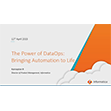
Data Quality Getting Worse, Report Says
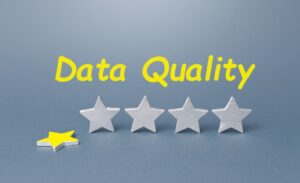
(Andrii-Yalanskyi/Shutterstock)
For as long as “big data” has been a thing, data quality has been a big question mark. Working with data to make it suitable for analysis was the task that data professionals spent the bulk of their time doing 15 years ago, and latest the data suggests that it’s an even greater concern now as we enter the era of AI.
One of the latest pieces of evidence pointing to data quality being a perpetual struggle comes to us from dbt Labs, the company behind the open source dbt tool that’s used widely among data engineering teams.
According to the company’s State of Analytics Engineering 2024 report released yesterday, poor data quality was the number one concern of the 456 analytics engineers, data engineers, data analysts, and other data professionals who took the survey.
The report shows that 57% of survey respondents rated data quality as one of the three most challenging aspects of the data preparation process. That’s a significant increase from the 2022 State of Analytics Engineering report, when 41% indicated poor data quality was one of the top three challenges.
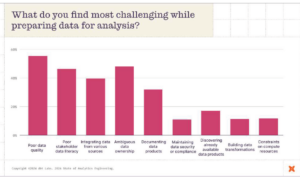
Data quality was cited as the number one concern during data prep, per dbt Labs State of Analytics Engineering 2024 report
Data quality isn’t the only concern. Other things that worry data professionals include ambiguous data ownership, poor data literacy, integrating multiple data sources, and documenting data products, all of which were listed by 30% of the engineers, analysts, scientists, and managers who took the survey last month. Lesser concerns include security and compliance, discovering data products, building data transformations, and constraints on compute resources.
When asked whether their organizations would be increasing or decreasing investments in data quality and observability, about 60% of the dbt survey respondents said they would keep the same investment, while about 25% said they would increase it. Only about 5% said they would decrease investment in data quality and observability in the coming year.
Dbt isn’t the only vendor to find that data quality is getting worse. Data observability vendor Monte Carlo published a report a year ago that came to a similar conclusion. The vendor’s State of Data Quality report found that the number of data quality incidents was on the rise, with the average number of incidents increasing from 59 per organization to 67 in 2023.
Another data observability vendor, Bigeye, also found that data quality was a top concern among its users. It found that one-fifth of companies had experienced two or more severe data incidents that directly impacted the business’s bottom line in the previous six months. The average company was experiencing five to 10 data quality incidents per quarter, it said.
The downward trend is data quality is not a confidence builder, particularly as data becomes more critical for decision-making. As companies begin to lean on predictive analytics and AI, the potential impact of bad data grows even more.
In 2021, Gartner study estimated that poor data quality costs organizations an average of $12.9 million per year, which is a staggering sum. However, the smart folks from Stamford, Connecticut expected data quality to be increasing in the years to come, not going down.
Bad data is particularly harmful for generative AI. In February, an Informatica survey that looked into the top challenges to implementing GenAI found that–you guessed it–data quality was at the top of the list. The survey found that 42% of data leaders who are currently deploying GenAI or planning to cited data quality as the number one barrier to GenAI success.
Will we ever solve the data quality issue once and for all? Not likely, according to Jignesh Patel, computer science professor at Carnegie Mellon University and co-founder of DataChat.
“Data will never be fully clean,” he said. “You’re always going to need some ETL portion.”
The reason that data quality will never be a “solved problem,” Patel said, is partly because data will always be collected from various sources in various ways, and partly because or data quality lies in the eye of the beholder.
“You’re always collecting more and more data,” Patel told Datanami recently. “If you can find a way to get more data, and no one says no to it, it’s always going to be messy. It’s always going to be dirty.”
If a user managed to get a “perfect” data set for one particular data analysis project, there’s no guarantee that it will be “perfect” for the next project. “Depending upon the type of analysis that I’m doing, it may be completely fine and clean, or it could be completely messy and mucky,” he said.
Related Items:
Data Quality Top Obstacle to GenAI, Informatica Survey Says
Data Quality Is Getting Worse, Monte Carlo Says