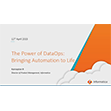
Four Dimensions of Analytics
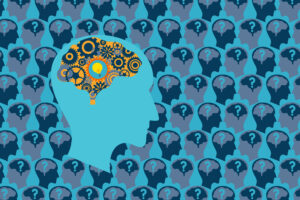
(apixelstudio/Shutterstock)
We’ve all heard the adage “data is the new oil” – a valuable, abundant resource that is only useful once refined. However, one of the most striking yet daunting comparisons is sending ships into the ocean for oil discovery; some will fail, and some will have a massive discovery.
Deep-sea exploration and drilling depend on precise mapping and a clear understanding of the ocean floor. The same is true in analytics; the most successful will have access to a trustworthy knowledge of enterprise data assets. That means having a robust data cataloging and governance process that provides context about assets, leverages the experience of others, builds trust around data, and helps understand the lineage of data as it flows through various systems. Business users want to understand and trace the metrics they see in BI reports to cloud data warehouses and, finally, to the source systems from which the data originally came. More importantly, it means having data-literate employees who can apply critical thinking to analytics.
Most companies have an overconfidence bias related to the analytic literacy of their employees, effectively nullifying any investment in programs and platforms meant to assist them.
Solving this begins by creating a strategic initiative to become a data-driven company and following through by creating a data literacy program.
Articulating the Problem
According to Jordan Morrow, author of the book “Be Data Literate,” there are four main stages to data analytics: descriptive, diagnostic, predictive, and prescriptive.
The descriptive stage is exactly how it sounds: companies describe situations with their data in a backward-looking manner. It tells us what happened and is what we see in 99% of the world’s dashboards, list reports, and business intelligence reports. They compare metrics over time for dimensions such as department, geography, store, product segment, and customer segment.
Descriptive analytics leads to diagnostics analytics, answering the ‘why?’ behind the descriptive-analytic results. While retrospective in nature, descriptive analytics provides a more detailed root-cause analysis that requires both subject matter and analytic domain expertise. Often this is the aim of programs and tools labeled as self-service.
Once you deeply understand the cause and effect of the ‘why,’ you can start to predict what will happen. Predictive analytics offers the first ability to look forward, so we can influence the future. In retail, Amazon suggests related products. In the business world, supply repurchase notifications based on depletion rate or the shift of parts to warehouses based on regional weather.
Finally, we can and should use all the analytical knowledge gathered to do more than simply ‘know the future.’ We should change the future by providing actions/activities. This is prescriptive analytics. If we know a high likelihood of something happening (predictive), we carry out measures to avoid, or ensure, it happens. For instance, if we predict the need for supply replenishment based on specific events and seasonal timing, we could prepurchase in the off-season and pre-stage to lower supply costs and smooth supply.
There is a three-fold problem related to data literacy:
- Access to trusted data. Most companies don’t provide transparent access to reliable data and continue to operate with minimal cross-functional sharing of assets. The results are a painstakingly slow and manual process of finding data fit for purpose, wasted time and money recreating the same analysis, and navigating inconsistencies in analytic results.
- Lack of data literacy training. There is a limited group of people data literate enough to move beyond the simple acceptance of descriptive analytics. Many require more training or need to be actively encouraged to apply critical thinking to descriptive results. To do so requires basic knowledge related to the use of scale, comparisons, basic calculations, units of measure, and time frames. Think of an organization that has repeatedly rolled out better self-service tools (mouse traps) without any corresponding data literacy training curriculum.
- Reliance on instinct versus proof. Leaders continue to rely on experience and ‘gut feel’ instead of deploying predictive and prescriptive analysis with empirical evidence that could help them continually optimize their business.
Setting Up for Success
A pre-step to descriptive analytics is creating a data asset knowledge base. We call it a catalog, also characterized as a data asset-centric knowledge base that supports technical and non-technical (business) personas.
The types of integrated assets in a catalog include term glossaries, metric glossaries, top users of crucial assets, discussion threads, voting, favorites, report and dashboard inventories, data classifiers and domains, related policies, quality indicators, popularity, published queries, and data products. All these assets are easily accessible using text-based search and natural language questions using a browser, spreadsheet, or messaging tool, such as Slack.
A best practice employed by leading companies is to create a data literacy curriculum in parallel with preparing the catalog, intertwining the two. Typically, the data literacy curriculum lives in a learning management system (LMS). Interlacing the catalog and LMS creates learning paths and links to data literacy courses from key asset pages in the catalog for specific personas. The goal is to supply learning at the point of data use and when someone needs it, as opposed to a separate (old, failed style) and mandatory data literacy training employees speed through to check the compliance box for the boss.
Moving Beyond Descriptive Analytics
Once set up, the catalog and data literacy curriculum can be systematically rolled out across business units. The typical roll-out includes onboarding courses that provide a baseline level of literacy for everyone, plus more specialized courses for understanding predictive and prescriptive analytics.
Data literacy is an ongoing program, not a one-and-done project. Appoint leaders who encourage engagement and reward employees for continuous participation.
What’s also vital is developing a culture of continually capturing and sharing what everyone learnings from analytical use. This creates a cycle of continuous improvement and a data micro-culture, reflecting the enterprise’s strategic goal.
Predictive and prescriptive analytics requires a much higher level of data literacy and specialized statistical/AI/ML skills to implement, which can be a significant hurdle. The goal should be to establish a pool of knowledgeable people to create functional requirements, supervise, validate, and sign off on the efficacy of analytic models built by a central team of experts or external consultants.
Regardless of who creates the more sophisticated models, avoid allowing them to be ‘black boxes’ without absolute transparency. All models and analytical approaches should be added to the catalog, fully described, and related to the data it consumes and produces. Now, you’re driving open participation and prioritizing shared learning and understanding.
Mastering the Art of Being ‘Data-Driven’
The good news is most companies realize they need a data culture to effectively harness their data. In fact, nearly 80% of businesses have a strategic initiative to become more data–driven. But knowing something and taking action to make progress is different. Data culture isn’t something you can buy; it’s something you build. A data-driven culture is something that starts at the very top.
If your company needs help with data management practices, now is the time to reevaluate current solutions and implement new strategies to get back on track. There are more tools than ever to help businesses make the most of their information and reach higher levels of analytics, from new software platforms and greater awareness of the importance of data literacy to technologies like AI that can help make collecting and organizing data more efficient. So, it is important to think big, but start small, and learn quickly.
About the author: Diby Malakar is the vice president of product management for Alation. Diby has more than 25 years of experience in the data management space and previously was head of product at Confluent and a vice president of product management at Oracle. Diby has also played numerous leadership roles in engineering and product management at companies like SnapLogic, Informatica, KPMG and TiVo. He has a Bachelors degree in Computer Science and an MBA from Santa Clara University.
Related Items:
The Critical Importance of Data Literacy
5 Steps to Data-Based Decisions
Achieving Data Literacy: Businesses Must First Learn New ABCs