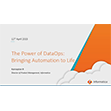
Why Integration and Governance Are Critical for Data Lake Success
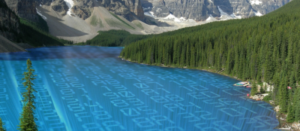
This is the final article in a three-part series exploring what it takes to build a data lake capable of meeting all the requirements of a truly enterprise-scale data management platform. While earlier installments focused on enterprise-scale data management in Hadoop, data onboarding into the data lake, and security, this article will focus on two things: Integrating the data lake within the broader enterprise IT landscape, and data governance.
As more lakes are deployed, we see patterns emerge for how data lakes are positioned relative to existing databases, data warehouses, analytic appliances, and enterprise applications in larger organizations.
Data Lakes: Here to Stay
Some data lakes are deployed from the outset as centralized system-of record data platforms, serving other systems in an enterprise scale, data-as-a-service model. As a centralized data lake builds momentum, collecting more data and attracting more use cases and users, its value grows as users collaborate on improving and reusing the data.
Other projects start at the edge of the organization to deliver data and meet the analytic needs of a specific business group. A localized data lake often expands to support multiple teams or spawn additional separate data lake instances to support other groups who want the same improved data access as the first group got.
Regardless of what pattern the data lake takes as it lands and expands in the organization, the data lake’s increasing role in the organization brings with it new requirements for enterprise readiness.
Integration Challenges
To be enterprise-ready, the data lake needs to support a set of capabilities that allow it to be integrated within the company’s overall data management strategy and IT applications and data flow landscape.
Here are some requirements to keep in mind:
- It must be possible to automate and embed the process of interacting with the data lake so that jobs to update the lake with new data or deliver data out of the lake can be automatically called and executed in a lights-out production mode. This means that the data lake needs to provide a RESTFul API that can be called by other scripts or schedules in the environment and which exposes all the functionality needed to interact with the data lake in a production environment.
- The data lake needs to be able to export data and associated metadata in multiple formats so that data from the lake can be easily integrated with other applications or downstream reporting/analytic systems.
- The data lake needs to support development, test, and production environments and allow for the easy promotion of data ingest, data preparation, and similar assets developed in the data lake environment from one environment to the next.
- The data lake needs to make it easy for parts of the lake to be shared across separate Hadoop clusters so that in a large organization with multiple data lakes, data, metadata and related assets can be easily and consistently shared.
- It must be possible for metadata collected and generated in the data lake to be exchanged with other enterprise standard metadata repositories.
Governing the Lake
In addition to streaming the integration of your data lake, you must prepare the lake to support a broad and expanding community of business users.
As more users begin working with the data lake directly or through downstream applications or reporting/analytic systems, the importance of having strong data governance grows. This topic — data governance — is the final dimension of enterprise readiness.
By bringing together typically hundreds of diverse data sets in a large repository and giving users unprecedented direct access to that data, data lakes create new governance challenges and opportunities.
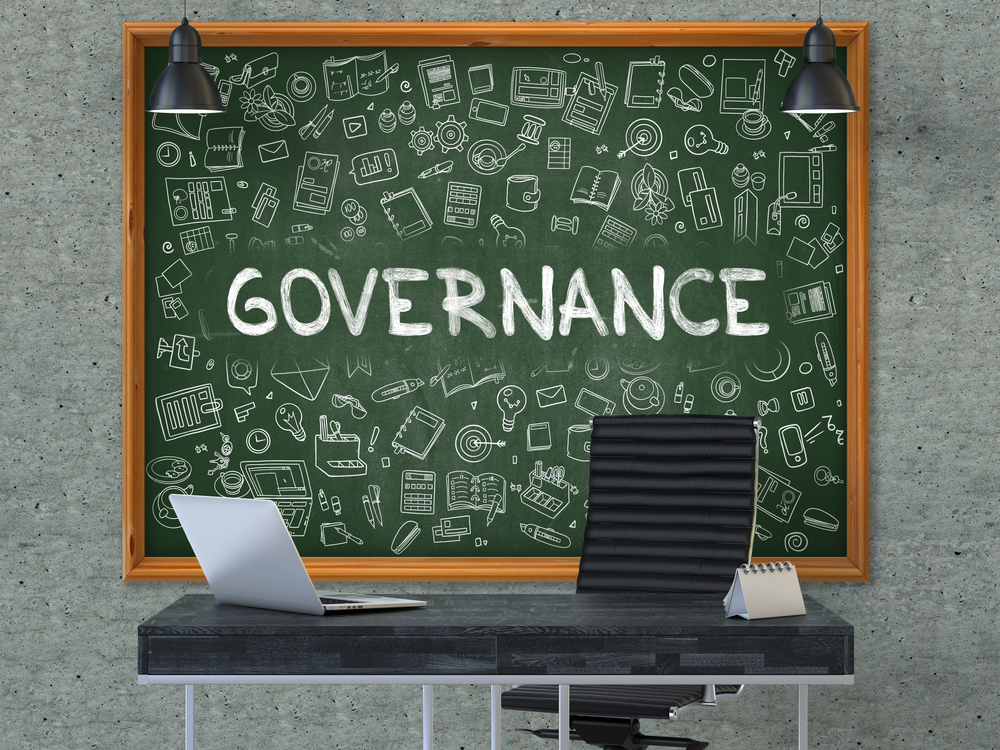
(Tashatuvango/Shutterstock)
The challenges have to do with ensuring that data governance policies and procedures exist and are enforced in the lake. Enterprise-ready data governance in the data lake starts with a clear definition of who owns or has custodial responsibility for each data asset as it enters the lake and as it is maintained and enhanced through the data lake process. In addition, the data lake needs to include well-documented policies regarding the required accuracy, accessibility, consistency, completeness, and updating of each data source.
To monitor and enforce application of these policies, the data lake environment must automatically profile each data source on ingest with respect to the data quality, character, and completeness. Additionally, the data lake should automatically track and record any manipulation of data assets (cleansing, transformation, preparation) to provide a clear audit trail of all users and activities occurring in the lake.
Finally, when it comes to enterprise-scale data governance in a data lake, it is essential that restrictions are in place to ensure that people only see the data they should be allowed to see. (See part two of this series for more on the importance of authentication, authorization and data access controls.)
Virtuous Cycles at Enterprise Scale
But data governance’s role in a truly enterprise-ready data lake isn’t only to reduce risk and enforce controls. It can also create added value and enable richer broader collaboration around data across users and groups.
If designed properly, data lakes are unique in their ability to allow large populations of non-technical business users to access, explore, and enhance data as they move it along the evolutionary path, from raw source system data to business user ready information.
Good data governance abets this process by helping business users enhance data with crowd-sourced business metadata and tagging that adds context, business definition, and meaning to the data. Combined with data governance policies that selectively cull and promote the best of this crowd-sourced insight to “gold standard” data in the organization, participation of a growing group of business users in the enterprise scale data lake can create a virtuous cycle in which user participation enhances data, bringing more users, more enhancement, and ultimately more value to the lake.
About the author: Dr. Paul Barth is founder and CEO of Podium Data, creator of the Podium big data management platform. Paul has spent decades developing advanced data and analytics solutions for Fortune 100 companies, and is a recognized thought-leader on business-driven data strategies and best practices. Prior to founding Podium Data, Paul co-founded NewVantage Partners, a boutique consultancy advising C-level executives at leading banking, investment, and insurance firms. In his roles at Schlumberger, Thinking Machines, Epsilon, Tessera, and iXL, Dr. Barth led the discovery and development of parallel processing and machine learning technologies to dramatically accelerate and simplify data management and analytics. Paul holds a PhD in computer science from the MIT, and an MS from Yale University.
Related Items:
Delivering on the Data Lake Promise
Building the Enterprise-Ready Data Lake: What It Takes To Do It Right
May 9, 2025
- BigID Introduces Executive Console to Streamline Privacy Reporting and Decision-Making
- Cerebras Partners with IBM to Accelerate Enterprise AI Adoption
- Franz Launches AllegroGraph 8.4 with Enhanced Natural Language Query for Agentic AI
- Peer Software Introduces New Features to PeerGFS for Multi-Protocol AI and Edge Workflows
May 8, 2025
- Amplitude Announces New Strategic Collaboration Agreement with AWS
- Domino Survey Shows Enterprises Prioritizing Governance Over GenAI Hype
- DataRobot Launches New Federal AI Application Suite to Unlock Efficiency and Impact
- Qlik Announces Close of Significant Investment Led by ADIA and Thoma Bravo
- OpenSearch 3.0 Enhances Vector Database Performance, Search Infrastructure and Scalability to Meet AI-driven Demand
May 7, 2025
- SAS Viya Expands AI Portfolio with Copilot, Intelligent Decisioning, and Synthetic Data Tools
- Grafana Labs Demonstrates Open Source Leadership at GrafanaCON 2025
- Neo4j Launches Industry’s 1st Graph Analytics Offering for Any Data Platform
- Cockroach Labs Brings Distributed SQL to IBM LinuxONE and Linux on IBM Z
- SAS Unveils AI Agents with Customizable Human-AI Interaction for Transparent Decisioning
- Coralogix Launches Continuous Profiling for Real-Time Application Visibility with Minimal Overhead
May 6, 2025
- Forrester Unveils Top 10 Emerging Technologies for 2025
- NetApp and Intel Partner to Redefine AI for Enterprises
- Algolia Introduces Smart Groups to Bring AI-Powered Curation and Automation to Content Teams and Merchandisers
- Traject Data Boosts Visibility into Google’s AI Overview with New Enhancements
- Qlik Accelerates Move to Cloud with New Analytics Migration Tool
- PayPal Feeds the DL Beast with Huge Vault of Fraud Data
- The Active Data Architecture Era Is Here, Dresner Says
- Slash Your Cloud Bill with Deloitte’s Three Stages of FinOps
- Thriving in the Second Wave of Big Data Modernization
- Monte Carlo Brings AI Agents Into the Data Observability Fold
- Inside the Chargeback System That Made Harvard’s Storage Sustainable
- AI Today and Tomorrow Series #4: Frontier Apps and Bizops
- Ambari Hadoop Cluster Manager is Back on the Elephant
- Databricks to Open Source Unity Catalog
- Three Ways AI Can Weaken Your Cybersecurity
- More Features…
- Google Cloud Cranks Up the Analytics at Next 2025
- GigaOM Report Highlights Top Performers in Unstructured Data Management for 2025
- SnapLogic Connects the Dots Between Agents, APIs, and Work AI
- Databricks and KPMG Invest in LlamaIndex to Unlock Scalable Enterprise AI
- AI One Emerges from Stealth to “End the Data Lake Era”
- Supabase’s $200M Raise Signals Big Ambitions
- Dataminr Bets Big on Agentic AI for the Future of Real-Time Data Intelligence
- Fivetran Aims to Close Data Movement Loop with Census Acquisition
- Do You Own Your Data? Third-Party Doctrine Says No
- Sigma Secures $200M Round to Advance Its BI and Analytics Solutions
- More News In Brief…
- Gartner Predicts 40% of Generative AI Solutions Will Be Multimodal By 2027
- GitLab Announces the General Availability of GitLab Duo with Amazon Q
- BigDATAwire Unveils 2025 People to Watch
- Dataminr Raises $100M to Accelerate Global Push for Real-Time AI Intelligence
- SAS Unveils AI Agents with Customizable Human-AI Interaction for Transparent Decisioning
- Databricks Announces Data Intelligence Platform for Communications
- Dremio Named Top Vendor in Dresner 2025 Active Data Architecture Report
- Kroger and NVIDIA to Reinvent the Shopping Experience Through AI-Enabled Applications and Services
- Dataminr Unveils Agentic AI Roadmap to Advance Real-Time Decision-Making
- LogicMonitor Expands AI Observability Platform with Agentic AIOps and New Partnerships
- More This Just In…
Sponsored Partner Content
-
Mainframe data: A powerful source for AI insights
-
CData recognized in the 2024 Gartner ® Magic Quadrant™ Report
-
Introducing AIStor, the most powerful version of MinIO to date
-
Designing a Copilot for Data Transformation
-
Get your Data AI Ready – Celebrate One Year of Deep Dish Data Virtual Series!
-
Supercharge Your Data Lake with Spark 3.3