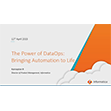
Stanford Pushes Genetic Research Data to Limits
Under the direction of Dr. Michael Snyder, Stanford’s Genetics Department is helping to push the envelope on big data and how it can advance genome research.
Snyder, a professor and chair of the university’s genetics program also directs the Stanford Center for Genomics and Personalized Medicine. This year he and his team will explore the medical benefits of their integrative personal genomics profile, or iPOP.
“Currently, we routinely measure fewer than 20 variables in a standard laboratory blood test,” says Snyder, the Stanford W. Ascherman, MD, FACS, Professor in Genetics. “We could, and should, be measuring many, many thousands. We could get a much clearer resolution of what’s going on with our health at any one point in time.”
The iPOP not only goes significantly beyond the scope of a standard blood test, but also that of the Human Genome Project. The goal is to utilize genetic information such that the diseases an individual is likely to develop are treated proactively as opposed to reactively. In order to do that, Snyder’s nucleotides are sequenced and re-sequenced 270 times over. By comparison, the Human Genome Project covered each nucleotide about eight times.
“Today’s sequencing technologies don’t sequence an entire chromosome in one swoop,” Conger writes, “they first break it into millions of short, random fragments. After each fragment is sequenced, computer algorithms assemble the small chunks into chromosome-length pieces based on bits of sequence overlap among the fragments. It’s necessary to save the raw data to closely investigate any discrepancies in critical disease-associated regions.”
Snyder and his colleagues developed RiskOGram, an algorithm that generates a risk profile for an individual based on his or her whole-genome sequence. The RiskOGram was tested on the family of entrepreneur John West before being used on Snyder’s information. As it turned out, according to RiskOGram, Snyder had a 47 percent risk of developing type-2 diabetes, more than double the usual risk of men his age.
One potentially cringe-worthy (for scientists, at least) principle behind the development of these analytics is the departure from hypothesis-based testing. Stanford’s Euan Ashley, MD, one of the developers on Snyder’s team notes, “We’ve been so focused on generating hypotheses but the availability of big data sets allows the data to speak to you. Meaningful things can pop out that you hadn’t expected. In contrast, with a hypothesis, you’re never going to be truly surprised at your result.” Snyder’s high risk of diabetes counts as a meaningful thing that can pop out. Snyder himself remarked that, “I was amused to see Type-2 diabetes emerging so strongly.”
Despite the doctor’s initial protests that the probability of his having diabetes was low, Snyder went ahead and had himself tested. The tests came back positive. The iPOP had turned up something a doctor would not have even thought to test for. It was a potentially life-saving revelation.
Of course, there are still drawbacks. Snyder sends physical hard drives to those who wish to corroborate his data since it is beyond difficult to transmit the two terabytes electronically. While the cost of these genomic workups has dropped dramatically (mapping the human genome cost approximately $300 million in 2001 while the same procedure today costs $1,000), it remains high for the layperson. Finally, and possibly most importantly, the only space in which to store more than a couple of persons’ genomes is the cloud. Storing genetic information in the cloud raises patient privacy concerns.
Currently, the Genetic Information Nondiscrimination Act of 2008 prohibits health insurance companies and employers from penalizing individuals based on genetic information. However, that protection does not extend to life insurance companies.
That being said, Snyder and his Stanford team are taking strides toward big data effecting positive change in predictive medicine.