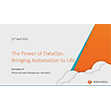
Unlocking the Full Potential of Generative AI for Enterprise Analytics
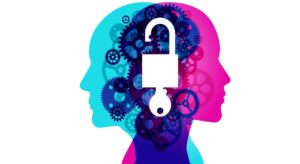
(Mr. Squid/Shutterstock)
Steve Jobs once compared the electric motor to the democratization of new technologies. When the electric motor was first invented, it could only be manufactured on a large-scale in a factory. They had great applications, but required significant resources to maintain, from elaborate belts and pulleys to a team of skilled mechanics. It was elusive to create a true lasting impact. Somewhere along the way, these large electric motors were downsized to fractional horsepower electric motors and proliferated to the point where we now easily have over 50 of them in the average household.
We’re at a similar crossroads with generative AI, where we’ll see this emerging technology proliferate to a point where it will be applied to nearly every possible use. An Alteryx survey found that nearly 80% of respondents are already seeing the benefits of generative AI in reaching their organizational goals, while predicting that their organization’s use of generative AI would increase from 32% to 53% over the next 3 years.
Generative AI has been pinned as the solution to many enterprise challenges. Enterprises looking to democratize data and analytics at scale are finding that generative AI proves to be extraordinarily fruitful. The reason is AI enhances enterprise analytics tools by improving their usability then synthesizing information in a consumable way.
Here we will explore how generative AI helps unlock the full potential of enterprise analytics. To do so, it’s important to understand the barriers that many organizations face when it comes to the democratization of analytics.
Pinpointing the Barriers
Achieving enterprise-wide analytics adoption is critical to empowering workforces – especially those in the line of business who typically do not have deep data skills. Every line of business leader needs to make highly informed decisions to win in an increasingly competitive market. This is easier said than done. For most, these can be summed up into three key challenges:
- People: Enterprises typically don’t have enough data scientists, AI or analytics experts to meet the demand for insights needed at a business-wide level.
- Systems: More often than not, enterprises are limited by having siloed and legacy systems that struggle to keep up with modern business questions that don’t fit neatly into any single enterprise system.
- Data: The increasing complexity and volume of data makes it much more challenging for enterprises to maximize its value. Using data meaningfully often becomes a slow, painful and ineffective exercise, leaving an untapped goldmine of usable data untouched.
Leveraging Generative AI for Added Efficacy
The good news is that generative AI can play a transformative role in overcoming these challenges. It can be harnessed to enable more people across all business areas to use analytics in their daily decision-making.
Generative AI makes analytics tools easier to use thanks to its ability to incorporate natural language interfaces, essentially allowing users to execute complicated tasks using basic English as a
“coding language.” Years ago, analytics tasks could only be executed with code – a skill that requires specific technical expertise that can take years to truly master. Then, visual tools made analytics more accessible. Now, generative AI shifts this paradigm further by enabling users to easily ask natural language questions to perform analytics tasks.
We also see tremendous gains in improving automation quality across the entire data analytics lifecycle. AI tools can translate more than just natural language. By being conversant across a wide array of coding languages and data formats, AI can be a powerful tool for automation by translating the enterprises stated expectations quickly into the systems of execution, without the burden of navigating the intricacies of instrumenting them all by hand.
Balancing Generative AI with a Unified Analytics Approach
As with every emerging technology, generative AI also presents several challenges, risks, and limitations to its wide adoption. This includes concerns around data privacy, exorbitant costs, and hallucinations or the generation of false information.
The key to balancing generative AI’s benefits and potential challenges is finding a solution or platform that bakes in different mechanisms able to control these challenges and follows responsible AI principles. Examples include prompt engineering techniques that make AI outputs trustworthy and reliable and data and metadata management capabilities that ensure data governance. Moreover, analytics is the perfect domain to practice responsible AI, as the presence of a domain expert analyst who understands the shape of the organizations data is always present to interrogate the result. With enterprise-grade guardrails such as these in place, companies can truly harness the potential of generative AI to create new heights in value from data.
The impact of generative AI in scaling and optimizing enterprise analytics is clear. When coupled with a governed enterprise-grade solution and a holistic approach that prioritizes democratizing access to data and analytics, companies can better leverage generative AI and unlock its full potential to drive better, more informed business outcomes.
About the author: Asa Whillock is Vice President and General Manager of Machine Learning and Artificial Intelligence at Alteryx. Asa’s 30 years of experience spans market-leading companies such as Intel, Macromedia, Adobe, and now Alteryx. His passion is incubating new businesses, having founded 13 across the domains of machine learning, analytics, platforms, streaming video, communications, privacy, security, and customer experience. Asa has also been granted 37 patents and received the 2016 Technology & Engineering Emmy and the 2017 Adobe Founders Award.
Related Items:
Linux Foundation Promotes Open Source RAG with OPEA Launch
What’s Holding Up the ROI for GenAI?
Why A Bad LLM Is Worse Than No LLM At All