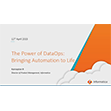
Why Digital Transformations Failed and AI Implementations Are Likely To
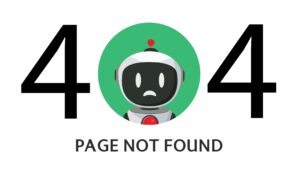
(ZeinousGDS/Shutterstock)
In January, IBM presented a detailed study on why digital transformations have only provided between a -5% and 10% ROI instead of the projected 150% ROI. This is a huge delta that’s in line with our experiences with initial client/server implementations in the 1980s, operating system migrations in the 1990s, big data implementations in the early 2000s, and analytics implementations over the last decade.
This isn’t to say all implementations failed to the degree mentioned above, only that the vast majority did. The recurring problem is a lack of maturity in the technology, the companies that are providing that technology, and the companies that deploy it wrapped with impressive sales efforts that convince the buyer of the benefits coupled with a lack of follow up to assure the promised benefits have been achieved.
The same thing is happening right now with AI. At the front-end, you don’t need sales promises. You need vendors that understand the technology and that will or have spent the time understanding your unique needs and can help you not only create the ideal solution but have the rigor in their process and the experience to assure the outcome.
Let’s talk about how to avoid this recurring problem.
The Cause
I’ve been through each of those earlier painful trends. I was working at IBM when the client/server trend hit, and even though the technology wasn’t ready at the time, it nearly put the company under as the market attempted to switch to something that wasn’t ready.
The cause is that sales can often get ahead of where the products are and well ahead of the services that must surround them. When a new trend like AI hits, everyone and their brother wants on the gravy train. But consider that last year, other than companies like IBM and NVIDIA that have been working on AI for decades, no one else (including Google which had collapsed its effort) was at all ready for Microsoft’s announcement that it was taking AI vertical.
The reason IBM is so excited this time around is that it has watsonx which is the most mature AI solution currently in market. Also this time, IBM is the most mature in terms of enterprise-class generative AI capabilities, while others are living off sales and marketing promises with little or no underlying foundation.
When sales gets ahead of the technology, the buyer gets screwed and, according to the IBM numbers on digital transformation, a lot of people were screwed because they didn’t do their due diligence.
The Fix: Do Your Homework and Follow a Process
One of the most successful processes in a situation like this is “pilot to production.” Don’t move to deployment in one painful step. Once you are convinced a vendor has a solution mature and complete enough for you, create one or more pilot projects to validate your belief. Even mature products don’t work in every situation. You don’t want to fail at scale, but failing in a pilot is recoverable.
But even before the pilot, you need assurance that the vendor’s claims on benefits and ROI are achievable. Ask for references from others who have deployed and achieved the promised benefits, query the vendor to see if they have deployed this technology internally, and ask to speak to the IT folks in the company that did it (they will often be surprisingly honest with you).
Do your research with others who are attempting this same task and capture best practices, realizing that not every solution will work with every company or even every division.
Hybrid multi-cloud is the practice that provides the greatest balance between uptime, cost, availability, and reliability. You need a vendor that understands this concept, has deep relationships with the cloud vendors you trust, and that has also gained enough experience so it isn’t learning on your nickel.
Particularly with AI data, quality is critical, and you’ll need a lot of help to assure it. You don’t want a biased or hallucinating AI any more than you wanted analytics that consistently provide inaccurate answers.
These new AI capabilities are expected to be multimodal and encompass natural language, images, audio, video, and even critical time elements. And the AI’s uses tend to optimize against one of these data types and perform poorly on others, so you need to understand the difference and have a vendor that is willing to tell you another vendor might be a better choice in areas where it doesn’t have competence.
Finally, you need help with metrics and milestones so that if a vendor isn’t performant, you can determine the problem early and either change vendors or change teams. If the vendor you are working with can’t help you set up metrics and objective goals for the project, you have the wrong vendor.
Wrapping Up:
The recurring problem we have with big recent technologies ranging from client/server in the 1980s to AI today, is that sales gets way ahead of where the product and support structure is. The result is that deployments fail to meet goals and expectations. If you don’t have a way to bring the pitch back to reality or to understand that the vendor is too far over their skis and likely to fall on your dime, you’ll be screwed.
Were I still in IT, I’d develop a relationship with NVIDIA, which is providing much of the core technology and use it as a sounding board to help select a short list of vendors and service providers who are up to speed on AI even though it will likely be another vendor you’ll buy from who uses their technology. I’d back this up by talking to my peers at industry events who are or have deployed similar solutions and get a feel for which vendors are executing well and use that information to qualify the vendors I’d want to research.
Toward the top of my list would be vendors who had deployed similar solutions to what I’m anticipating internally and externally, but not take any of this at face value. And I would interview those with knowledge of these deployments to validate results and the skill set of the company.
In many cases, it may be wiser to wait until the right partner, the right team, and the right solution is available before you pull the trigger and find out real-time how badly one of these deployments can go.
About the author: As President and Principal Analyst of the Enderle Group, Rob Enderle provides regional and global companies with guidance in how to create credible dialogue with the market, target customer needs, create new business opportunities, anticipate technology changes, select vendors and products, and practice zero dollar marketing. For over 20 years Rob has worked for and with companies like Microsoft, HP, IBM, Dell, Toshiba, Gateway, Sony, USAA, Texas Instruments, AMD, Intel, Credit Suisse First Boston, ROLM, and Siemens.
Related Items:
2024 and the Danger of the Logarithmic AI Wave
How Hybrid AI Will Enable Far Greater AI Use
The Three Approaches to AI Implementation