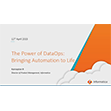
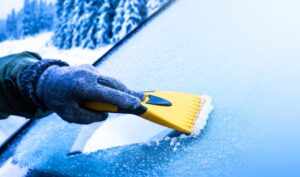
(Kim Kuperkova/Shutterstock)
Snowflake made a slew of announcements today during its “Snowday 2023” launch event, including a new generative AI offering dubbed Cortex, updates to its Snowpark environment for traditional machine learning, support for Iceberg Tables, updates to its Horizon data governance tool, and a new Snowflake Notebook.
The company describes Snowflake Cortex as a fully managed service for building and running all sorts of AI applications in its cloud, including generative AI applications. Currently in private preview, Cortex spans a set of serverless “specialized functions” and “general-purpose functions” that customers can call with a few lines of SQL or Python.
Specialized functions in Cortex include language models that can detect sentiment in text, summarize text, extract answers from text, and translate text into other languages. There are also specialized functions for tapping into traditional machine learning models, such as for forecasting, anomaly detection, and classification.
In the “general-purpose function” bucket, we find large language models such as Meta’s Llama 2 and several “high-performance Snowflake LLMs” that will enable customers to “chat” with their data, the company says. We also find things like vector embeddings and vector search capabilities in the general-purpose function bucket of Cortex. Snowflake is also adding vector as a native data type within its data cloud.
As a serverless offering on the Snowflake Data Cloud, Cortex is simple to use doesn’t require any AI expertise, doesn’t require the need to set up GPUs, and borrows from Snowflake’s inherent security, says Sridhar Ramaswamy, Snowflake’s senior vice president of AI.
“This is great for our users because they don’t have to do any provisioning,” Ramaswamy said at a press conference last week. “We do the provisioning. We do the deployment. It looks just like an API, similar to, say, what OpenAI offers, but it’s done right within Snowflake. Data does not leave anywhere. And it comes with the kind of guarantees that our customers want and demand, which is that the data is obviously isolated. It’s never intermingled in any kind of cross-customer training.”
As part of its Cortex launch, Snowflake is also unveiling private previews of a few “native LLM experiences” that will provide GenAI capabilities that leverage Cortex functions. This includes Document AI, Snowflake Copilot, and Universal Search.
These building blocks, combined with the public preview of support for the Streamlit development environment in Snowflake, should help to turbo-charge LLM and GenAI application development, such as for chatbots, Ramaswamy said.
“A chatbot is nothing but a combination of vector indexes and a language model that uses retrieval done on the index to do the prompting,” he said. “And this is when we give the power into the hands of our users so that the more adventurous among them can build meaningful applications very, very quickly.”
Snowpark Updates and a Notebooks Too
The company also today launched a private preview of Snowflake Notebooks, which enable users to explore data and develop machine learning applications in a familiar Juptyer-like environment running on their laptop.
“This brings you a cell-based development experience where you can build on a cell, execute, and iteratively grow–mix and match across SQL, Python, and markdown,” said Jeff Hollan director of product for Snowflake Developer Platform and Snowpark.
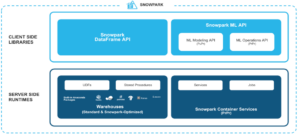
Snowpark provides libraries and runtimes for manipulating non-SQL data in Snowflake (Image source: Snowflake)
“A big part of analyzing and understanding your data is visualization, and this notebook integrated directly with Streamlit visualizations,” he continued. “I often like to say one my favorite things about Steramlit is there is no such thing as an ugly Streamlit app. Streamlit just has beautiful visualization out of the box, and you can use those exact visualizations inside of your notebook to get insight into your data and what it’s doing.”
Snowflake Notebooks is a component of Snowpark, the company’s collection of Python, Java, and Scala runtimes and libraries for working with non-SQL data housed in Snowflake. The company made several other announcements regarding Snowpark aimed at customers who are trying to develop traditional machine learning models on data housed in Snowflake.
For starters, it announced that the Snowpark ML Modeling API will soon be generally available. This API empowers developers and data scientists to scale out feature engineering and simplify model training for faster and more intuitive model development in Snowflake, according to the company.
It also announced Snowpark Model Registry, which will provide a one-stop-shop for cataloging and accessing all of the models used across the Snowpark environment, including traditional ML as well as LLMs for GenAI. The Snowpark Model Registry will be in public preview soon.
Lastly, it announced the start of a private preview for the Snowflake Feature Store, which will provide a repository for creating, storing, managing, and serving the ML features that data scientists and machine learning engineers want to use to train a model, as well as for running inference.
“These are three really exciting building blocks,” Hollan said. “The theme of all of these is allowing you to take those best practices that exist in the machine learning ecosystem but bringing in the simplicity, and the scale, and performance that SF can uniquely provide.”
New Horizon for Governance
Data governance has always been a core building block for developing and sustaining AI development. But now that the GenAI explosion is turbocharging interest in AI, data governance has emerged as a real stumbling block that could prevent all forms of AI success.
To that end, Snowflake today made updates to Horizon, its pre-existing offering for automating data governance tasks such as compliance, security, privacy, interoperability, and access capabilities in Snowflake’s cloud.
Without good data, every AI project will fail. With that in mind, Snowflake is launching a Horizon capability called Data Quality Monitoring. Currently in private preview, Data Quality Monitoring is aimed at making it easier for customers to measure and record data quality metrics for reporting, alerting, and debugging, the company said. Another new Horizon capability in private preview is Data Lineage, which is designed to give customers “a bird’s eye visualization of the upstream and downstream lineage of objects,” the company says.
On the privacy and security front, customers soon will be able to utilize Differential Privacy Policies, a new Snowflake capability that will allow customers to protect sensitive data “by ensuring that the output of any one query does not contain information that can be used to draw conclusions about any individual record in the underlying data set,” the company says. It’s currently in development.
Snowflake is also shipping new data classifiers that will enhance customers capability to define what sensitive data means in their business. Finally a new Trust Center, which will soon be in private preview, aims to help customers streamline their cross-cloud security and compliance monitoring by putting it in one place.
Cost is a perpetual concern when you run in the cloud, and something that Snowflake has said that it’s sensitive to. To that end, it is adding a new Cost Management Interface to Horizon that will enable admins “to easily understand, control, and optimize their spend,” the company says.
Last but not least, the company announced a public preview for Iceberg Tables. The company has already made Iceberg its preferred open table format, but there were some differences in how Iceberg tables were supported. With this announcement, it’s moving to simplify and unify that support.
“Instead of two separate table types for Iceberg, we are combining Iceberg External Tables and Native Iceberg Tables into one table type with a similar user experience,” Snowflake engineers Ron Ortloff and Steve Herbert wrote in a blog earlier this year. “You can easily configure your Iceberg catalog to match the capabilities you need.”
Related Items:
Databricks Versus Snowflake: Comparing Data Giants
Snowflake Gives Everybody a Little Something at Summit
Open Table Formats Square Off in Lakehouse Data Smackdown
July 3, 2025
- FutureHouse Launches AI Platform to Accelerate Scientific Discovery
- KIOXIA AiSAQ Software Advances AI RAG with New Version of Vector Search Library
- NIH Highlights AI and Advanced Computing in New Data Science Strategic Plan
- UChicago Data Science Alum Transforms Baseball Passion into Career with Seattle Mariners
July 2, 2025
- Bright Data Launches AI Suite to Power Real-Time Web Access for Autonomous Agents
- Gartner Finds 45% of Organizations with High AI Maturity Sustain AI Projects for at Least 3 Years
- UF Highlights Role of Academic Data in Overcoming AI’s Looming Data Shortage
July 1, 2025
- Nexdata Presents Real-World Scalable AI Training Data Solutions at CVPR 2025
- IBM and DBmaestro Expand Partnership to Deliver Enterprise-Grade Database DevOps and Observability
- John Snow Labs Debuts Martlet.ai to Advance Compliance and Efficiency in HCC Coding
- HighByte Releases Industrial MCP Server for Agentic AI
- Qlik Releases Trust Score for AI in Qlik Talend Cloud
- Dresner Advisory Publishes 2025 Wisdom of Crowds Enterprise Performance Management Market Study
- Precisely Accelerates Location-Aware AI with Model Context Protocol
- MongoDB Announces Commitment to Achieve FedRAMP High and Impact Level 5 Authorizations
June 30, 2025
- Campfire Raises $35 Million Series A Led by Accel to Build the Next-Generation AI-Driven ERP
- Intel Xeon 6 Slashes Power Consumption for Nokia Core Network Customers
- Equal Opportunity Ventures Leads Investment in Manta AI to Redefine the Future of Data Science
- Tracer Protect for ChatGPT to Combat Rising Enterprise Brand Threats from AI Chatbots
June 27, 2025
- Inside the Chargeback System That Made Harvard’s Storage Sustainable
- What Are Reasoning Models and Why You Should Care
- Databricks Takes Top Spot in Gartner DSML Platform Report
- Why Snowflake Bought Crunchy Data
- LinkedIn Introduces Northguard, Its Replacement for Kafka
- Change to Apache Iceberg Could Streamline Queries, Open Data
- Snowflake Widens Analytics and AI Reach at Summit 25
- Fine-Tuning LLM Performance: How Knowledge Graphs Can Help Avoid Missteps
- Agentic AI Orchestration Layer Should be Independent, Dataiku CEO Says
- Top-Down or Bottom-Up Data Model Design: Which is Best?
- More Features…
- Mathematica Helps Crack Zodiac Killer’s Code
- ‘The Relational Model Always Wins,’ RelationalAI CEO Says
- Confluent Says ‘Au Revoir’ to Zookeeper with Launch of Confluent Platform 8.0
- Solidigm Celebrates World’s Largest SSD with ‘122 Day’
- AI Agents To Drive Scientific Discovery Within a Year, Altman Predicts
- DuckLake Makes a Splash in the Lakehouse Stack – But Can It Break Through?
- The Top Five Data Labeling Firms According to Everest Group
- Supabase’s $200M Raise Signals Big Ambitions
- Toloka Expands Data Labeling Service
- With $17M in Funding, DataBahn Pushes AI Agents to Reinvent the Enterprise Data Pipeline
- More News In Brief…
- Astronomer Unveils New Capabilities in Astro to Streamline Enterprise Data Orchestration
- Databricks Unveils Databricks One: A New Way to Bring AI to Every Corner of the Business
- BigID Reports Majority of Enterprises Lack AI Risk Visibility in 2025
- Seagate Unveils IronWolf Pro 24TB Hard Drive for SMBs and Enterprises
- Astronomer Introduces Astro Observe to Provide Unified Full-Stack Data Orchestration and Observability
- Snowflake Openflow Unlocks Full Data Interoperability, Accelerating Data Movement for AI Innovation
- BigBear.ai And Palantir Announce Strategic Partnership
- Gartner Predicts 40% of Generative AI Solutions Will Be Multimodal By 2027
- Databricks Donates Declarative Pipelines to Apache Spark Open Source Project
- Code.org, in Partnership with Amazon, Launches New AI Curriculum for Grades 8-12
- More This Just In…