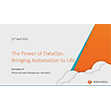
Will New Government Guidelines Spur Adoption of Privacy-Preserving Tech?
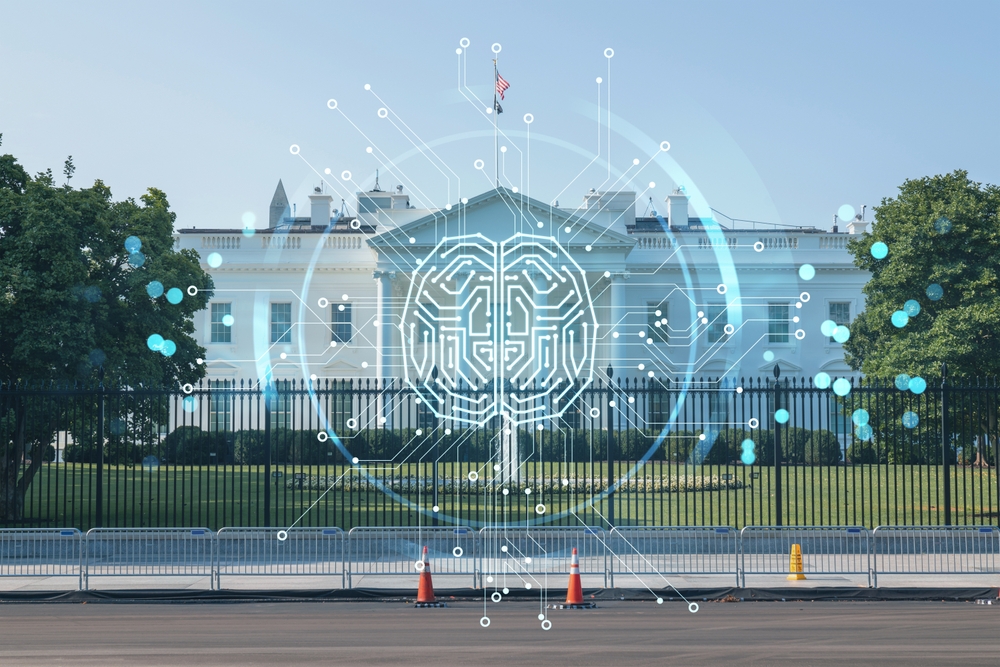
(VideoFlow/Shutterstock)
Michael Hughes, the chief business officer for Duality Technologies, could barely conceal his excitement when the White House Office of Science and Technology issued a directive calling for the adoption of privacy-enhancing technology recently. “I was just blown away,” Hughes said. “That was big.”
Up to this point, the market at large has been relatively slow to adopt privacy-preserving technologies, which allow sensitive data to be used for analytics or AI without sacrificing privacy. Hughes expects that to change soon in light of several developments, including a potential new US law dubbed the American Privacy Rights Act (APRA) and President Biden’s October 30 executive order on AI. The White House OST has issued other directives too, including the March 2023 report on the importance of privacy-preserving tech.
Regulators in Europe and Asia are also starting to use the same language. For instance, the U.K.’s Information Commissioner’s Office recently stated that organizations should be using privacy-enhancing technologies so they can leverage data in a GDPR-compliant way, Hughes said. Regulators in Singapore and Canada have issued similar guidance on the technology.
“It continues to give us traction, because oftentimes people ask the question ‘What do regulators say?’ And now we have an answer,” Hughes said. “So I feel that the market is moving quickly and 2024 is going to be a great year. We’re going to see a lot more adoption.”
Obfuscating the Real World…
Duality Technologies offers a range of privacy-preserving services that allow customers to get value out of sensitive data. The company owns dozens of patents on homomorphic encryption, which allows users to do something that seems impossible: manipulate, analyze, and even train machine learning models using encrypted data.
But Duality’s offerings go beyond homomorphic encryption, which isn’t a good fit for all privacy-encryption use cases. For instance, since homomorphic encryption only supports a handful of classic machine learning algorithms, such as logistic regression and classifiers, so it can’t be used to train a large language model (LLM).
The company also leverages federated learning techniques for training AI models and running inference using sensitive data that cannot be moved. With this approach–which is useful for bringing two data collaborators together–the training or inference is performed at one endpoint, the intermediate results are encrypted, brought to a common location, where it’s joined with the other parties’ encrypted data and the compute is run on the encrypted joined data.
Finally, it supports Trusted Execution Environment (TEE). With a TEE, encrypted data is moved into a secure computing environment and decrypted into clear text for the computation to be done. Once the computation is done, the answer is encrypted before it’s sent to the final destination. TEEs are offered on all of the major clouds. AWS Nitro Enclaves, Google Cloud’s Confidential Space, and Microsoft Azure Confidential Computing. Users can also set up their own TEEs using special chips from Intel and AMD.
“Our secret sauce on top of that is to make all of these technologies completely obfuscated from the end user,” Hughes said. “They don’t care. They just want the output. Data scientists want to be able to use the tools that they already have in house, and we facilitate that.”
…Brings Real-World Benefits
The benefits of privacy-preserving technologies are potentially enormous, particularly as generative AI begins to take off. That’s because concerns over data privacy and security are slowing the roll-out of AI, which is expected to bring trillions of dollars in new business and cost savings in the years to come.
According to Cisco’s 2024 Data Privacy Benchmark Study, for which it surveyed 2,600 security and privacy professionals across the world, 91% of organizations say they need to do more to reassure customers about their data use with AI.
“The risks of AI are real, but they are manageable when thoughtful governance practices are in place as enablers, not obstacles, to responsible innovation,” Dev Stahlkopf, Cisco’s executive VP and chief legal officer, said in the report.
One of the big potential ways to benefit from privacy-preserving technology is enabling multiple parties to share their most valuable and sensitive data, but do so in a privacy-preserving manner.
“My data alone is good,” Hughes said. “My data plus your data is better, because you have indicators that I might not see, and vice versa. Now our models are smarter as a result.”
Carmakers could benefit by using privacy-preserving technology to combine sensor data collected from engines.
“I’m Mercedes. You’re Rolls-Royce. Wouldn’t it be great if we combined our engine data to be able to build a model on top of that could identify and predict maintenance schedules better and therefore recommend a better maintenance schedule?” Hughes said.
Privacy-preserving tech could also improve public health through the creation of precision medicine techniques or new medications. Duality recently did some work with the Dana-Farber Cancer Institute and Harvard School of Medicine that involved combining people’s genomic data with their clinical data, with the goal of identifying potential health problems that could arise due to how an individual’s genetic disposition interacts with the real world.
One can now use computer vision algorithms with homomorphic encryption to analyze images without compromising the privacy of the user.
“Say I want to query a publicly available data source of satellite imagery–I’m just using this as an example–to identify where is it in the world that this type of plane has been found in a satellite image recently,” Hughes said. “So if I can encrypt a MiG fighter and I’m working in a particularly region in Kazakhstan, I want to be able to do that in a way that protects the law enforcement organizations from disclosing who it is they’re asking questions about.”
The examples go on and on. There are piles upon piles of data that companies can’t leverage for fear of violating someone’s privacy. Thanks to privacy-preserving technologies, companies can begin to use this data for advanced analytics and AI use cases without violating individuals’ right to privacy.
That doesn’t mean that companies can revert to a Wild-West, anything-goes mentality, as was typical in the early days of the big data revolution. Companies still must do the hard work to ensure that they’re not violating tenets of good data science. There are some data sets that should not be combined. Data bias, model bias, and model drift are still issues that demand the attention of competent and good-intentioned data science professionals.
“There’s no getting around the fact that you need to be able to account for that,” Hughes said. “Non of those things go away. It’s just at the point of computation when either training is occurring or inference is being derived, you need to know that what needs to remain sensitive is protected.”
Related Items:
In Homomorphic Encryption We (Must) Trust
Three Privacy Enhancing Techniques That Can Bolster the COVID-19 Response
Yes, You Can Do AI Without Sacrificing Privacy