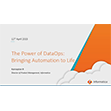
Speech-to-Text Puts New Twist on Sentiment Analysis
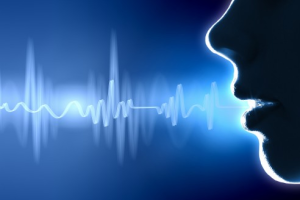
Mining Facebook and Twitter posts for clues about customer sentiment is one of the most common big data workloads today. Now, thanks to improvements in the accuracy of speech-to-text algorithms and dropping storage costs, big data firms like Clarabridge are helping companies tap into customers’ raw sentiments and emotions, as expressed through recordings of call center conversations.
Clarabridge helps big companies like Wal-Mart, United Airlines, and Bank of America better understand their customers. The customer experience management (CEM) vendor runs a hosted Hadoop cluster, where it collects and analyzes data using a variety of techniques, with the goal of identifying what makes customers happy or unhappy, and providing tips to improve products and customer satisfaction.
Clarabridge has long relied on summaries of call center interactions as a source of data, along with social media data, emails, chats, forums, feedback from surveys, and data from review sites like Lithium. Each channel has its advantages and disadvantages. Twitter is notoriously “noisy” from a data quality point of view, for example, while Facebook fan pages skew positive. People who call customer service numbers tend to be angry. Getting a mix of inputs for the data analysis is crucial for accuracy’s sake.
With the introduction of Clarabridge Speech, the Reston, Virginia company is hoping to add the customer’s own voice to the mix. The company has partnered with Voci, a provider of hardware-based speech recognition technology, to enable sentiment analysis to be performed on customers’ spoken words.
The new speech-to-text analytics offering is the result of recent technological breakthroughs, Clarabridge CEO and founder Sid Banerjee says. “In the past we weren’t convinced that voice transcription technology was either accurate enough to be useful [or could scale] to support the volumes and the quantity of big data that gets produced when you deal with every single call,” Banerjee says.
Several factors contributed to the breakthrough, including improvements in the speech-to-text transcription algorithms and better price/performance of processing and storage capacity. Today, it’s not only feasible for a company to record every single incoming or outgoing call, but to train the speech-to-text algorithms on the entire data set as opposed to a sample, Banerjee says. That boosts the transcription accuracy rates and makes this sort of analysis possible.
Once Voci delivers Clarabridge the transcript, it can set lose a collection of natural language processing (NLP), sentiment scoring, and classification algorithms, using both semantic and machine approaches. “When we’re done, we have a highly indexed data set, which is numbers and scores and tags, about every part of every interaction in basically every conversation that occurs between customers and agents,” Banerjee says.
If that sounds like a lot of data, it is. In the eight years it’s been in business, Clarabridge has collected and processed hundreds of terabytes of data on behalf of its 400 or so customers. It initially developed its software on a relational database, but soon switched to Hadoop, and is now using HBase to store unstructured data.
The addition of speech analytics gives Clarabridge customers a “truer” view of their customers, Banerjee says. “It’s the actual words the customer says and it gives you a higher fidelity view,” he tells Datanami. “Just like social data, you get much more emotion and content than if somebody summarizes the conversation you have. We’re finding it’s a good way to create more color, nuance, and more completeness on the interaction channel.
Clarabridge will allow retailers, airlines, and banks to not only detect when their customers are unhappy, but also to identify what they talked about and provide an average emotion level. It can store and index this information for every minute of every call the company takes, whether it’s 10,000 calls or 100 million.
The new service will allow companies to deliver better and safer products and services, whether it’s improving the training of call center workers for a hotel chain or cleaning up cluttered retail stores. “We’ve had grocery stores make recall decisions based on complaints that products were improperly packaged or making people sick,” Banerjee says. “You get all kinds of information from things like Twitter and Facebook pages and complaints into call centers.”
For many companies, call centers provide a critical human link to customers, a connection of last resort after websites, IM chats, and mobile apps have failed to resolve an issue. These calls generate a treasure trove of data, but until now it’s been too expensive to collect and refine it. If the work that Clarabridge is doing with Voci pans out, it could trigger a new rush to include the things we say into the big data mix.
Related Items:
Deep Neural Networks Power Big Gains in Speech Recognition
A Prediction Machine Powered by Big Social Data
Fighting Telephone Fraud with Data Analytics