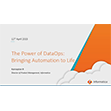
Enterprise AI: A Slow Progression Toward the Frontal Lobe, Not a Race to the Bottom of the Brain Stem
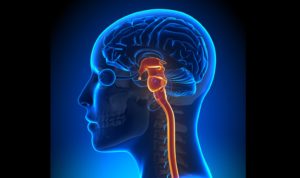
(decade3d-anatomy-online/Shutterstock)
What is your daily experience as a consumer with AI? Newsfeeds dominated by high school friends and anger-inducing political memes? Trying to get kids to put down addictive devices? Maybe even a sense of loss when you find there’s no middle ground in trying to talk with people who see the world differently?
For me–a professional working mom living in San Francisco, working with diverse, global colleagues, with personal ties to the South and to Idaho–these are the most real and tragic results from consumer AI. You may feel different results more acutely, but I hazard to guess that you, too, have personally experienced some of the negative effects from consumer AI. No one could try to claim consumer AI is all negative, of course. Optimized drive routing, personalized healthy reminders, and a wider range of music discovery are the features I appreciate the most.
Still, the dark side of consumer AI should not get washed over as a mere side effect of innovation. It’s a complicated problem worthy of scholars and congressional hearings, and not my focus here, except in so far as to draw a contrast. Tristan Harris, director of the Center for Humane Technology, a former Googler, and bearer of many other qualifications in AI, testified before a US Senate hearing committee: “Today’s tech platforms are caught in a race to the bottom of the brain stem to extract human attention.”
The visceral image of human decline as animalistic instincts take over our actions online is not simply an analogy. Mr. Harris is referring to the base instinct that these consumer AI platforms are designed to optimize, to continually increase as the algorithms improve: the dopamine rush that comes from likes and shares or from fear and outrage.
And that is the central question that differentiates consumer AI and enterprise AI: What metric is the AI designed to maximize? Or, where is the AI taking us?
For many consumer platforms, the metric being optimized is “engagement” – how long a person stays on a page watching videos, or how many shares, posts, comments, and emoji responses they make. When it comes to enterprise AI however, the metrics we optimize are fundamentally different. Some of those metrics include throughput, accuracy, and cost savings. Optimizing these enterprise metrics is a challenging job for data scientists because the influencing factors are complex, and results are less immediate to measure. Focusing AI algorithms on these enterprise metrics also has the important effect of directly benefitting organizations, while elevating, rather than debasing, human decision making.
Unlike consumer AI, which is in a race, (more on the destination later) enterprise AI is on a slow and steady progression, and there’s good reason for the difference. First, the metrics being optimized are more complicated. Enterprise applications are configured very differently for customers in different industries, with different management structures, different sales models, different financial reporting requirements, etc. That means the definition of something seemingly as straightforward as “throughput” for invoice processing, for example, may manifest differently for a public telecommunications company vs. a small private service company vs. a B2B industrial manufacturer vs. an acquisitive financial holding company – due to the semantic variation in their data. Enterprise AI data pipelines automate and accommodate these semantic variations, and this increases the overall complexity.
The core purpose of enterprise applications is to be the transactional system of record for an organization’s most important transactions. The power of enterprise AI is to turn these transactional systems into systems of intelligence, helping customers harness their data in more powerful ways. The process of harnessing data for new data science-driven opportunities inevitably uncovers gaps in data availability in those transactional systems of record. For example, harnessing the power of the sales transactional system to optimize the sales process is a perfect use of enterprise AI, but when the CRM is not used consistently or regularly by salespeople, data is too sparse and may lead to misleading results from algorithms.
In cases with sparsely populated data, the solution can be to prioritize enterprise AI use cases that lead to more fully populated data as a first step, then to make more powerful recommendations and AI-automated actions as data availability improves. As an example, an HR system may drive significant functionality based on the skills profile of an employee, but if, in practice, the skills profile of most employees remains empty, other higher order functionality must take a backseat to AI-driven (or perhaps even manual, human-driven) nudges that encourage employees to choose recommended skills for their profile. The need to increase the richness of data captured in customers’ enterprise applications is another reason behind the slow, steady progression of enterprise AI.
We all know the hero of the story is the tortoise not the hare, so the fact that the progression of enterprise AI is slow and steady shouldn’t be cause for concern. What’s more important is the difference in the destinations between enterprise AI and consumer AI. As Tristan Harris testified to Congress and as many others have written about, the focus on “engagement” as the metric for consumer AI to optimize has led these solutions to capitalize (and exacerbate) human weaknesses. As humans we all have instincts, bad habits, and insecurities, and the best way to get us to watch longer, share more, or check and refresh repeatedly is to trigger these tendencies most of us would call weaknesses – the human tendencies that originate “from the bottom of the brain stem” according to neuroscience.
In contrast, we know that the positive human qualities of reason, self-control, problem-solving, etc. that originate from the frontal lobe of the brain are the desirable human traits we strive to exercise. Fundamentally, the human decisions that we want AI to emulate and optimize in the context of enterprise technology, are those decisions that emanate from the “executive functions” of the frontal lobe, according to neuroscience.
Decisions about which prospects to call on, how to handle accounting for different expenses, how to respond quickly and accurately to a service request–these are the realm of enterprise AI, and they simply aren’t made more effective or efficient by exploiting human weaknesses. Skeptics will argue that it is still possible to distort business decisions with AI that preys on our base instincts. Unchecked enterprise AI that recommends candidates to hire could exploit human biases that lead to lack of diversity. Enterprise productivity tools could increase proliferation and usage with addictive content that doesn’t actually help employees get their jobs done.
However, the very nature of business productivity is a natural counterbalance to implementing AI code written in bad faith. The goals of enterprise AI customers are literally at odds with our exploitable bad habits. Enterprises wouldn’t benefit from their employees being distracted or from employees’ individual biases distorting company hiring decisions, but they can make extraordinary gains from software that can be built to emulate executive function. As such, the enterprise AI vendors that sell to these enterprises also have no incentive to resort to techniques that drift toward the bottom of the brain stem rather than continually aiming for the frontal lobe. To be blunt, there’s no business value in doing so.
That brings me to the personal side of this story. Being confronted with the dark side of consumer AI in daily life leads to a lot of soul-searching for every person of good faith who is working in this industry. Tristan Harris, as one example, decided that he couldn’t make the changes that he thought needed to happen from within Google, so he started making change from the outside. I’m quite certain that many other smart, well-intentioned people decide to work on ethical AI efforts within their consumer AI workplaces, and certainly others find their own personal ways to reconcile the cognitive dissonance that arises from being part of the wave of AI technology.
For me, the right balance is to focus on AI work in the enterprise, knowing full well that we are on a longer road than our consumer-focused colleagues. This longer road may lack the thrilling speed of consumer data that is measured in thousands of orders of magnitude greater than enterprise data, but it brings other technical delights related to complexity, and most importantly to me, genuinely solves problems for customers. Finally, I take heart in knowing that it is also the higher road. We continue to set our optimization functions toward the metrics that come from the “executive functions” of the frontal lobe. It’s gratifying to be the tortoise, especially when you’re going the right direction.
About the author: Miranda Nash serves as a Group Vice President of Product Development & Strategy at Oracle. She is responsible for the Oracle Adaptive Intelligent (AI Apps) products designed to bring machine learning, data-driven capabilities, and differentiating business value to Oracle’s SaaS portfolio. Miranda started her career at Oracle as an engineer in Server Technologies, and she has held various engineering and product leadership roles inside and outside the company spanning 25 years. Before her most recent return to Oracle, she was Co-Founder/CEO at Qeople, the talent marketplace for senior-level part-time professionals. Previously, she founded a boutique private equity firm and led Jobscience, a SaaS provider to the recruiting industry, as the company’s President. She holds an MBA from Stanford Graduate School of Business and a B.S. in Computer Science from Stanford.
Related Items:
Composite AI: What Is It, and Why You Need It
A ‘Glut’ of Innovation Spotted in Data Science and ML Platforms