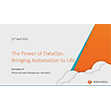
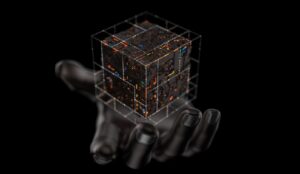
(Connect-world/Shutterstock)
What does “open” mean in the context of AI? Must we accept hidden layers? Do copyrights and patents still hold sway? And do consumers have the right to opt out of data collection? These are the types of questions that the folks at the Open Source Initiative are trying to get to the bottom of, as part of a deep dive to define “open source AI.”
The rules around what could be considered open source in tech used to be fairly well-defined, according to Stefano Maffulli, the executive director of the Open Source Initiative. Back in the 1970s, it was generally accepted that only things generated by a human could be legally protected with a copyright or a patent. Stuff generated by a machine, such as binary code, generally could not be protected.
That began to change with the PC revolution in the 1980s and Microsoft’s massive success selling software. Following several policy changes and landmark lawsuits, people began seeking and gaining protection for things such as source code and machine-generated binary code, Maffulli says.
With the advent of massive generative AI models that are trained on public data scraped from the Internet, we find ourselves at the edge of what current copyright law can cover. In fact, according to Maffulli, we’ve likely already passed that point, and now find ourselves in dire need of new ideas and new frameworks to define what can and should be protected, and what can and should be open and accessible to all.
“When [GitHub] CoPilot was announced [in October 2021], it suddenly dawned that there were new copyright issues appearing on the horizon,” Maffulli tells Datanami in a recent interview. “Then I started diving a little bit deeper into how AI [works], how machine learning, deep learning, neural networks work, and it dawned on me again that there were new artifacts, new things. And we were really at the dawn of a new era where we need new laws, we need new frameworks to understand what’s happening. And we need to do that very quickly.”
OSI ‘Deep Dive’
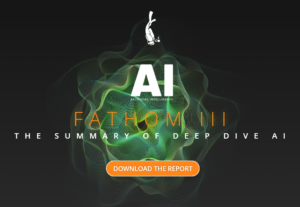
You can access the OSI deep dive report on open AI here
With its “Defining Open Source Deep Dive” program, the OSI organization is taking a disciplined and multi-pronged approach to understanding all aspects of the openness in AI question.
It set the process in motion earlier this year with a 20-page report on AI openness in February. In early June, it posted a public call for papers and research on the topic, followed by a set of kickoff meetings in San Francisco later that month. There were two community review workshops in July, in Oregon and Switzerland, followed by a third workshop last week in Spain.
If all goes according to schedule, OSI hopes to submit the first release candidate of a new definition of open source for AI paper next month. The process will continue into 2024, according to the group’s website.
The group is trying to remain open to all perspectives in coming up with its definition and policy recommendations. “It largely depends on what people want to do,” Maffulli says. “At the Open Source Initiative, we’re just driving this conversation. We’re not really forcing our opinions on anyone.”
A New Age of Data
The radical openness that defined the first 40 years of the Internet served the community well and sowed the seeds of technological progress to come. The egalitarianism of the Internet’s first phase of development fostered a community that thrived with openness and a ethos of sharing.
That started to change with the dawn of the big data age and the advent of social media and smart phones. Tech firms realized they could scrape the Internet for data freely shared by users, as well as some data not freely shared but still available (such as books), to amass huge data sets. Those data sets are now being used to train massive generative AI models that have the potential to not only reshape consumers’ relationship with technology for years to come, but also separate winners from losers on the corporate and creative battlefields.
One of the big questions that OSI is struggling with is: Does current copyright law still work in the age of AI? The answer hasn’t been determined yet, but it doesn’t look like it will.
“I think we’re at the point where we should make a decision whether we want those to be covered by copyright or whether we need to create new rights and new obligations for society,” Maffulli says. “What’s the best approach?”
There are different perspectives to these questions, and each deserves to be considered. The debate touches on several aspects of intellectual property rights, including copyrights, patents, trademarks, and trade secrets. But it’s also tied up into privacy rights, security obligations, and labor law, which adds to the complexity.
Maffulli says he understand the plight of creative workers whose past work can be harnessed to train a GenAI model that can re-create that workers’ output, potentially putting him out of work. Is there any legal recourse for him? Should he be granted legal protections? It’s tempting, he says.
“The reaction to that is to say, wait a second, you have been feeding my images, my text, into this machine and now this machine is capable of replacing me? No!” he says. “I have copyright rights on the work that I have produced. I never authorized anyone to use the archive of my work as a data mining source. Therefore, I want you to ask me for permission. I think that that’s a very fair approach a very fair reaction.”
However, if communities and government opt to stiffen data protections, it will naturally make it harder to obtain data to train AI models. That will not only slow down the overall rate of AI innovation, but it will likely also have the side effect of entrenching the already dominant positions that OpenAI, Google, and Meta enjoy in the space, he says.
“I think the biggest threat is there will not be the possibility to have a diverse amount of players in the field,” he says. “This is a field that naturally, at every step, favors the ones with the big resources, large amounts of resources. Because the main three components are data, knowledge, and hardware.”
The tech giants already have the data, which they have been systematically scraping from the Internet for years. They have the financial resources to afford the giant GPU clusters needed to train AI models. And they naturally attract the top minds in the field as a byproduct of having massive GPU clusters and lots of data to play with.
Maffulli sounds pragmatic about the potential to enact meaningful change by strengthening copyright protections. The tech giants already have the means to bury lawsuits brought by individuals, he says. And besides, they already have all the data. In many cases, they acquired it fair and square, thanks to consumers’ tendency to click “yes” on every privacy policy dialog box they’re presented.
‘Cat’s Out of the Bag’
For years Maffulli shared his image and title liberally across the Web. Then at one point, he tried to rein in back in by deleting his image on every major site. It’s his likeness and his right, he figured. He would force the tech giants to forget they ever saw him, he thought. At some point, he realized it was likely impossible.
That experience has informed his view on what is possible to be done with data and the open future of AI. “I think it’s better off if we just let it go,” Maffulli says. “The cat is out of the bag.”
In other words, instead of trying to put the cats back in the bag, we are better off just managing the loose cats as best we can. That means stronger operational controls on data that’s already out in the open, and better guardrails to guide those cats to happy homes.
“I do think that it cannot be solved by copyright law,” Maffulli says. “It needs to be solved by having strong policy, privacy protection laws, strong control from the individual to say ‘I don’t want to be recognized. Therefore, even if you have my face in the database, it gets deactivated. You cannot use it.’”
There are plusses and minuses to open source and to copyright protections, and they must be weighed carefully. OSI’s policy is not to judge how practitioners use open source software, noting that it’s impossible to draw a line between moral and immoral uses. As the debate plays out over what open means in AI, that line is murkier than ever.
Related Items:
Why Truly Open Communities are Vital to Open Source Technology
Do Customers Want Open Data Platforms?
Open Data Hub: A Meta Project for AI/ML Work
April 26, 2024
- Google Announces $75M AI Opportunity Fund and New Course to Skill One Million Americans
- Elastic Reports 8x Speed and 32x Efficiency Gains for Elasticsearch and Lucene Vector Database
- Gartner Identifies the Top Trends in Data and Analytics for 2024
- Satori and Collibra Accelerate AI Readiness Through Unified Data Management
- Argonne’s New AI Application Reduces Data Processing Time by 100x in X-ray Studies
April 25, 2024
- Salesforce Unveils Zero Copy Partner Network, Offering New Open Data Lake Access via Apache Iceberg
- Dataiku Enables Generative AI-Powered Chat Across the Enterprise
- IBM Transforms the Storage Ownership Experience with IBM Storage Assurance
- Cleanlab Launches New Solution to Detect AI Hallucinations in Language Models
- University of Maryland’s Smith School Launches New Center for AI in Business
- SAS Advances Public Health Research with New Analytics Tools on NIH Researcher Workbench
- NVIDIA to Acquire GPU Orchestration Software Provider Run:ai
April 24, 2024
- AtScale Introduces Developer Community Edition for Semantic Modeling
- Domopalooza 2024 Sets a High Bar for AI in Business Intelligence and Analytics
- BigID Highlights Crucial Security Measures for Generative AI in Latest Industry Report
- Moveworks Showcases the Power of Its Next-Gen Copilot at Moveworks.global 2024
- AtScale Announces Next-Gen Product Innovations to Foster Data-Driven Industry-Wide Collaboration
- New Snorkel Flow Release Empowers Enterprises to Harness Their Data for Custom AI Solutions
- Snowflake Launches Arctic: The Most Open, Enterprise-Grade Large Language Model
- Lenovo Advances Hybrid AI Innovation to Meet the Demands of the Most Compute Intensive Workloads
Most Read Features
Sorry. No data so far.
Most Read News In Brief
Sorry. No data so far.
Most Read This Just In
Sorry. No data so far.
Sponsored Partner Content
-
Get your Data AI Ready – Celebrate One Year of Deep Dish Data Virtual Series!
-
Supercharge Your Data Lake with Spark 3.3
-
Learn How to Build a Custom Chatbot Using a RAG Workflow in Minutes [Hands-on Demo]
-
Overcome ETL Bottlenecks with Metadata-driven Integration for the AI Era [Free Guide]
-
Gartner® Hype Cycle™ for Analytics and Business Intelligence 2023
-
The Art of Mastering Data Quality for AI and Analytics
Sponsored Whitepapers
Contributors
Featured Events
-
AI & Big Data Expo North America 2024
June 5 - June 6Santa Clara CA United States -
CDAO Canada Public Sector 2024
June 18 - June 19 -
AI Hardware & Edge AI Summit Europe
June 18 - June 19London United Kingdom -
AI Hardware & Edge AI Summit 2024
September 10 - September 12San Jose CA United States -
CDAO Government 2024
September 18 - September 19Washington DC United States