the “Legacy Database” model, where the analyst is still heavily reliant on the DBA “to manage transformation and maintenance jobs, design and implement aggregations, tune performance, etc.” Thus the analyst is still stuck in the DBA/database gyration that can cause slowdowns for both the project, and the organization as a whole – especially in cases where complex queries on wrong tables chew up resources, and slow down every project that relies on the Hadoop cluster.
“This is not the scalable big-data architecture of the future, and it is exactly the painful world that every customer we talk to is trying to escape,” says Werther.
Werther makes the case that the Platfora platform solves this problem by taking raw data in Hadoop out of the cluster and building scale-out in-memory aggregates that users can query at will. In much the way a gold panner digs into the stream to pan for gold, the business level analyst can use Platfora to pan into Hadoop for a data set, and examine that set to their heart’s content for the nuggets of insight they’re looking for. All while freeing up the Hadoop cluster for the next data panner.
“Platfora connects in minutes to any Hadoop distribution and automatically generates MapReduce jobs to build and maintain scale-out in-memory aggregates,” explains Werther (also noting that Impala acceleration is on the roadmap). “Our scale-out middle tier is simultaneously an ‘aggregate cache’ of the data below, and a lighting fast in-memory analytical query engine to the users above.”
The theoretical end result is the elimination of the tango that happens between the analyst and the DBA, as well as the constant resource taxing on the Hadoop cluster that can slow other projects down.
Related Articles:
Cloudera Runs Real-Time with Impala
Could the Data Scientist Be a Bad Thing for Big Data?
Alteryx Aims to Bypass Data Scientists by “Humanizing” Big Data